我用 PyTorch 复现了 LeNet-5 神经网络(CIFAR10 数据集篇)!
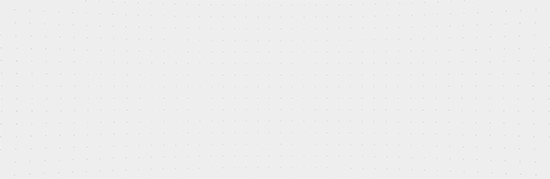
极市导读
大家好,我是红色石头!今天我们将使用 Pytorch 来继续实现 LeNet-5 模型,并用它来解决 CIFAR10 数据集的识别。 >>加入极市CV技术交流群,走在计算机视觉的最前沿
正文开始!
LeNet-5 网络本是用来识别 MNIST 数据集的,下面我们来将 LeNet-5 应用到一个比较复杂的例子,识别 CIFAR-10 数据集。
CIFAR-10 是由 Hinton 的学生 Alex Krizhevsky 和 Ilya Sutskever 整理的一个用于识别普适物体的小型数据集。一共包含 10 个类别的 RGB 彩色图 片:飞机( airlane )、汽车( automobile )、鸟类( bird )、猫( cat )、鹿( deer )、狗( dog )、蛙类( frog )、马( horse )、船( ship )和卡车( truck )。图片的尺寸为 32×32 ,数据集中一共有 50000 张训练圄片和 10000 张测试图片。
CIFAR-10 的图片样例如图所示。
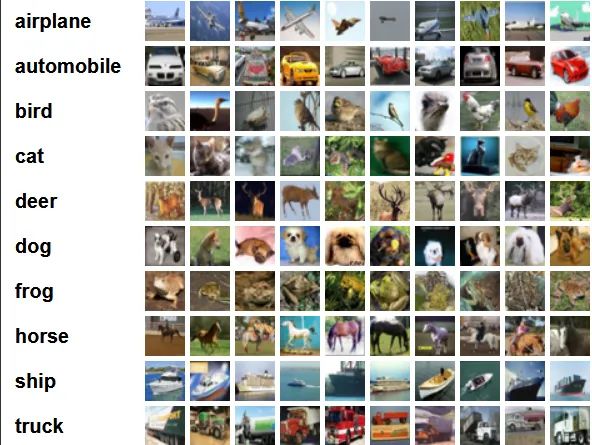
1 下载并加载数据,并做出一定的预先处理
pipline_train = transforms.Compose([
#随机旋转图片
transforms.RandomHorizontalFlip(),
#将图片尺寸resize到32x32
transforms.Resize((32,32)),
#将图片转化为Tensor格式
transforms.ToTensor(),
#正则化(当模型出现过拟合的情况时,用来降低模型的复杂度)
transforms.Normalize((0.5, 0.5, 0.5), (0.5, 0.5, 0.5))
])
pipline_test = transforms.Compose([
#将图片尺寸resize到32x32
transforms.Resize((32,32)),
transforms.ToTensor(),
transforms.Normalize((0.5, 0.5, 0.5), (0.5, 0.5, 0.5))
])
#下载数据集
train_set = datasets.CIFAR10(root="./data/CIFAR10", train=True, download=True, transform=pipline_train)
test_set = datasets.CIFAR10(root="./data/CIFAR10", train=False, download=True, transform=pipline_test)
#加载数据集
trainloader = torch.utils.data.DataLoader(train_set, batch_size=64, shuffle=True)
testloader = torch.utils.data.DataLoader(test_set, batch_size=32, shuffle=False)
# 类别信息也是需要我们给定的
classes = ('plane', 'car', 'bird', 'cat','deer', 'dog', 'frog', 'horse', 'ship', 'truck')
2 搭建 LeNet-5 神经网络结构,并定义前向传播的过程
LeNet-5 网络上文已经搭建过了,由于 CIFAR10 数据集图像是 RGB 三通道的,因此 LeNet-5 网络 C1 层卷积选择的滤波器需要 3 通道,网络其它结构跟上文都是一样的。
class LeNetRGB(nn.Module):
def __init__(self):
super(LeNetRGB, self).__init__()
self.conv1 = nn.Conv2d(3, 6, 5) # 3表示输入是3通道
self.relu = nn.ReLU()
self.maxpool1 = nn.MaxPool2d(2, 2)
self.conv2 = nn.Conv2d(6, 16, 5)
self.maxpool2 = nn.MaxPool2d(2, 2)
self.fc1 = nn.Linear(16*5*5, 120)
self.fc2 = nn.Linear(120, 84)
self.fc3 = nn.Linear(84, 10)
def forward(self, x):
x = self.conv1(x)
x = self.relu(x)
x = self.maxpool1(x)
x = self.conv2(x)
x = self.maxpool2(x)
x = x.view(-1, 16*5*5)
x = F.relu(self.fc1(x))
x = F.relu(self.fc2(x))
x = self.fc3(x)
output = F.log_softmax(x, dim=1)
return output
3 将定义好的网络结构搭载到 GPU/CPU,并定义优化器
使用 SGD(随机梯度下降)优化,学习率为 0.001,动量为 0.9。
#创建模型,部署gpu
device = torch.device("cuda" if torch.cuda.is_available() else "cpu")
model = LeNetRGB().to(device)
#定义优化器
optimizer = optim.SGD(model.parameters(), lr=0.01, momentum=0.9)
4 定义训练过程
