3000字 “婴儿级” 爬虫图文教学 | 手把手教你用Python爬取 “实习网”!
回复“书籍”即可获赠Python从入门到进阶共10本电子书
1. 为"你"而写
2. 页面分析
① 你要爬取的网站是什么?
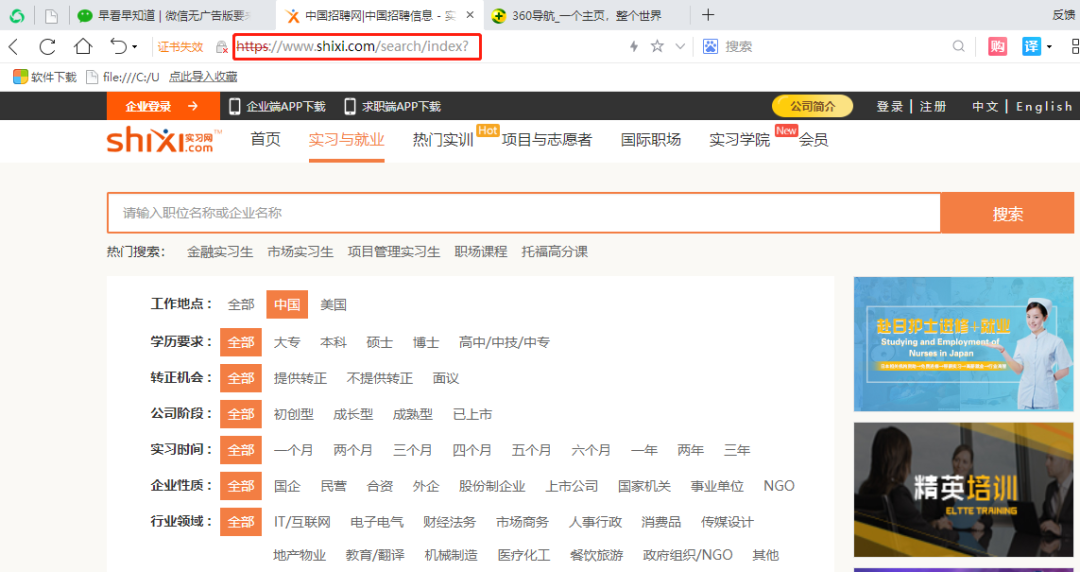
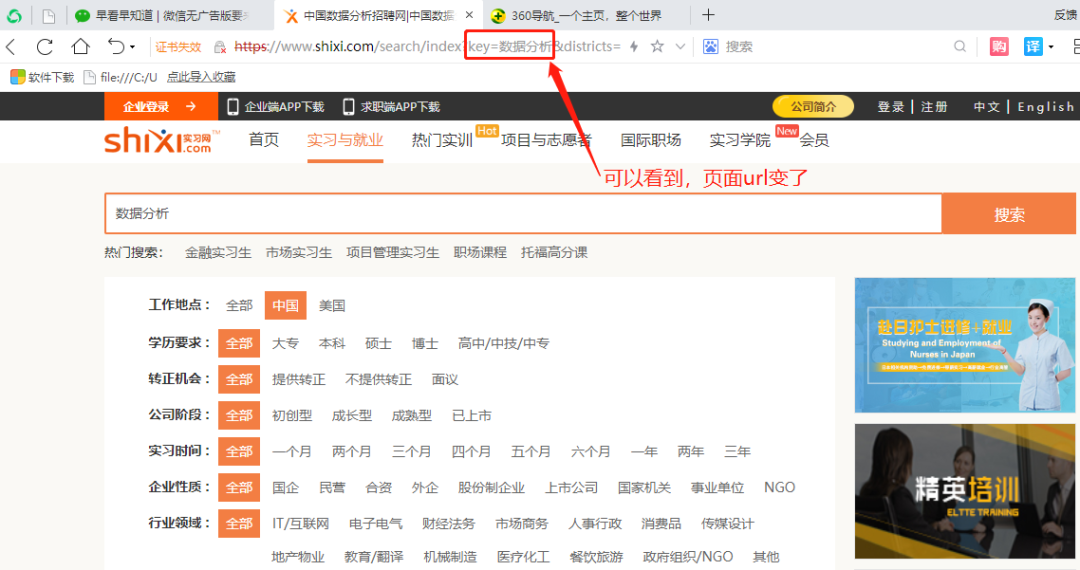
