【Python基础】Python数据分析实战之分布分析
机器学习初学者
共 3475字,需浏览 7分钟
· 2020-09-14
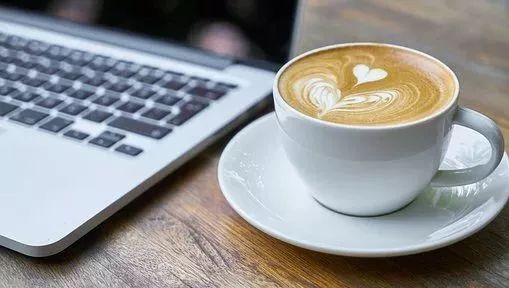
◆ ◆ ◆ ◆ ◆
import pandas as pd
import matplotlib.pyplot as plt
import math
>>> df = pd.read_csv('UserInfo.csv')
>>> df.info()
RangeIndex: 1000000 entries, 0 to 999999
Data columns (total 4 columns):
UserId 1000000 non-null int64
CardId 1000000 non-null int64
LoginTime 1000000 non-null object
DeviceType 1000000 non-null object
dtypes: int64(2), object(2)
memory usage: 30.5+ MB
# 提取出生日期需要先把身份证号码转换成字符串
'CardId'] = df['CardId'].astype('str') > df[
# 提取出生日期,并生成新字段
'DateofBirth'] = df.CardId.apply(lambda x : x[6:10]+"-"+x[10:12]+"-"+x[12:14]) > df[
# 提取性别,待观察性别分布
'Gender'] = df['CardId'].map(lambda x : 'Male' if int(x[-2]) % 2 else 'Female') > df[
> df.head()
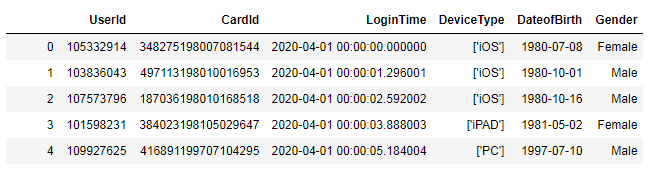
3.计算年龄
# 提取出生日期:月和日
>>> df[['month','day']] = df['DateofBirth'].str.split('-',expand=True).loc[:,1:2]
# 提取小月,查看是否有31号
>>> df_small_month = df[df['month'].isin(['02','04','06','09','11'])]
# 无效数据,如图所示
>>> df_small_month[df_small_month['day']=='31']
# 统统删除,均为无效数据
>>> df.drop(df_small_month[df_small_month['day']=='31'].index,inplace=True)
# 同理,校验2月
>>> df_2 = df[df['month']=='02']
# 2月份的校验大家可以做的仔细点儿,先判断是否润年再进行删减
>>> df_2[df_2['day'].isin(['29','30','31'])]
# 统统删除
>>> df.drop(df_2[df_2['day'].isin(['29','30','31'])].index,inplace=True)
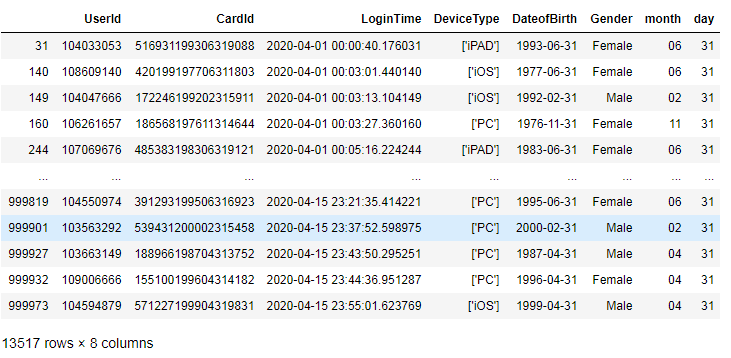
# 计算年龄
# 方法一
'Age'] = df['DateofBirth'].apply(lambda x : math.floor((pd.datetime.now() - pd.to_datetime(x)).days/365)) df[
# 方法二
'DateofBirth'].apply(lambda x : pd.datetime.now().year - pd.to_datetime(x).year) df[
# 查看年龄区间,进行分区
>>> df['Age'].max(),df['Age'].min()
# (45, 18)
>>> bins = [0,18,25,30,35,40,100]
>>> labels = ['18岁及以下','19岁到25岁','26岁到30岁','31岁到35岁','36岁到40岁','41岁及以上']
>>> df['年龄分层'] = pd.cut(df['Age'],bins, labels = labels)
# 查看是否有重复值
>>> df.duplicated('UserId').sum() #47681
# 数据总条目
>>> df.count() #980954
>>> df.groupby('年龄分层')['UserId'].count()
年龄分层
18岁及以下 25262
19岁到25岁 254502
26岁到30岁 181751
31岁到35岁 181417
36岁到40岁 181589
41岁及以上 156433
Name: UserId, dtype: int64
# 通过求和,可知重复数据也被计算进去
>>> df.groupby('年龄分层')['UserId'].count().sum()
# 980954
>>> df.groupby('年龄分层')['UserId'].nunique()
年龄分层
18岁及以下 24014
19岁到25岁 242199
26岁到30岁 172832