def train_runner(model, device, trainloader, optimizer, epoch):
#训练模型, 启用 BatchNormalization 和 Dropout, 将BatchNormalization和Dropout置为True
model.train()
total = 0
correct =0.0
#enumerate迭代已加载的数据集,同时获取数据和数据下标
for i, data in enumerate(trainloader, 0):
inputs, labels = data
#把模型部署到device上
inputs, labels = inputs.to(device), labels.to(device)
#初始化梯度
optimizer.zero_grad()
#保存训练结果
outputs = model(inputs)
#计算损失和
#多分类情况通常使用cross_entropy(交叉熵损失函数), 而对于二分类问题, 通常使用sigmod
loss = F.cross_entropy(outputs, labels)
#获取最大概率的预测结果
#dim=1表示返回每一行的最大值对应的列下标
predict = outputs.argmax(dim=1)
total += labels.size(0)
correct += (predict == labels).sum().item()
#反向传播
loss.backward()
#更新参数
optimizer.step()
if i % 1000 == 0:
#loss.item()表示当前loss的数值
print("Train Epoch{} \t Loss: {:.6f}, accuracy: {:.6f}%".format(epoch, loss.item(), 100*(correct/total)))
Loss.append(loss.item())
Accuracy.append(correct/total)
return loss.item(), correct/total
5 定义测试过程
def test_runner(model, device, testloader):
#模型验证, 必须要写, 否则只要有输入数据, 即使不训练, 它也会改变权值
#因为调用eval()将不启用 BatchNormalization 和 Dropout, BatchNormalization和Dropout置为False
model.eval()
#统计模型正确率, 设置初始值
correct = 0.0
test_loss = 0.0
total = 0
#torch.no_grad将不会计算梯度, 也不会进行反向传播
with torch.no_grad():
for data, label in testloader:
data, label = data.to(device), label.to(device)
output = model(data)
test_loss += F.cross_entropy(output, label).item()
predict = output.argmax(dim=1)
#计算正确数量
total += label.size(0)
correct += (predict == label).sum().item()
#计算损失值
print("test_avarage_loss: {:.6f}, accuracy: {:.6f}%".format(test_loss/total, 100*(correct/total)))
6 运行
#调用
epoch = 20
Loss = []
Accuracy = []
for epoch in range(1, epoch+1):
print("start_time",time.strftime('%Y-%m-%d %H:%M:%S',time.localtime(time.time())))
loss, acc = train_runner(model, device, trainloader, optimizer, epoch)
Loss.append(loss)
Accuracy.append(acc)
test_runner(model, device, testloader)
print("end_time: ",time.strftime('%Y-%m-%d %H:%M:%S',time.localtime(time.time())),'\n')
print('Finished Training')
plt.subplot(2,1,1)
plt.plot(Loss)
plt.title('Loss')
plt.show()
plt.subplot(2,1,2)
plt.plot(Accuracy)
plt.title('Accuracy')
plt.show()
经历 20 次 epoch 迭代训练之后:
start_time 2021-11-27 22:29:09
Train Epoch20 Loss: 0.659028, accuracy: 68.750000%
test_avarage_loss: 0.030969, accuracy: 67.760000%
end_time: 2021-11-27 22:29:44
训练集的 loss 曲线和 Accuracy 曲线变化如下:
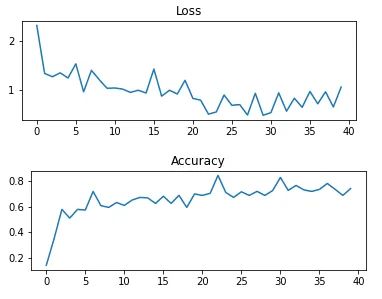
7 保存模型
print(model)
torch.save(model, './models/model-cifar10.pth') #保存模型
LeNet-5 的模型会 print 出来,并将模型模型命令为 model-cifar10.pth 保存在固定目录下。