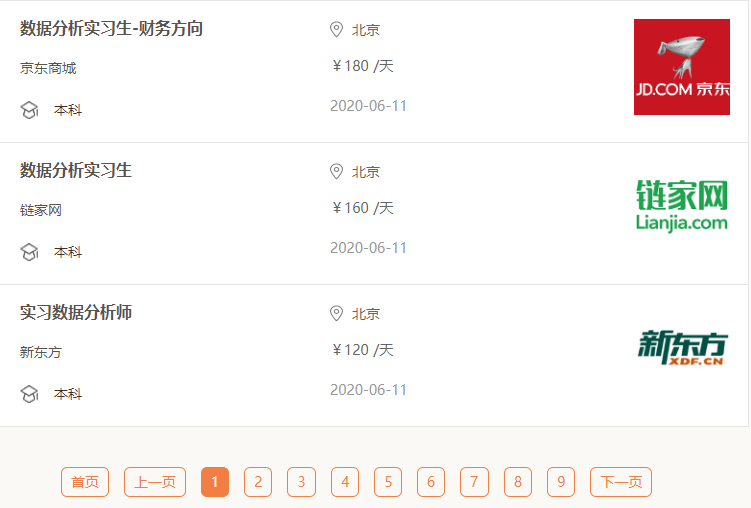
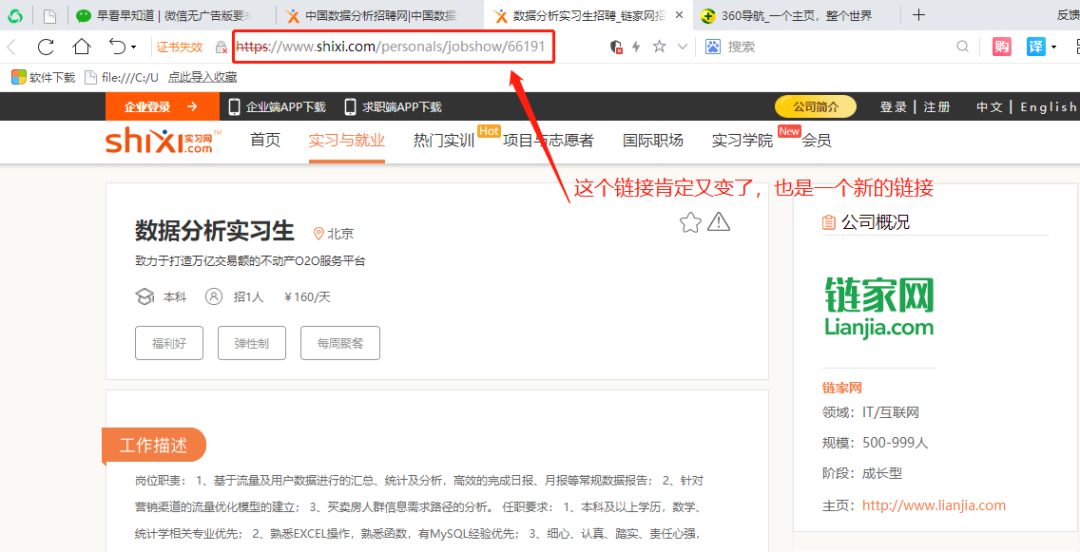
② 你要爬取页面上的哪些信息?
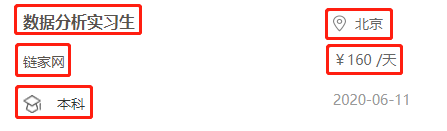
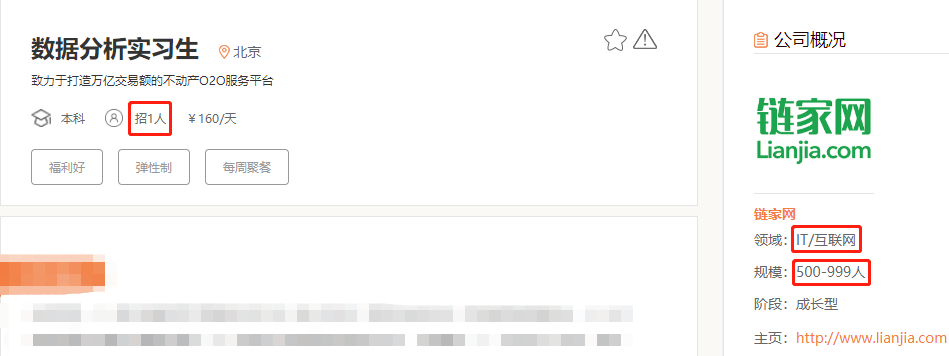
③ 页面是 “静态网页”,还是“动态网页”?
