如何使用PyTorch的量化功能?
共 36328字,需浏览 73分钟
· 2021-02-06
点击上方“AI算法与图像处理”,选择加"星标"或“置顶”
重磅干货,第一时间送达
来源:paperweekly
背景
更少的模型体积,接近 4 倍的减少; 可以更快的计算,由于更少的内存访问和更快的 int8 计算,可以快 2~4 倍。
Post Training Dynamic Quantization,模型训练完毕后的动态量化;
Post Training Static Quantization,模型训练完毕后的静态量化;
QAT(Quantization Aware Training),模型训练中开启量化。
Tensor的量化
>>> x = torch.rand(2,3, dtype=torch.float32)
>>> x
tensor([[0.6839, 0.4741, 0.7451],
[0.9301, 0.1742, 0.6835]])
>>> xq = torch.quantize_per_tensor(x, scale = 0.5, zero_point = 8, dtype=torch.quint8)
tensor([[0.5000, 0.5000, 0.5000],
[1.0000, 0.0000, 0.5000]], size=(2, 3), dtype=torch.quint8,
quantization_scheme=torch.per_tensor_affine, scale=0.5, zero_point=8)
>>> xq.int_repr()
tensor([[ 9, 9, 9],
[10, 8, 9]], dtype=torch.uint8)
xq = round(x / scale + zero_point)
xq = round(zero_point) = zero_point
# xq is a quantized tensor with data represented as quint8
>>> xdq = xq.dequantize()
>>> xdq
tensor([[0.5000, 0.5000, 0.5000],
[1.0000, 0.0000, 0.5000]])
xdq = (xq - zero_point) * scale
量化会有精度损失;
我们这里随便选取的 scale 和 zp 太烂,选择合适的 scale 和 zp 可以有效降低精度损失。不信你把 scale 和 zp 分别换成 scale = 0.0036, zero_point = 0试试。
Post Training Dynamic Quantization
Post:也就是训练完成后再量化模型的权重参数;
Dynamic:也就是网络在前向推理的时候动态的量化 float32 类型的输入。
torch.quantization.quantize_dynamic(model, qconfig_spec=None, dtype=torch.qint8, mapping=None, inplace=False)
Linear
LSTM
LSTMCell
RNNCell
GRUCell
qconfig_spec 指定了一组 qconfig,具体就是哪个 op 对应哪个 qconfig;
每个 qconfig 是 QConfig 类的实例,封装了两个 observer;
这两个 observer 分别是 activation 的 observer 和 weight 的 observer;
但是动态量化使用的是 QConfig 子类 QConfigDynamic 的实例,该实例实际上只封装了 weight 的 observer;
activate 就是 post process,就是 op forward 之后的后处理,但在动态量化中不包含;
observer 用来根据四元组(min_val,max_val,qmin, qmax)来计算 2 个量化的参数:scale 和 zero_point;
qmin、qmax 是算法提前确定好的,min_val 和 max_val 是从输入数据中观察到的,所以起名叫 observer。
qconfig_spec 赋值为一个 set,比如:{nn.LSTM, nn.Linear},意思是指定当前模型中的哪些 layer 要被 dynamic quantization;
qconfig_spec 赋值为一个 dict,key 为 submodule 的 name 或 type,value 为 QConfigDynamic 实例(其包含了特定的 Observer,比如 MinMaxObserver、MovingAverageMinMaxObserver、PerChannelMinMaxObserver、MovingAveragePerChannelMinMaxObserver、HistogramObserver)。
qconfig_spec = {
nn.Linear : default_dynamic_qconfig,
nn.LSTM : default_dynamic_qconfig,
nn.GRU : default_dynamic_qconfig,
nn.LSTMCell : default_dynamic_qconfig,
nn.RNNCell : default_dynamic_qconfig,
nn.GRUCell : default_dynamic_qconfig,
}
default_dynamic_qconfig = QConfigDynamic(activation=default_dynamic_quant_observer, weight=default_weight_observer)
default_dynamic_quant_observer = PlaceholderObserver.with_args(dtype=torch.float, compute_dtype=torch.quint8)
default_weight_observer = MinMaxObserver.with_args(dtype=torch.qint8, qscheme=torch.per_tensor_symmetric)
