使用Python和OCR进行文档解析的完整代码演示(附代码)

来源:DeepHub IMBA 本文约2300字,建议阅读5分钟 本文中将使用Python演示如何解析文档(如pdf)并提取文本,图形,表格等信息。
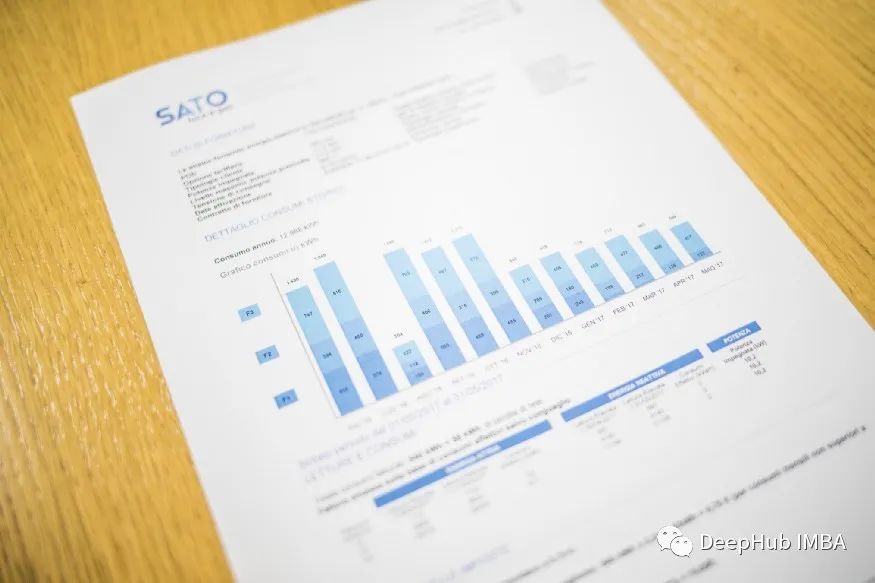
https://s2.q4cdn.com/470004039/files/doc_financials/2021/q4/_10-K-2021-(As-Filed).pdf
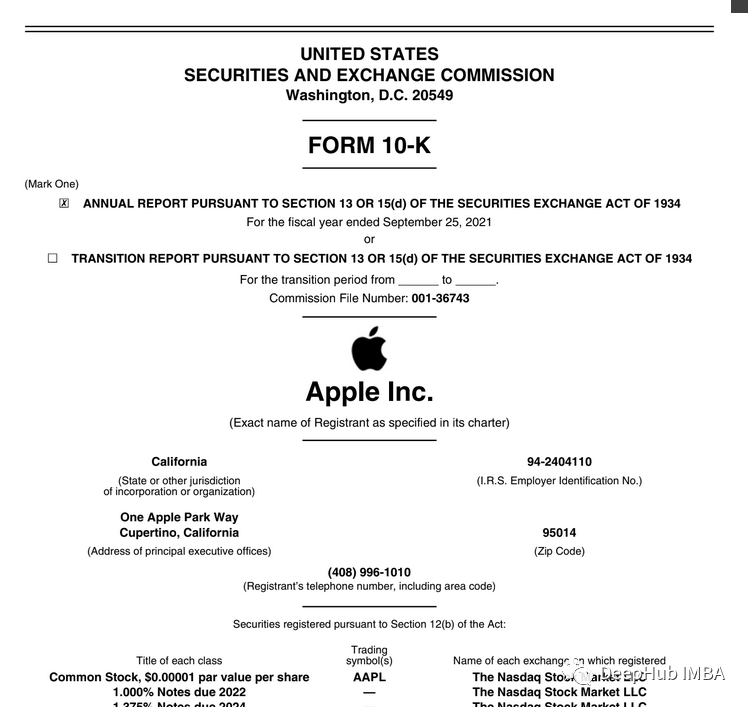
环境设置
以文本方式处理文档:用PyPDF2提取文本,用Camelot或TabulaPy提取表,用PyMuPDF提取图形。 将文档转换为图像(OCR):使用pdf2image进行转换,使用PyTesseract以及许多其他的库提取数据,或者只使用LayoutParser。
# with pip
pip install python-poppler
# with conda
conda install -c conda-forge poppler
# READ AS IMAGE
import pdf2imagedoc = pdf2image.convert_from_path("doc_apple.pdf")
len(doc) #<-- check num pages
doc[0] #<-- visualize a page
# Save imgs
import osfolder = "doc"
if folder not in os.listdir():
os.makedirs(folder)p = 1
for page in doc:
image_name = "page_"+str(p)+".jpg"
page.save(os.path.join(folder, image_name), "JPEG")
p = p+1
pip install layoutparser torchvision && pip install "git+https://github.com/facebookresearch/detectron2.git@v0.5#egg=detectron2"