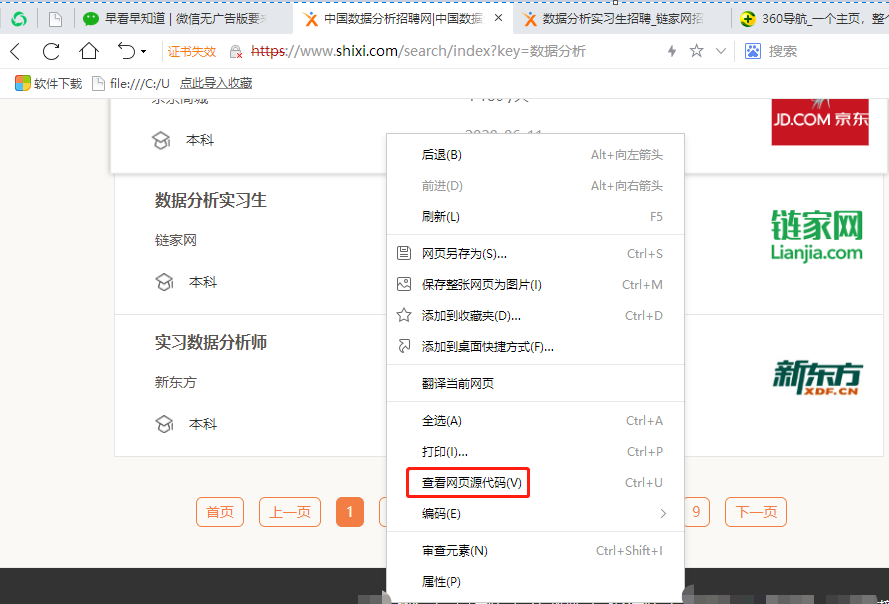
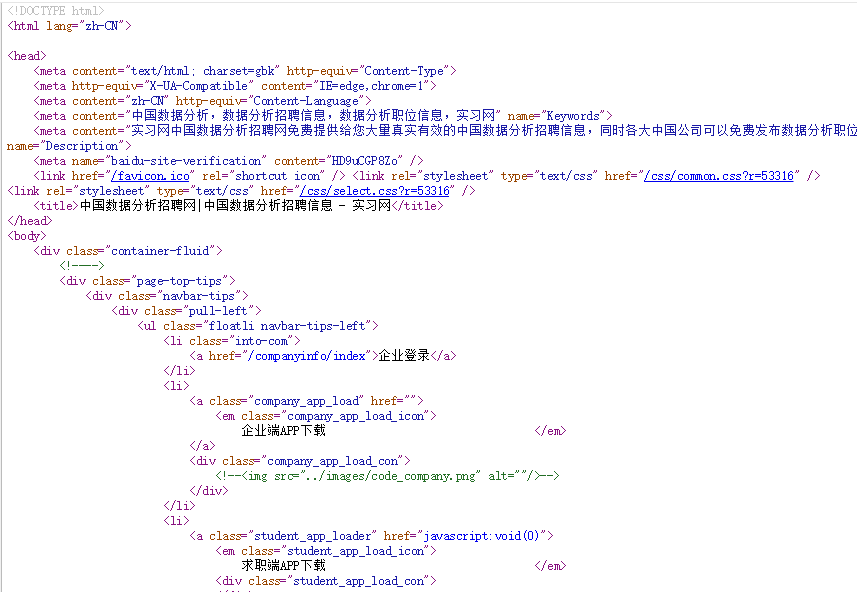
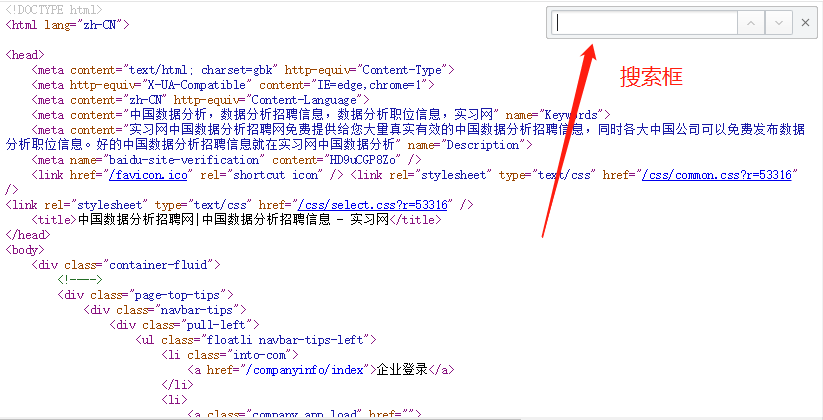
3. 如何定位数据
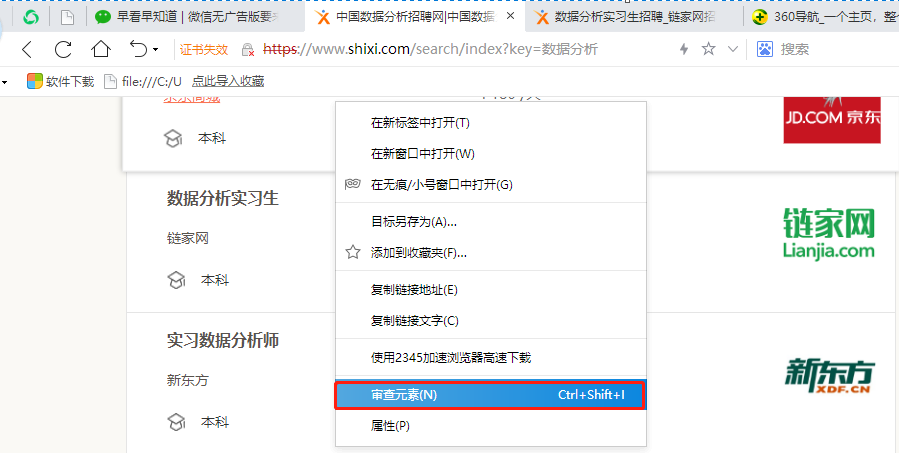
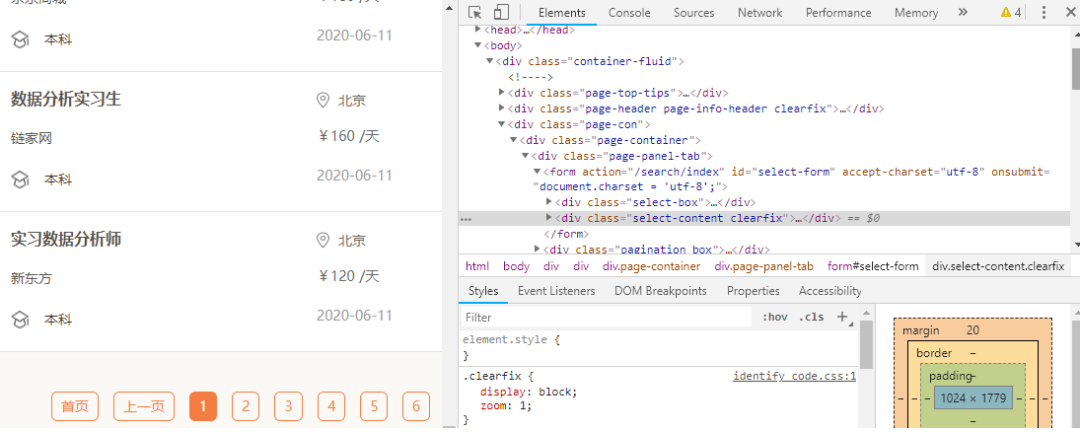
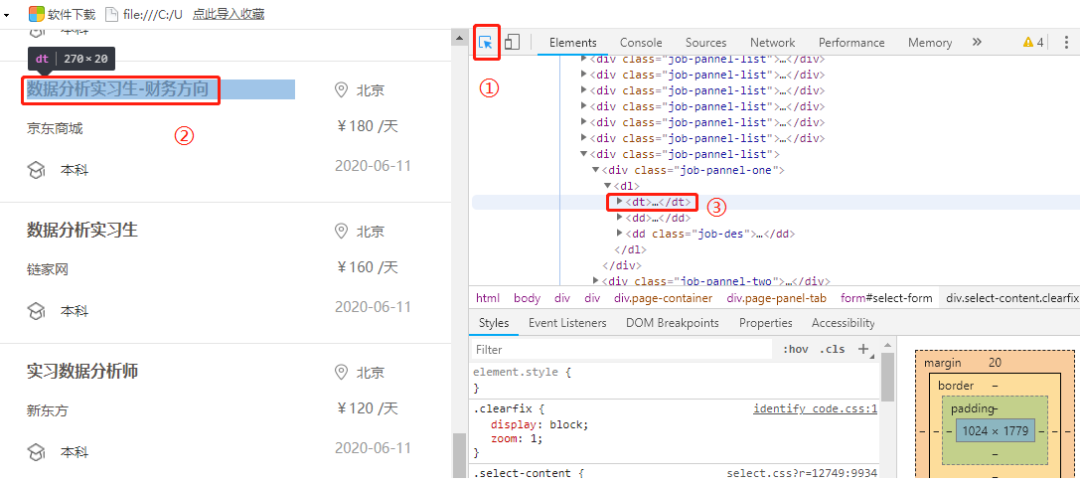
4. 爬虫代码讲解
① 导入相关库
import pandas as pd # 用于数据存储
import requests # 用于请求网页
import chardet # 用于修改编码
import re # 用于提取数据
from lxml import etree # 解析数据的库
import time # 可以粗糙模拟人为请求网页的速度
import warnings # 忽略代码运行时候的警告信息
warnings.filterwarnings("ignore")
② 请求一级页面的网页源代码
url = 'https://www.shixi.com/search/index?key=数据分析&districts=&education=0&full_opportunity=0&stage=0&practice_days=0&nature=0&trades=&lang=zh_cn'
headers = {'User-Agent': 'Mozilla/5.0 (Windows NT 6.1; Win64; x64) AppleWebKit/537.36 (KHTML, like Gecko) Chrome/71.0.3578.98 Safari/537.36'}
rqg = requests.get(url, headers=headers, verify=False) ①
rqg.encoding = chardet.detect(rqg.content)['encoding'] ②
html = etree.HTML(rqg.text)
③ 解析一级页面网页中的信息
# 1. 公司名
company_list = html.xpath('//div[@class="job-pannel-list"]//div[@class="job-pannel-one"]//a/text()')
company_list = [company_list[i].strip() for i in range(len(company_list)) if i % 2 != 0]
# 2. 岗位名
job_list = html.xpath('//div[@class="job-pannel-list"]//div[@class="job-pannel-one"]//a/text()')
job_list = [job_list[i].strip() for i in range(len(job_list)) if i % 2 == 0]
# 3. 地址
address_list = html.xpath('//div[@class="job-pannel-two"]//a/text()')