class CivilNet(nn.Module):
def __init__(self):
super(CivilNet, self).__init__()
gemfieldin = 1
gemfieldout = 1
self.conv = nn.Conv2d(gemfieldin, gemfieldout, kernel_size=1, stride=1, padding=0, groups=1, bias=False)
self.fc = nn.Linear(3, 2,bias=False)
self.relu = nn.ReLU(inplace=False)
def forward(self, x):
x = self.conv(x)
x = self.fc(x)
x = self.relu(x)
return x
#原始网络
CivilNet(
(conv): Conv2d(1, 1, kernel_size=(1, 1), stride=(1, 1), bias=False)
(fc): Linear(in_features=3, out_features=2, bias=False)
(relu): ReLU()
)
#quantize_dynamic 后
CivilNet(
(conv): Conv2d(1, 1, kernel_size=(1, 1), stride=(1, 1), bias=False)
(fc): DynamicQuantizedLinear(in_features=3, out_features=2, dtype=torch.qint8, qscheme=torch.per_tensor_affine)
(relu): ReLU()
)
# Default map for swapping dynamic modules
DEFAULT_DYNAMIC_QUANT_MODULE_MAPPINGS = {
nn.GRUCell: nnqd.GRUCell,
nn.Linear: nnqd.Linear,
nn.LSTM: nnqd.LSTM,
nn.LSTMCell: nnqd.LSTMCell,
nn.RNNCell: nnqd.RNNCell,
}
new_mod = mapping[type(mod)].from_float(mod)
使用 MinMaxObserver 计算模型中 op 权重参数中 tensor 的最大值最小值(这个例子中只有 Linear op),缩小量化时原始值的取值范围,提高量化的精度; 通过上述步骤中得到四元组中的 min_val 和 max_val,再结合算法确定的 qmin, qmax 计算出 scale 和 zp,参考前文“Tensor的量化”小节,计算得到量化后的weight,这个量化过程有torch.quantize_per_tensor 和 torch.quantize_per_channel两种,默认是前者(因为qchema默认是torch.per_tensor_affine); 实例化 nnqd.Linear,然后使用 qlinear.set_weight_bias 将量化后的 weight 和原始的 bias 设置到新的 layer 上。其中最后一步还涉及到 weight 和 bias 的打包,在源代码中是这样的:
#ifdef USE_FBGEMM
if (ctx.qEngine() == at::QEngine::FBGEMM) {
return PackedLinearWeight::prepack(std::move(weight), std::move(bias));
}
#endif
#ifdef USE_PYTORCH_QNNPACK
if (ctx.qEngine() == at::QEngine::QNNPACK) {
return PackedLinearWeightsQnnp::prepack(std::move(weight), std::move(bias));
}
#endif
TORCH_CHECK(false,"Didn't find engine for operation quantized::linear_prepack ",toString(ctx.qEngine()));
#input
torch.Tensor([[[[-1,-2,-3],[1,2,3]]]])
#经过卷积后(权重为torch.Tensor([[[[-0.7867]]]]))
torch.Tensor([[[[ 0.7867, 1.5734, 2.3601],[-0.7867, -1.5734, -2.3601]]]])
#经过fc后(权重为torch.Tensor([[ 0.4097, -0.2896, -0.4931], [-0.3738, -0.5541, 0.3243]]) )
torch.Tensor([[[[-1.2972, -0.4004], [1.2972, 0.4004]]]])
#经过relu后
torch.Tensor([[[[0.0000, 0.0000],[1.2972, 0.4004]]]])
#input
torch.Tensor([[[[-1,-2,-3],[1,2,3]]]])
#经过卷积后(权重为torch.Tensor([[[[-0.7867]]]]))
torch.Tensor([[[[ 0.7867, 1.5734, 2.3601],[-0.7867, -1.5734, -2.3601]]]])
#经过fc后(权重为torch.Tensor([[ 0.4085, -0.2912, -0.4911],[-0.3737, -0.5563, 0.3259]], dtype=torch.qint8,scale=0.0043458822183310986,zero_point=0) )
torch.Tensor([[[[-1.3038, -0.3847], [1.2856, 0.3969]]]])
#经过relu后
torch.Tensor([[[[0.0000, 0.0000], [1.2856, 0.3969]]]])
scale = max_val / (float(qmax - qmin) / 2) = 0.5541 / ((127 + 128) / 2) = 0.004345882...