LeNetRGB(
(conv1): Conv2d(3, 6, kernel_size=(5, 5), stride=(1, 1))
(relu): ReLU()
(maxpool1): MaxPool2d(kernel_size=2, stride=2, padding=0, dilation=1, ceil_mode=False)
(conv2): Conv2d(6, 16, kernel_size=(5, 5), stride=(1, 1))
(maxpool2): MaxPool2d(kernel_size=2, stride=2, padding=0, dilation=1, ceil_mode=False)
(fc1): Linear(in_features=400, out_features=120, bias=True)
(fc2): Linear(in_features=120, out_features=84, bias=True)
(fc3): Linear(in_features=84, out_features=10, bias=True)
)
8 模型测试
利用刚刚训练的模型进行 CIFAR10 类型图片的测试。
from PIL import Image
import numpy as np
if __name__ == '__main__':
device = torch.device('cuda' if torch.cuda.is_available() else 'cpu')
model = torch.load('./models/model-cifar10.pth') #加载模型
model = model.to(device)
model.eval() #把模型转为test模式
#读取要预测的图片
# 读取要预测的图片
img = Image.open("./images/test_cifar10.png").convert('RGB') # 读取图像
#img.show()
plt.imshow(img) # 显示图片
plt.axis('off') # 不显示坐标轴
plt.show()
# 导入图片,图片扩展后为[1,1,32,32]
trans = transforms.Compose(
[
#将图片尺寸resize到32x32
transforms.Resize((32,32)),
transforms.ToTensor(),
transforms.Normalize((0.5, 0.5, 0.5), (0.5, 0.5, 0.5))
])
img = trans(img)
img = img.to(device)
img = img.unsqueeze(0) #图片扩展多一维,因为输入到保存的模型中是4维的[batch_size,通道,长,宽],而普通图片只有三维,[通道,长,宽]
# 预测
classes = ('plane', 'car', 'bird', 'cat', 'deer', 'dog', 'frog', 'horse', 'ship', 'truck')
output = model(img)
prob = F.softmax(output,dim=1) #prob是10个分类的概率
print("概率:",prob)
print(predict.item())
value, predicted = torch.max(output.data, 1)
predict = output.argmax(dim=1)
pred_class = classes[predicted.item()]
print("预测类别:",pred_class)
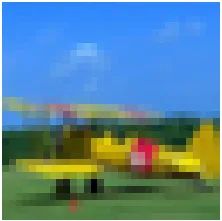
输出:
概率:tensor([[7.6907e-01, 3.3997e-03, 4.8003e-03, 4.2978e-05, 1.2168e-02, 6.8751e-06, 3.2019e-06, 1.6024e-04, 1.2705e-01, 8.3300e-02]],
grad_fn=)
5
预测类别:plane
模型预测结果正确!
以上就是 PyTorch 构建 LeNet-5 卷积神经网!络并用它来识别 CIFAR10 数据集的例子。全文的代码都是可以顺利运行的,建议大家自己跑一边。
值得一提的是,针对 MNIST 数据集和 CIFAR10 数据集,最大的不同就是 MNIST 是单通道的,CIFAR10 是三通道的,因此在构建 LeNet-5 网络的时候,C1层需要做不同的设置。至于输入图片尺寸不一样,我们可以使用 transforms.Resize 方法统一缩放到 32x32 的尺寸大小。
所有完整的代码我都放在 GitHub 上,GitHub地址为:https://github.com/RedstoneWill/ObjectDetectionLearner/tree/main/LeNet-5
如果觉得有用,就请分享到朋友圈吧!
公众号后台回复“transformer”获取最新Transformer综述论文下载~

# CV技术社群邀请函 #
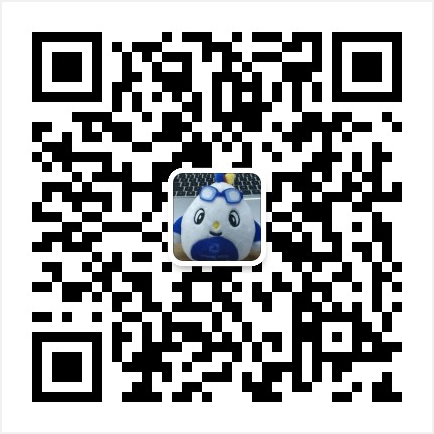
备注:姓名-学校/公司-研究方向-城市(如:小极-北大-目标检测-深圳)
即可申请加入极市目标检测/图像分割/工业检测/人脸/医学影像/3D/SLAM/自动驾驶/超分辨率/姿态估计/ReID/GAN/图像增强/OCR/视频理解等技术交流群
每月大咖直播分享、真实项目需求对接、求职内推、算法竞赛、干货资讯汇总、与 10000+来自港科大、北大、清华、中科院、CMU、腾讯、百度等名校名企视觉开发者互动交流~
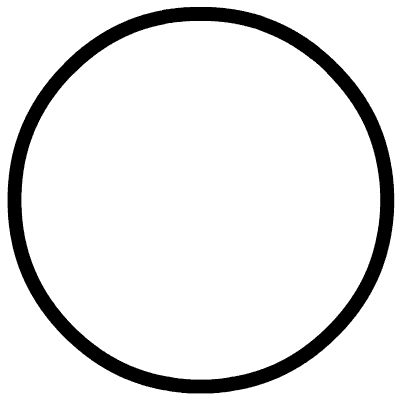