ViT:视觉Transformer backbone网络ViT论文与代码详解
机器学习实验室
共 9157字,需浏览 19分钟
· 2021-06-08
Visual Transformer
Author:louwill
Machine Learning Lab
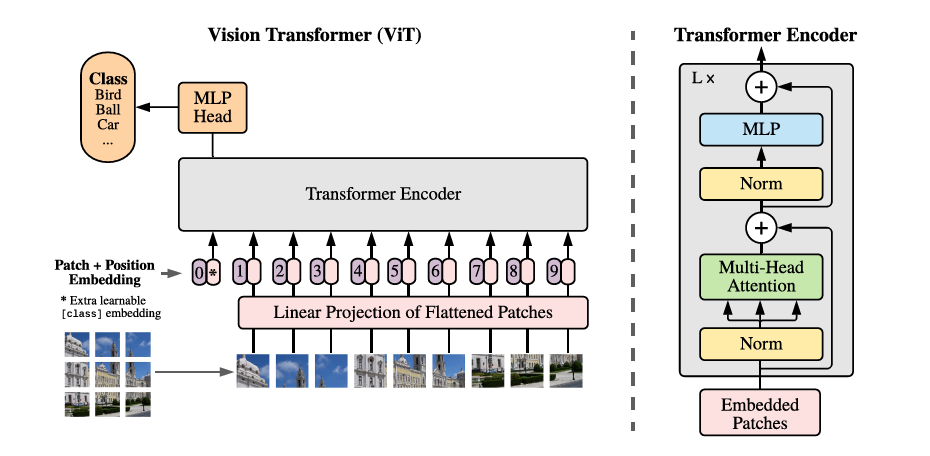


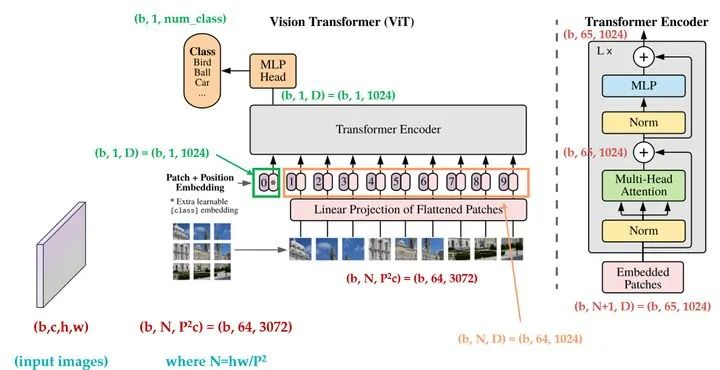
ILSVRC-2012 ImageNet dataset:1000 classes ImageNet-21k:21k classes JFT:18k High Resolution Images
CIFAR-10/100 Oxford-IIIT Pets Oxford Flowers-102 VTAB
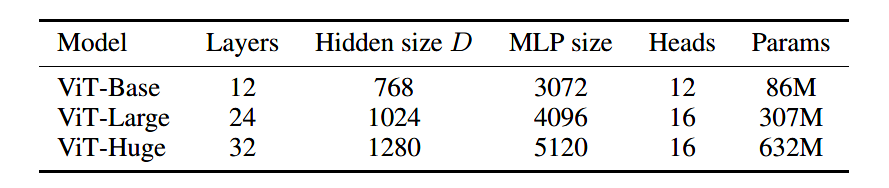
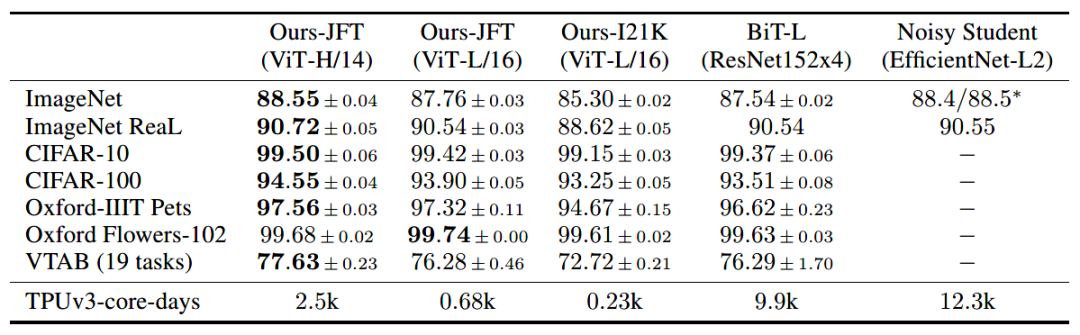
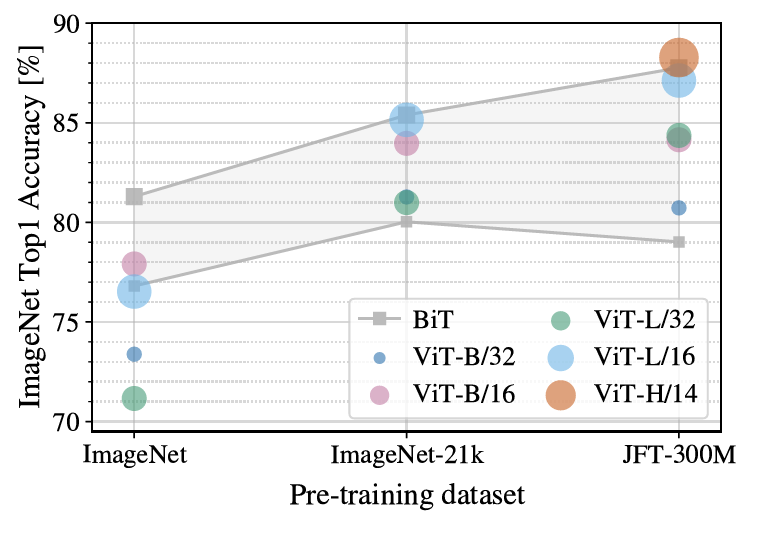
pip install vit-pytorch
import torch
from vit_pytorch import ViT
# 创建ViT模型实例
v = ViT(
image_size = 256,
patch_size = 32,
num_classes = 1000,
dim = 1024,
depth = 6,
heads = 16,
mlp_dim = 2048,
dropout = 0.1,
emb_dropout = 0.1
)
# 随机化一个图像输入
img = torch.randn(1, 3, 256, 256)
# 获取输出
preds = v(img) # (1, 1000)
image_size:原始图像尺寸
patch_size:图像块的尺寸
num_classes:类别数量
dim:Transformer隐变量维度大小
depth:Transformer编码器层数
Heads:MSA中的head数
dropout:失活比例
emb_dropout:嵌入层失活比例
# 导入相关模块
import torch
from torch import nn, einsum
import torch.nn.functional as F
from einops import rearrange, repeat
from einops.layers.torch import Rearrange
# 辅助函数,生成元组
def pair(t):
return t if isinstance(t, tuple) else (t, t)
# 规范化层的类封装
class PreNorm(nn.Module):
def __init__(self, dim, fn):
super().__init__()
self.norm = nn.LayerNorm(dim)
self.fn = fn
def forward(self, x, **kwargs):
return self.fn(self.norm(x), **kwargs)
# FFN
class FeedForward(nn.Module):
def __init__(self, dim, hidden_dim, dropout = 0.):
super().__init__()
self.net = nn.Sequential(
nn.Linear(dim, hidden_dim),
nn.GELU(),
nn.Dropout(dropout),
nn.Linear(hidden_dim, dim),
nn.Dropout(dropout)
)
def forward(self, x):
return self.net(x)
# Attention
class Attention(nn.Module):