zp = 0
#ifdef USE_FBGEMM
at::Tensor PackedLinearWeight::apply_dynamic_impl(at::Tensor input, bool reduce_range) {
...
fbgemm::xxxx
...
}
#endif // USE_FBGEMM
#ifdef USE_PYTORCH_QNNPACK
at::Tensor PackedLinearWeightsQnnp::apply_dynamic_impl(at::Tensor input) {
...
qnnpack::qnnpackLinearDynamic(xxxx)
...
}
#endif // USE_PYTORCH_QNNPACK
Tensor q_input = at::quantize_per_tensor(input_contig, q_params.scale, q_params.zero_point, c10::kQUInt8);
requant_scale = input_scale_fp32 * weight_scale_fp32 / output_scale_fp32
auto output_scale = 1.f
auto inverse_output_scale = 1.f /output_scale;
requant_scales[i] = (weight_scales_data[i] * input_scale) * inverse_output_scale;
#原始的模型,所有的tensor和计算都是浮点型
previous_layer_fp32 -- linear_fp32 -- activation_fp32 -- next_layer_fp32
/
linear_weight_fp32
#动态量化后的模型,Linear和LSTM的权重是int8
previous_layer_fp32 -- linear_int8_w_fp32_inp -- activation_fp32 -- next_layer_fp32
/
linear_weight_int8
Post Training Static Quantization
fuse_modules(model, modules_to_fuse, inplace=False, fuser_func=fuse_known_modules, fuse_custom_config_dict=None)
torch.quantization.fuse_modules(gemfield_model, [['conv1', 'bn1', 'relu1']], inplace=True)
class CivilNet(nn.Module):
def __init__(self):
super(CivilNet, self).__init__()
syszuxin = 1
syszuxout = 1
self.conv = nn.Conv2d(syszuxin, syszuxout, kernel_size=1, stride=1, padding=0, groups=1, bias=False)
self.fc = nn.Linear(3, 2,bias=False)
self.relu = nn.ReLU(inplace=False)
def forward(self, x):
x = self.conv(x)
x = self.fc(x)
x = self.relu(x)
return x
CivilNet(
(conv): Conv2d(1, 1, kernel_size=(1, 1), stride=(1, 1), bias=False)
(fc): Linear(in_features=3, out_features=2, bias=False)
(relu): ReLU()
)
CivilNet(
(conv): Conv2d(1, 1, kernel_size=(1, 1), stride=(1, 1), bias=False)
(fc): LinearReLU(
(0): Linear(in_features=3, out_features=2, bias=False)
(1): ReLU()
)
(relu): Identity()
)
torch.quantization.fuse_modules(a_sequential_module, ['0', '1', '2'], inplace=True)
Convolution, Batch normalization
Convolution, Batch normalization, Relu
Convolution, Relu
Linear, Relu
Batch normalization, Relu
DEFAULT_OP_LIST_TO_FUSER_METHOD : Dict[Tuple, Union[nn.Sequential, Callable]] = {
(nn.Conv1d, nn.BatchNorm1d): fuse_conv_bn,
(nn.Conv1d, nn.BatchNorm1d, nn.ReLU): fuse_conv_bn_relu,
(nn.Conv2d, nn.BatchNorm2d): fuse_conv_bn,
(nn.Conv2d, nn.BatchNorm2d, nn.ReLU): fuse_conv_bn_relu,
(nn.Conv3d, nn.BatchNorm3d): fuse_conv_bn,
(nn.Conv3d, nn.BatchNorm3d, nn.ReLU): fuse_conv_bn_relu,
(nn.Conv1d, nn.ReLU): nni.ConvReLU1d,
(nn.Conv2d, nn.ReLU): nni.ConvReLU2d,
(nn.Conv3d, nn.ReLU): nni.ConvReLU3d,
(nn.Linear, nn.ReLU): nni.LinearReLU,
(nn.BatchNorm2d, nn.ReLU): nni.BNReLU2d,
(nn.BatchNorm3d, nn.ReLU): nni.BNReLU3d,
}
#如果要部署在x86 server上