pip install "layoutparser[ocr]"
import layoutparser as lp
import cv2
import numpy as np
import io
import pandas as pd
import matplotlib.pyplot as plt
检测
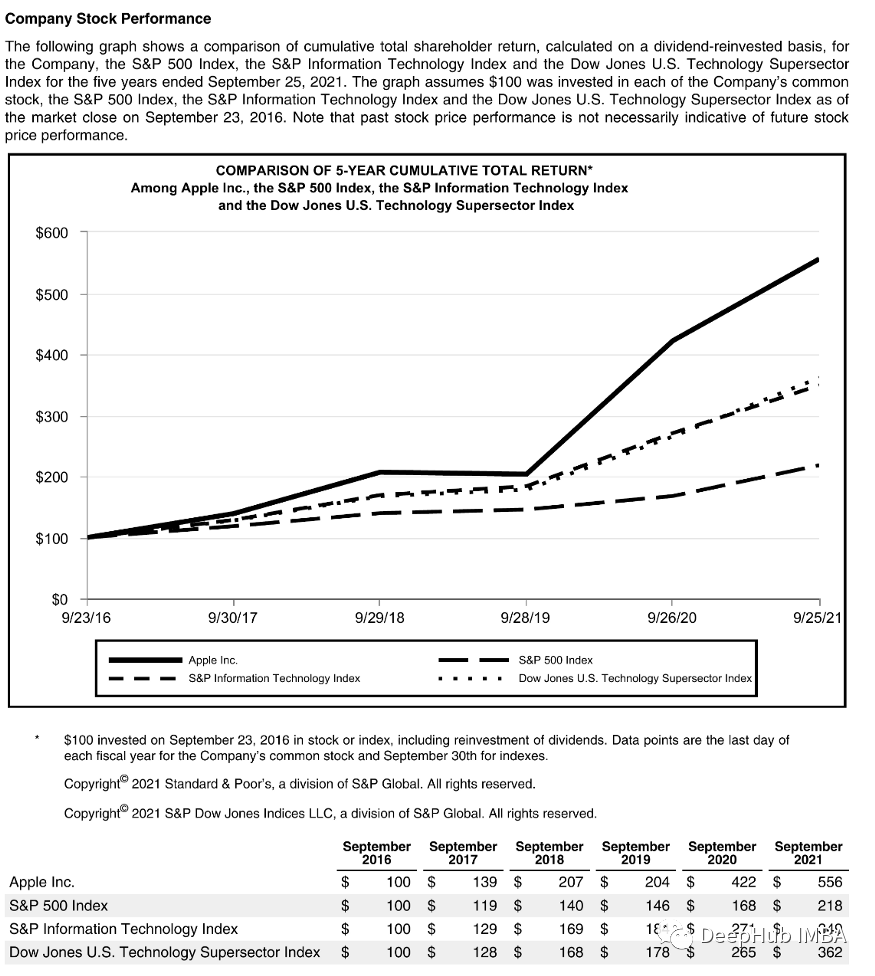
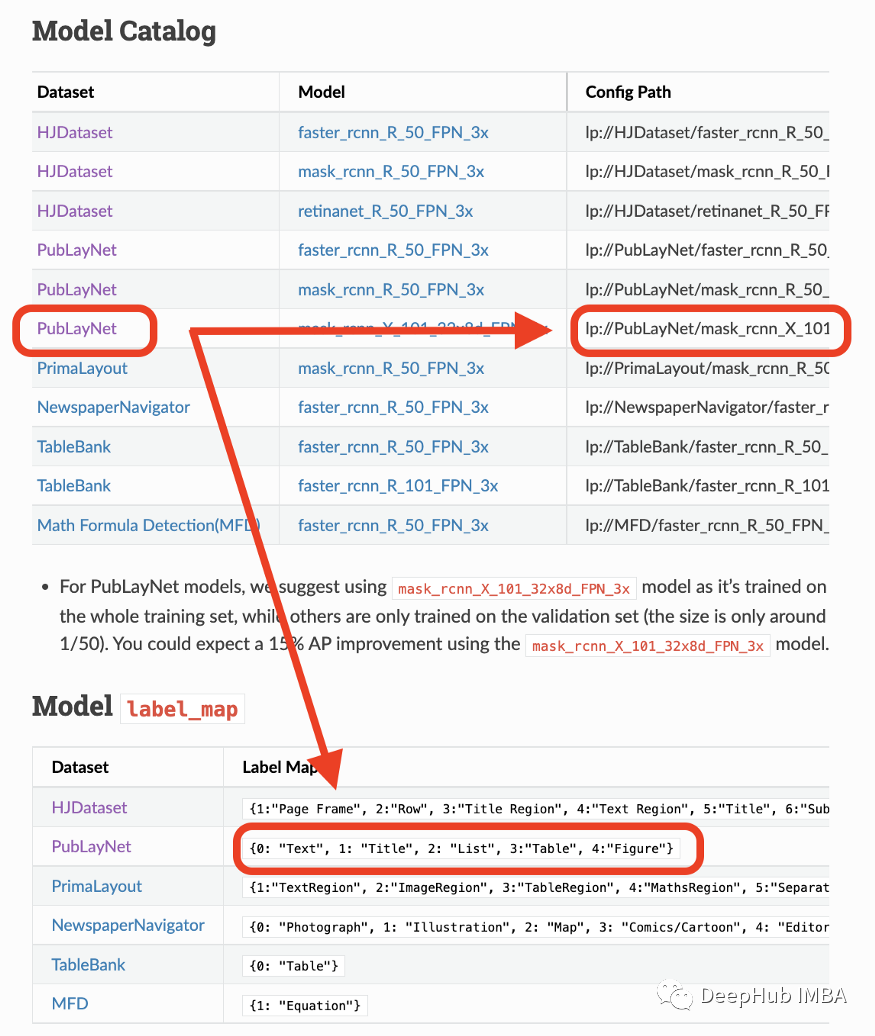
## load pre-trained model
model = lp.Detectron2LayoutModel(
"lp://PubLayNet/mask_rcnn_X_101_32x8d_FPN_3x/config",
extra_config=["MODEL.ROI_HEADS.SCORE_THRESH_TEST", 0.8],
label_map={0:"Text", 1:"Title", 2:"List", 3:"Table", 4:"Figure"})
## turn img into array
i = 21
img = np.asarray(doc[i])
## predict
detected = model.detect(img)
## plot
lp.draw_box(img, detected, box_width=5, box_alpha=0.2,
show_element_type=True)
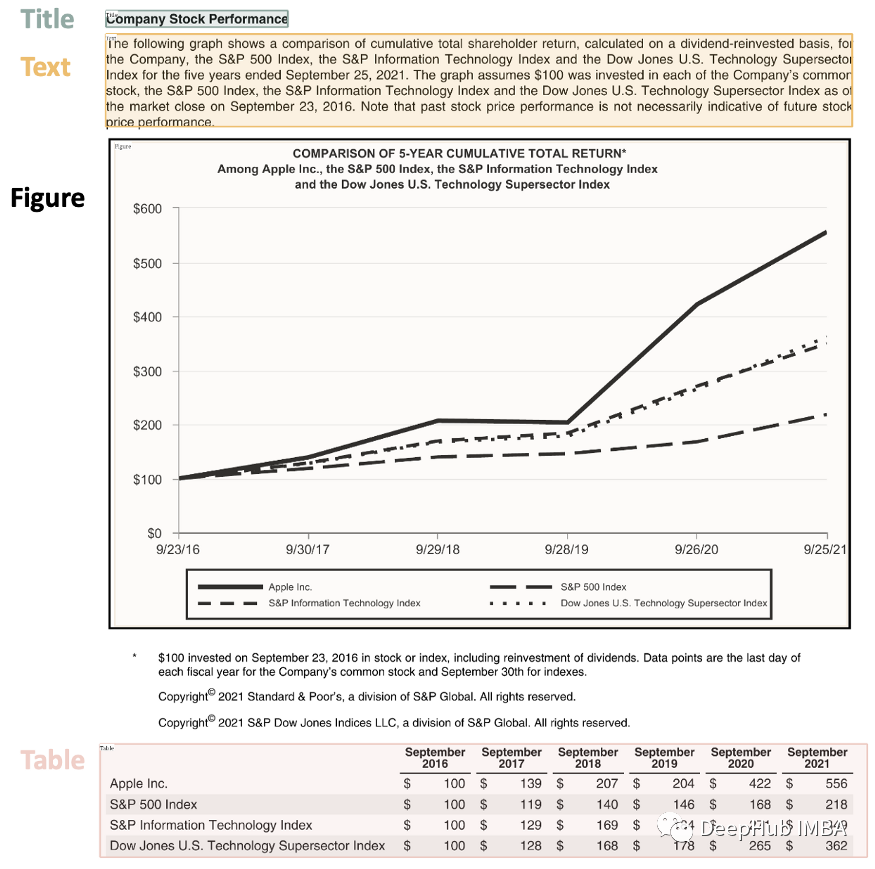
## sort
new_detected = detected.sort(key=lambda x: x.coordinates[1])
## assign ids
detected = lp.Layout([block.set(id=idx) for idx,block in
enumerate(new_detected)])## check