31岁到35岁 172608
36岁到40岁 172804
41岁及以上 148816
Name: UserId, dtype: int64
>>> df.groupby('年龄分层')['UserId'].nunique().sum()
# 933273 = 980954(总)-47681(重复)
# 计算年龄分布
>>> result = df.groupby('年龄分层')['UserId'].nunique()/df.groupby('年龄分层')['UserId'].nunique().sum()
>>> result
# 结果
年龄分层
18岁及以下 0.025731
19岁到25岁 0.259516
26岁到30岁 0.185189
31岁到35岁 0.184949
36岁到40岁 0.185159
41岁及以上 0.159456
Name: UserId, dtype: float64
# 格式化一下
>>> result = round(result,4)*100
>>> result.map("{:.2f}%".format)
年龄分层
18岁及以下 2.57%
19岁到25岁 25.95%
26岁到30岁 18.52%
31岁到35岁 18.49%
36岁到40岁 18.52%
41岁及以上 15.95%
Name: UserId, dtype: object
往期精彩回顾
获取一折本站知识星球优惠券,复制链接直接打开:
https://t.zsxq.com/662nyZF
本站qq群1003271085。
加入微信群请扫码进群(如果是博士或者准备读博士请说明):
评论
英伟达Blackwell平台网络配置分析
本文来自“英伟达Blachwell平台网络配置详解”。GTC大会英伟达展示了全新的 Blackwell 平台系列产品,包括 HGX B100 服务器、NVLINK Switch、GB200Superchip Computer Node、Quantum X800 交换机和 CX8 网卡(InfiniB
架构师技术联盟
0
金融研究 | 使用Python测量关键审计事项的「信息含量」
Tips: 公众号推送后内容只能更改一次,且只能改20字符。如果内容出问题,或者想更新内容, 只能重复推送。为了更好的阅读体验,建议阅读本文博客版, 链接地址https://textdata.cn/blog/2023-01-13-information-content-of-critical-aud
大邓和他的Python
0
CVPR 2024|大视觉模型的开山之作!无需任何语言数据即可打造大视觉模型
↑ 点击蓝字 关注极市平台作者丨科技猛兽编辑丨极市平台极市导读 本文提出一种序列建模 (sequential modeling) 的方法,不使用任何语言数据,训练大视觉模型。>>加入极市CV技术交流群,走在计算机视觉的最前沿本文目录1 序列建模打造大视觉模型(来自 U
极市平台
1
金融研究(更新) | 使用Python构建关键审计事项的「信息含量」
Tips: 公众号推送后内容只能更改一次,且只能改20字符。如果内容出问题,或者想更新内容, 只能重复推送。为了更好的阅读体验,建议阅读本文博客版, 链接地址https://textdata.cn/blog/2023-01-13-information-content-of-critical-aud
大邓和他的Python
0
谷歌员工爆料 Python 基础团队原地解散
转自 | 机器之心编辑 | 蛋酱什么?谷歌解雇了整个 Python 基础团队?「当与你直接共事的每个人,包括你的主管,都被裁员 —— 哦,是职位被削减,而你被要求安排他们的替代者入职,这些人被告知在不同的国家担任同样的职位,但他们并不为此感到高兴,这是很艰难的一天。」发布这一动态的 Tho
机器学习算法与Python实战
0
5000w+ 的大表如何拆?亿级别大表拆分实战复盘
前言笔者是在两年前接手公司的财务系统的开发和维护工作。在系统移交的初期,笔者和团队就发现,系统内有一张5000W+的大表。跟踪代码发现,该表是用于存储资金流水的表格,关联着众多功能点,同时也有众多的下游系统在使用这张表的数据。进一步的观察发现,这张表还在以每月600W+的数据持续增长,也就是说,不超
码农编程进阶笔记
0
管理世界2024 | 使用管理层讨论与分析测量「企业人工智能指标」
Tips: 公众号推送后内容只能更改一次,且只能改20字符。如果内容出问题,或者想更新内容, 只能重复推送。为了更好的阅读体验,建议阅读本文博客版, 链接地址 https://textdata.cn/blog/2024-04-19-ai-improve-firm-productivity/
大邓和他的Python
0
五一抢票难,Github上这几个Python项目,你可以试试
又到五一长假啦(虽然其实就放了1天),大家是打算家里蹲or出去玩,又或者是在公司加班呢...今天给大家介绍三个和12306相关的项目,看看你是否用得上。/01/ py12306py12306购票助手,顾名思义,12306买票的~需要在python 3.6以上版本运行程序。1. 安装依赖gi
Crossin的编程教室
0