gemfield_model.qconfig = torch.quantization.get_default_qconfig('fbgemm')
#如果要部署在ARM上
gemfield_model.qconfig = torch.quantization.get_default_qconfig('qnnpack')
def get_default_qconfig(backend='fbgemm'):
if backend == 'fbgemm':
qconfig = QConfig(activation=HistogramObserver.with_args(reduce_range=True),weight=default_per_channel_weight_observer)
elif backend == 'qnnpack':
qconfig = QConfig(activation=HistogramObserver.with_args(reduce_range=False),weight=default_weight_observer)
else:
qconfig = default_qconfig
return qconfig
量化的 backend | activation | weight |
fbgemm | HistogramObserver | PerChannelMin MaxObserver (default_per_channel _weight_observer) |
qnnpack | HistogramObserver | MinMaxObserver (default_weight _observer) |
默认(非 fbgemm和qnnpack) | MinMaxObserver (default_observer) | MinMaxObserver (default_weight _observer) |
gemfield_model_prepared = torch.quantization.prepare(gemfield_model)
CivilNet(
(conv): Conv2d(
1, 1, kernel_size=(1, 1), stride=(1, 1), bias=False
(activation_post_process): HistogramObserver()
)
(fc): Linear(
in_features=3, out_features=2, bias=False
(activation_post_process): HistogramObserver()
)
(relu): ReLU(
(activation_post_process): HistogramObserver()
)
(quant): QuantStub(
(activation_post_process): HistogramObserver()
)
(dequant): DeQuantStub()
)
#至少观察个几百迭代
for data in data_loader:
gemfield_model_prepared(data)
gemfield_model_prepared_int8 = torch.quantization.convert(gemfield_model_prepared)
DEFAULT_STATIC_QUANT_MODULE_MAPPINGS = {
QuantStub: nnq.Quantize,
DeQuantStub: nnq.DeQuantize,
nn.BatchNorm2d: nnq.BatchNorm2d,
nn.BatchNorm3d: nnq.BatchNorm3d,
nn.Conv1d: nnq.Conv1d,
nn.Conv2d: nnq.Conv2d,
nn.Conv3d: nnq.Conv3d,
nn.ConvTranspose1d: nnq.ConvTranspose1d,
nn.ConvTranspose2d: nnq.ConvTranspose2d,
nn.ELU: nnq.ELU,
nn.Embedding: nnq.Embedding,
nn.EmbeddingBag: nnq.EmbeddingBag,
nn.GroupNorm: nnq.GroupNorm,
nn.Hardswish: nnq.Hardswish,
nn.InstanceNorm1d: nnq.InstanceNorm1d,
nn.InstanceNorm2d: nnq.InstanceNorm2d,
nn.InstanceNorm3d: nnq.InstanceNorm3d,
nn.LayerNorm: nnq.LayerNorm,
nn.LeakyReLU: nnq.LeakyReLU,
nn.Linear: nnq.Linear,
nn.ReLU6: nnq.ReLU6,
# Wrapper Modules:
nnq.FloatFunctional: nnq.QFunctional,
# Intrinsic modules:
nni.BNReLU2d: nniq.BNReLU2d,
nni.BNReLU3d: nniq.BNReLU3d,
nni.ConvReLU1d: nniq.ConvReLU1d,
nni.ConvReLU2d: nniq.ConvReLU2d,
nni.ConvReLU3d: nniq.ConvReLU3d,
nni.LinearReLU: nniq.LinearReLU,
nniqat.ConvBn1d: nnq.Conv1d,
nniqat.ConvBn2d: nnq.Conv2d,
nniqat.ConvBnReLU1d: nniq.ConvReLU1d,
nniqat.ConvBnReLU2d: nniq.ConvReLU2d,
nniqat.ConvReLU2d: nniq.ConvReLU2d,
nniqat.LinearReLU: nniq.LinearReLU,
# QAT modules:
nnqat.Linear: nnq.Linear,
nnqat.Conv2d: nnq.Conv2d,
}
QuantStub 的 scale 和 zp 是怎么来的(静态量化需要插入 QuantStub,后文有说明)?