# 4. 学历
degree_list = html.xpath('//div[@class="job-pannel-list"]//dd[@class="job-des"]/span/text()')
# 5. 薪资
salary_list = html.xpath('//div[@class="job-pannel-two"]//div[@class="company-info-des"]/text()')
salary_list = [i.strip() for i in salary_list]
# 获取二级页面的链接
deep_url_list = html.xpath('//div[@class="job-pannel-list"]//dt/a/@href')
x = "https://www.shixi.com"
deep_url_list = [x + i for i in deep_url_list]
④ 解析二级页面网页中的信息
demand_list = []
area_list = []
scale_list = []
for deep_url in deep_url_list:
rqg = requests.get(deep_url, headers=headers, verify=False) ①
rqg.encoding = chardet.detect(rqg.content)['encoding'] ②
html = etree.HTML(rqg.text) ③
# 6. 需要几人
demand = html.xpath('//div[@class="container-fluid"]//div[@class="intros"]/span[2]/text()')
# 7. 公司领域
area = html.xpath('//div[@class="container-fluid"]//div[@class="detail-intro-title"]//p[1]/span/text()')
# 8. 公司规模
scale = html.xpath('//div[@class="container-fluid"]//div[@class="detail-intro-title"]//p[2]/span/text()')
demand_list.append(demand)
area_list.append(area)
scale_list.append(scale)
⑤ 翻页操作
https://www.shixi.com/search/index?key=%E6%95%B0%E6%8D%AE%E5%88%86%E6%9E%90&page=1
https://www.shixi.com/search/index?key=%E6%95%B0%E6%8D%AE%E5%88%86%E6%9E%90&page=2
https://www.shixi.com/search/index?key=%E6%95%B0%E6%8D%AE%E5%88%86%E6%9E%90&page=3
x = "https://www.shixi.com/search/index?key=数据分析&page="
url_list = [x + str(i) for i in range(1,61)]