def __init__(self, dim, heads = 8, dim_head = 64, dropout = 0.):
super().__init__()
inner_dim = dim_head * heads
project_out = not (heads == 1 and dim_head == dim)
self.heads = heads
self.scale = dim_head ** -0.5
self.attend = nn.Softmax(dim = -1)
self.to_qkv = nn.Linear(dim, inner_dim * 3, bias = False)
self.to_out = nn.Sequential(
nn.Linear(inner_dim, dim),
nn.Dropout(dropout)
) if project_out else nn.Identity()
def forward(self, x):
b, n, _, h = *x.shape, self.heads
qkv = self.to_qkv(x).chunk(3, dim = -1)
q, k, v = map(lambda t: rearrange(t, 'b n (h d) -> b h n d', h = h), qkv)
dots = einsum('b h i d, b h j d -> b h i j', q, k) * self.scale
attn = self.attend(dots)
out = einsum('b h i j, b h j d -> b h i d', attn, v)
out = rearrange(out, 'b h n d -> b n (h d)')
return self.to_out(out)
# 基于PreNorm、Attention和FFN搭建Transformer
class Transformer(nn.Module):
def __init__(self, dim, depth, heads, dim_head, mlp_dim, dropout = 0.):
super().__init__()
self.layers = nn.ModuleList([])
for _ in range(depth):
self.layers.append(nn.ModuleList([
PreNorm(dim, Attention(dim, heads = heads, dim_head = dim_head, dropout = dropout)),
PreNorm(dim, FeedForward(dim, mlp_dim, dropout = dropout))
]))
def forward(self, x):
for attn, ff in self.layers:
x = attn(x) + x
x = ff(x) + x
return x
(3) 搭建ViT
class ViT(nn.Module):
def __init__(self, *, image_size, patch_size, num_classes, dim, depth, heads, mlp_dim, pool = 'cls', channels = 3, dim_head = 64, dropout = 0., emb_dropout = 0.):
super().__init__()
image_height, image_width = pair(image_size)
patch_height, patch_width = pair(patch_size)
assert image_height % patch_height == 0 and image_width % patch_width == 0, 'Image dimensions must be divisible by the patch size.'