conv activation 的 scale 和 zp 是怎么来的?
conv weight 的 scale 和 zp 是怎么来的?
fc activation 的 scale 和 zp 是怎么来的?
fc weight 的 scale 和 zp 是怎么来的?
relu activation 的 scale 和 zp 是怎么来的?
relu weight 的...等等,relu 没有 weight。
if self.dtype == torch.qint8:
if self.reduce_range:
qmin, qmax = -64, 63
else:
qmin, qmax = -128, 127
else:
if self.reduce_range:
qmin, qmax = 0, 127
else:
qmin, qmax = 0, 255
#qscheme 是 torch.per_tensor_symmetric 或者torch.per_channel_symmetric时
max_val = torch.max(-min_val, max_val)
scale = max_val / (float(qmax - qmin) / 2)
scale = torch.max(scale, torch.tensor(self.eps, device=device, dtype=scale.dtype))
if self.dtype == torch.quint8:
zero_point = zero_point.new_full(zero_point.size(), 128)
#qscheme 是 torch.per_tensor_affine时
scale = (max_val - min_val) / float(qmax - qmin)
scale = torch.max(scale, torch.tensor(self.eps, device=device, dtype=scale.dtype))
zero_point = qmin - torch.round(min_val / scale)
zero_point = torch.max(zero_point, torch.tensor(qmin, device=device, dtype=zero_point.dtype))
zero_point = torch.min(zero_point, torch.tensor(qmax, device=device, dtype=zero_point.dtype))
scale = 0.7898 / ((127 + 128)/2 ) = 0.0062
zp = 0
scale = (2.9971 + 3) / (127 - 0) = 0.0472
zp = 0 - round(-3 /0.0472) = 64
#原始的CivilNet网络:
CivilNet(
(conv): Conv2d(1, 1, kernel_size=(1, 1), stride=(1, 1), bias=False)
(fc): Linear(in_features=3, out_features=2, bias=False)
(relu): ReLU()
)
#静态量化后的CivilNet网络:
CivilNet(
(conv): QuantizedConv2d(1, 1, kernel_size=(1, 1), stride=(1, 1), scale=0.0077941399067640305, zero_point=0, bias=False)
(fc): QuantizedLinear(in_features=3, out_features=2, scale=0.002811126410961151, zero_point=14, qscheme=torch.per_channel_affine)
(relu): QuantizedReLU()
)
import torch
import torch.nn as nn
class CivilNet(nn.Module):
def __init__(self):
super(CivilNet, self).__init__()
in_planes = 1
out_planes = 1
self.conv = nn.Conv2d(in_planes, out_planes, kernel_size=1, stride=1, padding=0, groups=1, bias=False)
self.fc = nn.Linear(3, 2,bias=False)
self.relu = nn.ReLU(inplace=False)
self.quant = QuantStub()
self.dequant = DeQuantStub()
def forward(self, x):
x = self.quant(x)
x = self.conv(x)
x = self.fc(x)
x = self.relu(x)
x = self.dequant(x)
return x
def forward(self, X):
return torch.quantize_per_tensor(X, float(self.scale), int(self.zero_point), self.dtype)
def forward(self, Xq):
return Xq.dequantize()
c = CivilNet()
t = torch.Tensor([[[[-1,-2,-3],[1,2,3]]]])
c(t)
#input
torch.Tensor([[[[-1,-2,-3],[1,2,3]]]])
#经过卷积后(权重为torch.Tensor([[[[-0.7867]]]]))
torch.Tensor([[[[ 0.7867, 1.5734, 2.3601],[-0.7867, -1.5734, -2.3601]]]])