import pandas as pd
import requests
import chardet
import re
from lxml import etree
import time
import warnings
warnings.filterwarnings("ignore")
def get_CI(url):
# ① 请求获取一级页面的源代码
url = 'https://www.shixi.com/search/index?key=数据分析&districts=&education=0&full_opportunity=0&stage=0&practice_days=0&nature=0&trades=&lang=zh_cn'
headers = {'User-Agent': 'Mozilla/5.0 (Windows NT 6.1; Win64; x64) AppleWebKit/537.36 (KHTML, like Gecko) Chrome/71.0.3578.98 Safari/537.36'}
rqg = requests.get(url, headers=headers, verify=False)
rqg.encoding = chardet.detect(rqg.content)['encoding']
html = etree.HTML(rqg.text)
# ② 获取一级页面中的信息:一共有ⅠⅡⅢⅣⅤⅥ个信息。
# Ⅰ 公司名
company_list = html.xpath('//div[@class="job-pannel-list"]//div[@class="job-pannel-one"]//a/text()')
company_list = [company_list[i].strip() for i in range(len(company_list)) if i % 2 != 0]
# Ⅱ 岗位名
job_list = html.xpath('//div[@class="job-pannel-list"]//div[@class="job-pannel-one"]//a/text()')
job_list = [job_list[i].strip() for i in range(len(job_list)) if i % 2 == 0]
# Ⅲ 地址
address_list = html.xpath('//div[@class="job-pannel-two"]//a/text()')
# Ⅳ 学历
degree_list = html.xpath('//div[@class="job-pannel-list"]//dd[@class="job-des"]/span/text()')
# Ⅴ 薪资
salary_list = html.xpath('//div[@class="job-pannel-two"]//div[@class="company-info-des"]/text()')
salary_list = [i.strip() for i in salary_list]
# Ⅵ 获取二级页面的url
deep_url_list = html.xpath('//div[@class="job-pannel-list"]//dt/a/@href')