# patch数量
num_patches = (image_height // patch_height) * (image_width // patch_width)
# patch维度
patch_dim = channels * patch_height * patch_width
assert pool in {'cls', 'mean'}, 'pool type must be either cls (cls token) or mean (mean pooling)'
# 定义块嵌入
self.to_patch_embedding = nn.Sequential(
Rearrange('b c (h p1) (w p2) -> b (h w) (p1 p2 c)', p1 = patch_height, p2 = patch_width),
nn.Linear(patch_dim, dim),
)
# 定义位置编码
self.pos_embedding = nn.Parameter(torch.randn(1, num_patches + 1, dim))
# 定义类别向量
self.cls_token = nn.Parameter(torch.randn(1, 1, dim))
self.dropout = nn.Dropout(emb_dropout)
self.transformer = Transformer(dim, depth, heads, dim_head, mlp_dim, dropout)
self.pool = pool
self.to_latent = nn.Identity()
# 定义MLP
self.mlp_head = nn.Sequential(
nn.LayerNorm(dim),
nn.Linear(dim, num_classes)
)
# ViT前向流程
def forward(self, img):
# 块嵌入
x = self.to_patch_embedding(img)
b, n, _ = x.shape
# 追加类别向量
cls_tokens = repeat(self.cls_token, '() n d -> b n d', b = b)
x = torch.cat((cls_tokens, x), dim=1)
# 追加位置编码
x += self.pos_embedding[:, :(n + 1)]
# dropout
x = self.dropout(x)
# 输入到transformer
x = self.transformer(x)
x = x.mean(dim = 1) if self.pool == 'mean' else x[:, 0]
x = self.to_latent(x)
# MLP
return self.mlp_head(x)
小结
参考资料:
An Image Is Worth 16X16 Words: Transformers for Image Recognition at Scale
https://github.com/lucidrains/vit-pytorch
https://mp.weixin.qq.com/s/ozUHHGMqIC0-FRWoNGhVYQ
往期精彩:
求个在看
评论
英伟达Blackwell平台网络配置分析
本文来自“英伟达Blachwell平台网络配置详解”。GTC大会英伟达展示了全新的 Blackwell 平台系列产品,包括 HGX B100 服务器、NVLINK Switch、GB200Superchip Computer Node、Quantum X800 交换机和 CX8 网卡(InfiniB
架构师技术联盟
0
一女子与一男子在阳台上打扑克,被邻居偷拍后...
