【图像识别】基于Matlab的迁移学习的图像分类案例
Matlab编程
Deep learning的基础知识
一、什么是迁移学习?
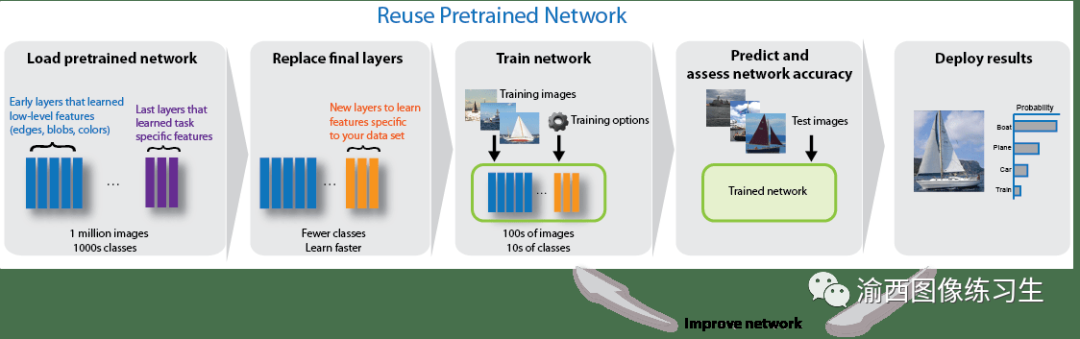
加载数据
unzip('MerchData.zip');
imds = imageDatastore('MerchData', ...
'IncludeSubfolders',true, ...
'LabelSource','foldernames');
[imdsTrain,imdsValidation] = splitEachLabel(imds,0.7,'randomized');
这个非常小的数据集现在包含 55 个训练图像和 20 个验证图像。
numTrainImages = numel(imdsTrain.Labels);
idx = randperm(numTrainImages,16);
figure
for i = 1:16
subplot(4,4,i)
I = readimage(imdsTrain,idx(i));
imshow(I)
end
加载预训练网络
net = alexnet;
使用 analyzeNetwork 可以交互可视方式呈现网络架构以及有关网络层的详细信息。
analyzeNetwork(net)
第一层(图像输入层)需要大小为 227×227×3 的输入图像
其中 3 是颜色通道数
inputSize = 1×3
227 227 3
三、网络的训练
替换最终层
layersTransfer = net.Layers(1:end-3);
numClasses = numel(categories(imdsTrain.Labels))
numClasses = 5
layers = [
layersTransfer
fullyConnectedLayer(numClasses,'WeightLearnRateFactor',20,'BiasLearnRateFactor',20)
softmaxLayer
classificationLayer];
训练网络
pixelRange = [-30 30];
imageAugmenter = imageDataAugmenter( ...
'RandXReflection',true, ...
'RandXTranslation',pixelRange, ...
'RandYTranslation',pixelRange);
augimdsTrain = augmentedImageDatastore(inputSize(1:2),imdsTrain, ...
'DataAugmentation',imageAugmenter);
对验证图像进行分类
使用经过微调的网络对验证图像进行分类
[YPred,scores] = classify(netTransfer,augimdsValidation);
显示四个示例验证图像及预测的标签。
idx = randperm(numel(imdsValidation.Files),4);
figure
for i = 1:4
subplot(2,2,i)
I = readimage(imdsValidation,idx(i));
imshow(I)
label = YPred(idx(i));
title(string(label));
end
计算针对验证集的分类准确度。准确度是网络预测正确的标签的比例
YValidation = imdsValidation.Labels;
accuracy = mean(YPred == YValidation)
accuracy = 1
今天你学废了吗???
来源:渝西图像练习生
End
声明:部分内容来源于网络,仅供读者学术交流之目的,文章版权归原作者所有。如有不妥,请联系删除。
评论