x = "https://www.shixi.com"
deep_url_list = [x + i for i in deep_url_list]
demand_list = []
area_list = []
scale_list = []
# ③ 获取二级页面中的信息:一共有ⅠⅡⅢ三个信息。
for deep_url in deep_url_list:
rqg = requests.get(deep_url, headers=headers, verify=False)
rqg.encoding = chardet.detect(rqg.content)['encoding']
html = etree.HTML(rqg.text)
# Ⅰ 需要几人
demand = html.xpath('//div[@class="container-fluid"]//div[@class="intros"]/span[2]/text()')
# Ⅱ 公司领域
area = html.xpath('//div[@class="container-fluid"]//div[@class="detail-intro-title"]//p[1]/span/text()')
# Ⅲ 公司规模
scale = html.xpath('//div[@class="container-fluid"]//div[@class="detail-intro-title"]//p[2]/span/text()')
demand_list.append(demand)
area_list.append(area)
scale_list.append(scale)
# ④ 将每个页面获取到的所有数据,存储到DataFrame中。
data = pd.DataFrame({'公司名':company_list,'岗位名':job_list,'地址':address_list,"学历":degree_list,
'薪资':salary_list,'岗位需求量':demand_list,'公司领域':area_list,'公司规模':scale_list})
return(data)
x = "https://www.shixi.com/search/index?key=数据分析&page="
url_list = [x + str(i) for i in range(1,61)]
res = pd.DataFrame(columns=['公司名','岗位名','地址',"学历",'薪资','岗位需求量','公司领域','公司规模'])
# ⑤ 这里进行“翻页”操作
for url in url_list:
res0 = get_CI(url)
res = pd.concat([res,res0])
time.sleep(3)
# ⑥ 保存最终数据
res.to_csv('aliang.csv',encoding='utf_8_sig')
往期精彩文章推荐:
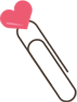
欢迎大家点赞,留言,转发,转载,感谢大家的相伴与支持
想加入Python学习群请在后台回复【入群】
万水千山总是情,点个【在看】行不行
/今日留言主题/
随便说一两句吧~
评论