近日网络上又发生了一起疑似黄色谣言的事件:一女子与一男子在阳台上打扑克,被邻居偷拍后上传到网上,引发广泛舆论讨论。根据网传视频显示,一名穿着吊带睡衣的女子与一名光着上身的男性在阳台上交谈,随后开始打起扑克牌。这一幕被邻居拍下并上传至网络后,引发了许多网友的关注和猜测,其中大部分涉及到了不当的假设。当
逆锋起笔
0
CVPR 2024|大视觉模型的开山之作!无需任何语言数据即可打造大视觉模型
↑ 点击蓝字 关注极市平台作者丨科技猛兽编辑丨极市平台极市导读 本文提出一种序列建模 (sequential modeling) 的方法,不使用任何语言数据,训练大视觉模型。>>加入极市CV技术交流群,走在计算机视觉的最前沿本文目录1 序列建模打造大视觉模型(来自 U
极市平台
1
字节的跳动职级与薪资(2024年)
上一篇:阿里公布年终奖,P7, 3.5+,22W年终奖,还有35W长期现金激励,真香字节跳动自2012年3月成立以来,已经迅速成长为一个全球性的科技公司。其产品和服务已经遍布全球150多个国家与地区,并且支持超过75种不同的语言。在字节跳动的官方网站上,列出了一系列引人注目的产品和服务,包括但不限于
开发者全社区
0
阿里的同事,写的代码真 TMD 优雅!
通过这篇文章你将了解到整洁的代码对项目、公司和你的重要性,以及如何书写整洁的代码.通过命名、类、函数、测试这四个章节,使我们的代码变得整洁.1、为什么要保持代码整洁?不整洁的代码随着时间的增加而增加时,生产力会随之降低.导致的结果就是:代码不易扩展或扩展容易引发其他问题程序崩溃加班增加公司成本(加人
Java专栏
1
老爸嘲讽我了,写破代码一年就挣十几万,他在工地带50个工人,一个月光人头费就3万,让我滚回去跟他干!
点击上方 "大数据肌肉猿"关注, 星标一起成长点击下方链接,进入高质量学习交流群今日更新| 1052个转型案例分享-大数据交流群来自:网络,侵删有个网友的父亲是做工程的,天天就嘲笑他,说他天天写着破代码有啥用,一年就拿个十多万的死工资,然后告诉他自己在工地里面带了50个工人,一个月能抽三万
程序源代码
0
光纤详解:光纤跳线如何分类,多向单模转换?
本文来自“光纤详解:光纤跳线如何分类,多向单模转换?”,光纤跳线作为光网络布线最基础的元件之一,被广泛应用于光纤链路的搭建中。如今,光纤制造商根据应用场景的不同推出众多类型的光纤跳线,如MPO/LC/SC/FC/ST光纤跳线,单工/双工光纤跳线,单模/多模光纤跳线等,它们之间各有特色,且不可替代。本
架构师技术联盟
0
管理世界2024 | 使用管理层讨论与分析测量「企业人工智能指标」
Tips: 公众号推送后内容只能更改一次,且只能改20字符。如果内容出问题,或者想更新内容, 只能重复推送。为了更好的阅读体验,建议阅读本文博客版, 链接地址 https://textdata.cn/blog/2024-04-19-ai-improve-firm-productivity/
大邓和他的Python
0