#经过fc后(权重为torch.Tensor([[ 0.4097, -0.2896, -0.4931], [-0.3738, -0.5541, 0.3243]]) )
torch.Tensor([[[[-1.2972, -0.4004], [1.2972, 0.4004]]]])
#经过relu后
torch.Tensor([[[[0.0000, 0.0000],[1.2972, 0.4004]]]])
#input
torch.Tensor([[[[-1,-2,-3],[1,2,3]]]])
#QuantStub后 (scale=tensor([0.0472]), zero_point=tensor([64]))
tensor([[[[-0.9916, -1.9833, -3.0221],[ 0.9916, 1.9833, 3.0221]]]],
dtype=torch.quint8, scale=0.04722102731466293, zero_point=64)
#经过卷积后(权重为torch.Tensor([[[[-0.7898]]]], dtype=torch.qint8, scale=0.0062, zero_point=0))
#conv activation(输入)的scale为0.03714831545948982,zp为64
torch.Tensor([[[[ 0.7801, 1.5602, 2.3775],[-0.7801, -1.5602, -2.3775]]]], scale=0.03714831545948982, zero_point=64)
#经过fc后(权重为torch.Tensor([[ 0.4100, -0.2901, -0.4951],[-0.3737, -0.5562, 0.3259]], dtype=torch.qint8, scale=tensor([0.0039, 0.0043]),zero_point=tensor([0, 0])) )
#fc activation(输入)的scale为0.020418135449290276, zp为64
torch.Tensor([[[[-1.3068, -0.3879],[ 1.3068, 0.3879]]]], dtype=torch.quint8, scale=0.020418135449290276, zero_point=64)
#经过relu后
torch.Tensor([[[[0.0000, 0.0000],[1.3068, 0.3879]]]], dtype=torch.quint8, scale=0.020418135449290276, zero_point=64)
#经过DeQuantStub后
torch.Tensor([[[[0.0000, 0.0000],[1.3068, 0.3879]]]])
import torch
import torch.nn.quantized as nnq
#输入
>>> x = torch.Tensor([[[[-1,-2,-3],[1,2,3]]]])
>>> x
tensor([[[[-1., -2., -3.],
[ 1., 2., 3.]]]])
#经过QuantStub
>>> xq = torch.quantize_per_tensor(x, scale = 0.0472, zero_point = 64, dtype=torch.quint8)
>>> xq
tensor([[[[-0.9912, -1.9824, -3.0208],
[ 0.9912, 1.9824, 3.0208]]]], size=(1, 1, 2, 3),
dtype=torch.quint8, quantization_scheme=torch.per_tensor_affine,
scale=0.0472, zero_point=64)
>>> xq.int_repr()
tensor([[[[ 43, 22, 0],
[ 85, 106, 128]]]], dtype=torch.uint8)
>>> c = nnq.Conv2d(1,1,1)
>>> weight = torch.Tensor([[[[-0.7898]]]])
>>> qweight = torch.quantize_per_channel(weight, scales=torch.Tensor([0.0062]).to(torch.double), zero_points = torch.Tensor([0]).to(torch.int64), axis=0, dtype=torch.qint8)
>>> c.set_weight_bias(qweight, None)
>>> c.scale = 0.03714831545948982
>>> c.zero_point = 64
>>> x = c(xq)
>>> x
tensor([[[[ 0.7801, 1.5602, 2.3775],
[-0.7801, -1.5602, -2.3775]]]], size=(1, 1, 2, 3),
dtype=torch.quint8, quantization_scheme=torch.per_tensor_affine,
scale=0.03714831545948982, zero_point=64)
def forward(self, input):
return ops.quantized.conv2d(input, self._packed_params, self.scale, self.zero_point)
def forward(self, x):
return torch.ops.quantized.linear(x, self._packed_params._packed_params, self.scale, self.zero_point)
#原始的模型,所有的tensor和计算都是浮点型
