机器学习中的19 种损失函数,你使用过几种?
作者:mingo_敏
https://blog.csdn.net/shanglianlm/article/details/85019768
来自:深度学习自然语言处理
tensorflow和pytorch很多都是相似的,这里以pytorch为例。
1. L1范数损失 L1Loss
torch.nn.L1Loss(reduction='mean')
reduction-三个值,none: 不使用约简;mean:返回loss和的平均值;sum:返回loss的和。默认:mean。
torch.nn.MSELoss(reduction='mean')
reduction-三个值,none: 不使用约简;mean:返回loss和的平均值;sum:返回loss的和。默认:mean。

torch.nn.CrossEntropyLoss(weight=None,ignore_index=-100, reduction='mean')
weight (Tensor, optional) – 自定义的每个类别的权重. 必须是一个长度为 C 的 Tensor ignore_index (int, optional) – 设置一个目标值, 该目标值会被忽略, 从而不会影响到 输入的梯度。 reduction-三个值,none: 不使用约简;mean:返回loss和的平均值;sum:返回loss的和。默认:mean。
torch.nn.KLDivLoss(reduction='mean')
reduction-三个值,none: 不使用约简;mean:返回loss和的平均值;sum:返回loss的和。默认:mean。
torch.nn.BCELoss(weight=None, reduction='mean')
weight (Tensor, optional) – 自定义的每个 batch 元素的 loss 的权重. 必须是一个长度为 “nbatch” 的 的 Tensor
torch.nn.BCEWithLogitsLoss(weight=None, reduction='mean', pos_weight=None)
weight (Tensor, optional) – 自定义的每个 batch 元素的 loss 的权重. 必须是一个长度 为 “nbatch” 的 Tensor
7 MarginRankingLoss
torch.nn.MarginRankingLoss(margin=0.0, reduction='mean')

参数:
margin:默认值0
8 HingeEmbeddingLoss
torch.nn.HingeEmbeddingLoss(margin=1.0, reduction='mean')
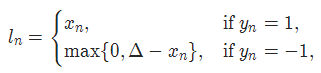
参数:
margin:默认值1
9 多标签分类损失 MultiLabelMarginLoss
torch.nn.MultiLabelMarginLoss(reduction='mean')

10 平滑版L1损失 SmoothL1Loss
torch.nn.SmoothL1Loss(reduction='mean')
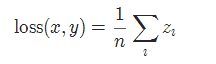
其中

11 2分类的logistic损失 SoftMarginLoss
torch.nn.SoftMarginLoss(reduction='mean')

12 多标签 one-versus-all 损失 MultiLabelSoftMarginLoss
torch.nn.MultiLabelSoftMarginLoss(weight=None, reduction='mean')

13 cosine 损失 CosineEmbeddingLoss
torch.nn.CosineEmbeddingLoss(margin=0.0, reduction='mean')

参数:
margin:默认值0
14 多类别分类的hinge损失 MultiMarginLoss
torch.nn.MultiMarginLoss(p=1, margin=1.0, weight=None, reduction='mean')

参数:
p=1或者2 默认值:1
margin:默认值1
15 三元组损失 TripletMarginLoss
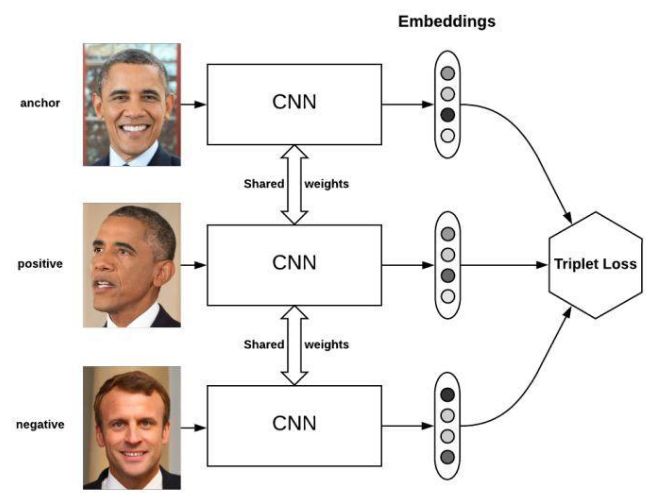
torch.nn.TripletMarginLoss(margin=1.0, p=2.0, eps=1e-06, swap=False, reduction='mean')

其中:

torch.nn.CTCLoss(blank=0, reduction='mean')
reduction-三个值,none: 不使用约简;mean:返回loss和的平均值;sum:返回loss的和。默认:mean。
torch.nn.NLLLoss(weight=None, ignore_index=-100, reduction='mean')
weight (Tensor, optional) – 自定义的每个类别的权重. 必须是一个长度为 C 的 Tensor ignore_index (int, optional) – 设置一个目标值, 该目标值会被忽略, 从而不会影响到 输入的梯度.
torch.nn.NLLLoss2d(weight=None, ignore_index=-100, reduction='mean')
weight (Tensor, optional) – 自定义的每个类别的权重. 必须是一个长度为 C 的 Tensor reduction-三个值,none: 不使用约简;mean:返回loss和的平均值;sum:返回loss的和。默认:mean。
torch.nn.PoissonNLLLoss(log_input=True, full=False, eps=1e-08, reduction='mean')
log_input (bool, optional) – 如果设置为 True , loss 将会按照公 式 exp(input) - target * input 来计算, 如果设置为 False , loss 将会按照 input - target * log(input+eps) 计算. full (bool, optional) – 是否计算全部的 loss, i. e. 加上 Stirling 近似项 target * log(target) - target + 0.5 * log(2 * pi * target). eps (float, optional) – 默认值: 1e-8
评论