for block in detected:
print("---", str(block.id)+":", block.type, "---")
print(block, end='\n\n')
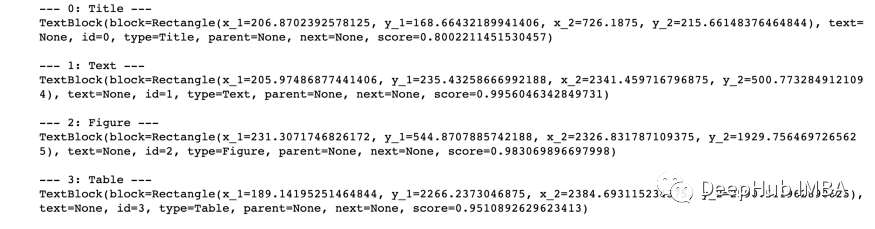
提取
'''
{'0-Title': '...',
'1-Text': '...',
'2-Figure': array([[ [0,0,0], ...]]),
'3-Table': pd.DataFrame,
}
'''
def parse_doc(dic):
for k,v in dic.items():
if "Title" in k:
print('\x1b[1;31m'+ v +'\x1b[0m')
elif "Figure" in k:
plt.figure(figsize=(10,5))
plt.imshow(v)
plt.show()
else:
print(v)
print(" ")
# load model
model = lp.TesseractAgent(languages='eng')
dic_predicted = {}
for block in [block for block in detected if block.type in ["Title","Text"]]:
## segmentation
segmented = block.pad(left=15, right=15, top=5,
bottom=5).crop_image(img)
## extraction
extracted = model.detect(segmented)
## save
dic_predicted[str(block.id)+"-"+block.type] =
extracted.replace('\n',' ').strip()
# check
parse_doc(dic_predicted)

for block in [block for block in detected if block.type == "Figure"]:
## segmentation
segmented = block.pad(left=15, right=15, top=5,
bottom=5).crop_image(img)
## save
dic_predicted[str(block.id)+"-"+block.type] = segmented
# check
parse_doc(dic_predicted)
for block in [block for block in detected if block.type == "Table"]:
## segmentation
segmented = block.pad(left=15, right=15, top=5,
bottom=5).crop_image(img)
## extraction
extracted = model.detect(segmented)
## save
dic_predicted[str(block.id)+"-"+block.type] = pd.read_csv(
io.StringIO(extracted) )
# check
parse_doc(dic_predicted)
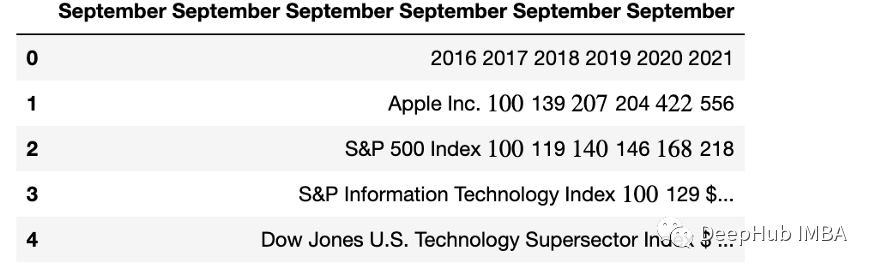
import tabula
tables = tabula.read_pdf("doc_apple.pdf", pages=i+1)
tables[0]

总结
本文的源代码:
https://github.com/mdipietro09/DataScience_ArtificialIntelligence_Utils/blob/master/computer_vision/example_ocr_parsing.ipynb
如果你安装Tesseract有问题的话,请看这个帖子
https://stackoverflow.com/questions/50951955/pytesseract-tesseractnotfound-error-tesseract-is-not-installed-or-its-not-i
编辑:于腾凯
评论