previous_layer_fp32 -- linear_fp32 -- activation_fp32 -- next_layer_fp32
/
linear_weight_fp32
#静态量化的模型,权重和输入都是int8
previous_layer_int8 -- linear_with_activation_int8 -- next_layer_int8
/
linear_weight_int8
静态量化的 float 输入必经 QuantStub 变为 int,此后到输出之前都是 int;
动态量化的 float 输入是经动态计算的 scale 和 zp 量化为 int,op 输出时转换回 float。
QAT(Quantization Aware Training)
cnet = CivilNet()
cnet.train()
cnet.qconfig = torch.quantization.get_default_qat_qconfig('fbgemm')
#activation的observer的参数
FakeQuantize.with_args(observer=MovingAverageMinMaxObserver,quant_min=0,quant_max=255,reduce_range=True)
#权重的observer的参数
FakeQuantize.with_args(observer=MovingAveragePerChannelMinMaxObserver,
quant_min=-128,
quant_max=127,
dtype=torch.qint8,
qscheme=torch.per_channel_symmetric,
reduce_range=False,
ch_axis=0)
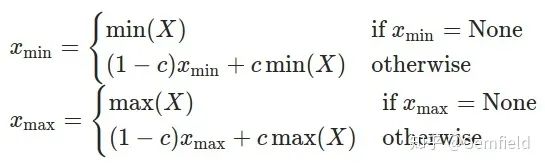
Xmin、Xmax 是当前运行中正在求解和最终求解的最小值、最大值;
X 是当前输入的 tensor;
c 是一个常数,PyTorch 中默认为 0.01,也就是最新一次的极值由上一次贡献 99%,当前的 tensor 贡献 1%。
prepare_qa t 要把 qconfig 安插到每个 op 上,qconfig 的内容本身就不同,参考五部曲中的第一步; prepare_qat 中需要多做一步转换子 module 的工作,需要 inplace 的把模型中的一些子 module 替换了,替换的逻辑就是从 DEFAULT_QAT_MODULE_MAPPINGS 的 key 替换为 value,这个字典的定义如下:
# Default map for swapping float module to qat modules
DEFAULT_QAT_MODULE_MAPPINGS : Dict[Callable, Any] = {
nn.Conv2d: nnqat.Conv2d,
nn.Linear: nnqat.Linear,
# Intrinsic modules:
nni.ConvBn1d: nniqat.ConvBn1d,
nni.ConvBn2d: nniqat.ConvBn2d,
nni.ConvBnReLU1d: nniqat.ConvBnReLU1d,
nni.ConvBnReLU2d: nniqat.ConvBnReLU2d,
nni.ConvReLU2d: nniqat.ConvReLU2d,
nni.LinearReLU: nniqat.LinearReLU
}
CivilNet(
(conv): QATConv2d(
1, 1, kernel_size=(1, 1), stride=(1, 1), bias=False
(activation_post_process): FakeQuantize(
fake_quant_enabled=tensor([1], dtype=torch.uint8), observer_enabled=tensor([1], dtype=torch.uint8), scale=tensor([1.]), zero_point=tensor([0])
(activation_post_process): MovingAverageMinMaxObserver(min_val=tensor([]), max_val=tensor([]))
)
(weight_fake_quant): FakeQuantize(
fake_quant_enabled=tensor([1], dtype=torch.uint8), observer_enabled=tensor([1], dtype=torch.uint8), scale=tensor([1.]), zero_point=tensor([0])
(activation_post_process): MovingAveragePerChannelMinMaxObserver(min_val=tensor([]), max_val=tensor([]))
)
)
(fc): QATLinear(
in_features=3, out_features=2, bias=False
(activation_post_process): FakeQuantize(
fake_quant_enabled=tensor([1], dtype=torch.uint8), observer_enabled=tensor([1], dtype=torch.uint8), scale=tensor([1.]), zero_point=tensor([0])
(activation_post_process): MovingAverageMinMaxObserver(min_val=tensor([]), max_val=tensor([]))
)
(weight_fake_quant): FakeQuantize(
