2000字详解 当Pandas遇上超大规模的数据集该如何处理呢?
Pandas
模块来对数据集进行进一步的分析与挖掘关键信息,但是当我们遇到数据集特别特别大的时候,内存就会爆掉,今天小编就来分享几个技巧,来帮助你避免遇到上述提到的这个情况。read_csv()
方法当中的chunksize
参数
read_csv()
方法当中的chunksize
参数顾名思义就是对于超大csv
文件,我们可以分块来进行读取,例如文件当中有7000万行的数据,我们将chunksize
参数设置为100万,每次分100万来分批读取,代码如下# read the large csv file with specified chunksize
df_chunk = pd.read_csv(r'data.csv', chunksize=1000000)
df_chunk
并非是一个DataFrame
对象,而是一个可迭代的对象。接下来我们使用for
循环并且将自己创立数据预处理的函数方法作用于每块的DataFrame数据集上面,代码如下chunk_list = [] # 创建一个列表chunk_list
# for循环遍历df_chunk当中的每一个DataFrame对象
for chunk in df_chunk:
# 将自己创建的数据预处理的方法作用于每个DataFrame对象上
chunk_filter = chunk_preprocessing(chunk)
# 将处理过后的结果append到上面建立的空列表当中
chunk_list.append(chunk_filter)
# 然后将列表concat到一块儿
df_concat = pd.concat(chunk_list)
将不重要的列都去除掉
# Filter out unimportant columns
df = df[['col_1','col_2', 'col_3', 'col_4', 'col_5', 'col_6','col_7', 'col_8', 'col_9', 'col_10']]
df.dropna()
方法,一般也可以提高数据的准确性以及减少内存的消耗转变数据格式
Pandas
模块会给数据列自动设置默认的数据类型,很多数据类型里面还有子类型,而这些子类型可以用更加少的字节数来表示,下表给出了各子类型所占的字节数Pandas
默认是int64
类型的某一列最大值与最小值分别是0和100,而int8
类型是可以存储数值在-128~127之间的,因此我们可以将该列从int64
类型转换成int8
类型,也就同时节省了不少内存的空间。def reduce_mem_usage(df):
""" 遍历DataFrame数据集中的每列数据集
并且更改它们的数据类型
"""
start_memory = df.memory_usage().sum() / 1024**2
print('DataFrame所占用的数据集有: {:.2f} MB'.format(start_memory))
for col in df.columns:
col_type = df[col].dtype
if col_type != object:
col_min = df[col].min()
col_max = df[col].max()
if str(col_type)[:3] == 'int':
if col_min > np.iinfo(np.int8).min and col_max < np.iinfo(np.int8).max:
df[col] = df[col].astype(np.int8)
elif col_min > np.iinfo(np.int16).min and col_max < np.iinfo(np.int16).max:
df[col] = df[col].astype(np.int16)
elif col_min > np.iinfo(np.int32).min and col_max < np.iinfo(np.int32).max:
df[col] = df[col].astype(np.int32)
elif col_min > np.iinfo(np.int64).min and col_max < np.iinfo(np.int64).max:
df[col] = df[col].astype(np.int64)
else:
if col_min > np.finfo(np.float16).min and col_max < np.finfo(np.float16).max:
df[col] = df[col].astype(np.float16)
elif col_min > np.finfo(np.float32).min and col_max < np.finfo(np.float32).max:
df[col] = df[col].astype(np.float32)
else:
df[col] = df[col].astype(np.float64)
end_memory = df.memory_usage().sum() / 1024**2
print('优化过之后数据集的内存占有: {:.2f} MB'.format(end_memory))
print('减少了大约有: {:.1f}%'.format(100 * (start_memory - end_memory) / start_memory))
return df
大家可以将小编写的这个函数方法拿去尝试一番,看一下效果如何?!
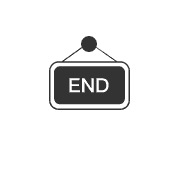
各位伙伴们好,詹帅本帅搭建了一个个人博客和小程序,汇集各种干货和资源,也方便大家阅读,感兴趣的小伙伴请移步小程序体验一下哦!(欢迎提建议)
推荐阅读
推荐阅读
评论