fake_quant_enabled=tensor([1], dtype=torch.uint8), observer_enabled=tensor([1], dtype=torch.uint8), scale=tensor([1.]), zero_point=tensor([0])
(activation_post_process): MovingAveragePerChannelMinMaxObserver(min_val=tensor([]), max_val=tensor([]))
)
)
(relu): ReLU(
(activation_post_process): FakeQuantize(
fake_quant_enabled=tensor([1], dtype=torch.uint8), observer_enabled=tensor([1], dtype=torch.uint8), scale=tensor([1.]), zero_point=tensor([0])
(activation_post_process): MovingAverageMinMaxObserver(min_val=tensor([]), max_val=tensor([]))
)
)
(quant): QuantStub(
(activation_post_process): FakeQuantize(
fake_quant_enabled=tensor([1], dtype=torch.uint8), observer_enabled=tensor([1], dtype=torch.uint8), scale=tensor([1.]), zero_point=tensor([0])
(activation_post_process): MovingAverageMinMaxObserver(min_val=tensor([]), max_val=tensor([]))
)
)
(dequant): DeQuantStub()
)
def forward(self, input):
return self.activation_post_process(self._conv_forward(input, self.weight_fake_quant(self.weight)))
def forward(self, input):
return self.activation_post_process(F.linear(input, self.weight_fake_quant(self.weight), self.bias))
#conv2d
weight=functools.partial(<class 'torch.quantization.fake_quantize.FakeQuantize'>,
observer=<class 'torch.quantization.observer.MovingAveragePerChannelMinMaxObserver'>,
quant_min=-128, quant_max=127, dtype=torch.qint8,
qscheme=torch.per_channel_symmetric, reduce_range=False, ch_axis=0))
activation=functools.partial(<class 'torch.quantization.fake_quantize.FakeQuantize'>,
observer=<class 'torch.quantization.observer.MovingAverageMinMaxObserver'>,
quant_min=0, quant_max=255, reduce_range=True)
def forward(self, X):
if self.observer_enabled[0] == 1:
#使用移动平均算法计算scale和zp
if self.fake_quant_enabled[0] == 1:
X = torch.fake_quantize_per_channel_or_tensor_affine(X...)
return X
DEFAULT_STATIC_QUANT_MODULE_MAPPINGS = {
......
# QAT modules:
nnqat.Linear: nnq.Linear,
nnqat.Conv2d: nnq.Conv2d,
}
# 原始的模型,所有的tensor和计算都是浮点
previous_layer_fp32 -- linear_fp32 -- activation_fp32 -- next_layer_fp32
/
linear_weight_fp32
# 训练过程中,fake_quants发挥作用
previous_layer_fp32 -- fq -- linear_fp32 -- activation_fp32 -- fq -- next_layer_fp32
/
linear_weight_fp32 -- fq
# 量化后的模型进行推理,权重和输入都是int8
previous_layer_int8 -- linear_with_activation_int8 -- next_layer_int8
/
linear_weight_int8
总结
https://github.com/DeepVAC/deepvac
参考文献
个人微信(如果没有备注不拉群!) 请注明:地区+学校/企业+研究方向+昵称
下载1:何恺明顶会分享
在「AI算法与图像处理」公众号后台回复:何恺明,即可下载。总共有6份PDF,涉及 ResNet、Mask RCNN等经典工作的总结分析
下载2:终身受益的编程指南:Google编程风格指南
在「AI算法与图像处理」公众号后台回复:c++,即可下载。历经十年考验,最权威的编程规范!
下载3 CVPR2020 在「AI算法与图像处理」公众号后台回复:CVPR2020,即可下载1467篇CVPR 2020论文
觉得不错就点亮在看吧