机器学习还能预测心血管疾病?没错,我用Python写出来了
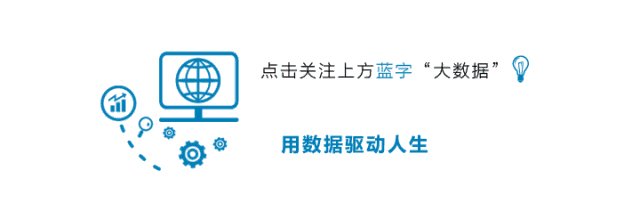
导读:手把手教你如何用Python写出心血管疾病预测模型。
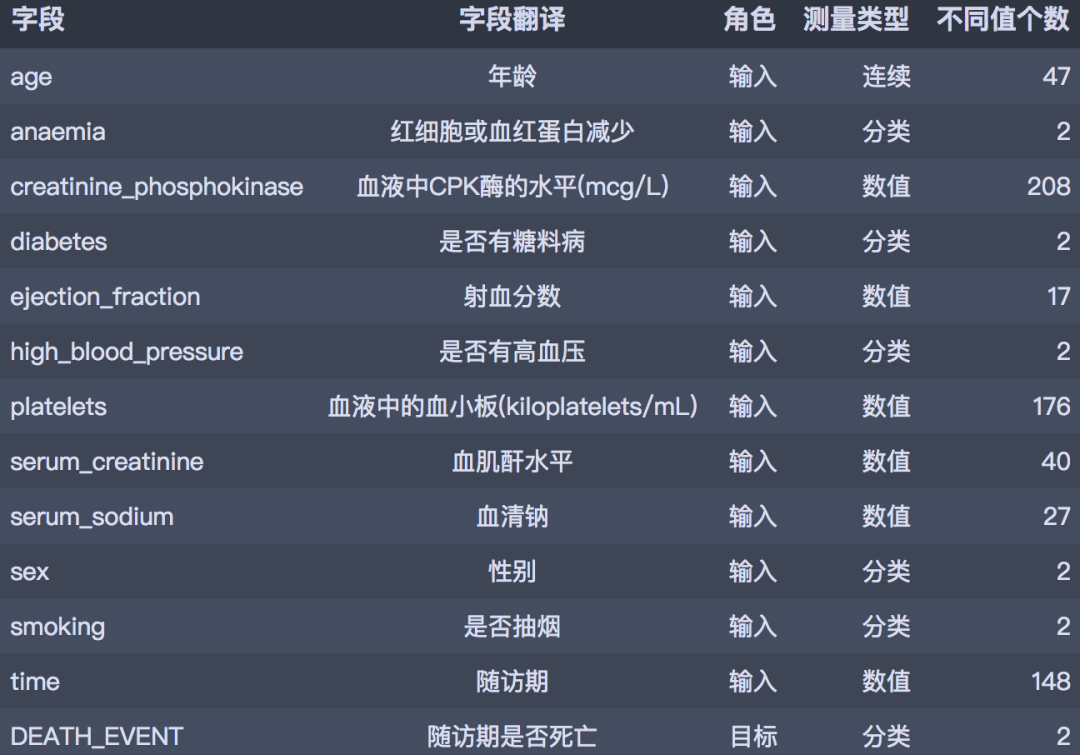
# 数据整理
import numpy as np
import pandas as pd
# 可视化
import matplotlib.pyplot as plt
import seaborn as sns
import plotly as py
import plotly.graph_objs as go
import plotly.express as px
import plotly.figure_factory as ff
# 模型建立
from sklearn.linear_model import LogisticRegression
from sklearn.tree import DecisionTreeClassifier
from sklearn.ensemble import GradientBoostingClassifier, RandomForestClassifier
import lightgbm
# 前处理
from sklearn.preprocessing import StandardScaler
# 模型评估
from sklearn.model_selection import train_test_split, GridSearchCV
from sklearn.metrics import plot_confusion_matrix, confusion_matrix, f1_score
# 读入数据
df = pd.read_csv('./data/heart_failure.csv')
df.head()

df.describe().T
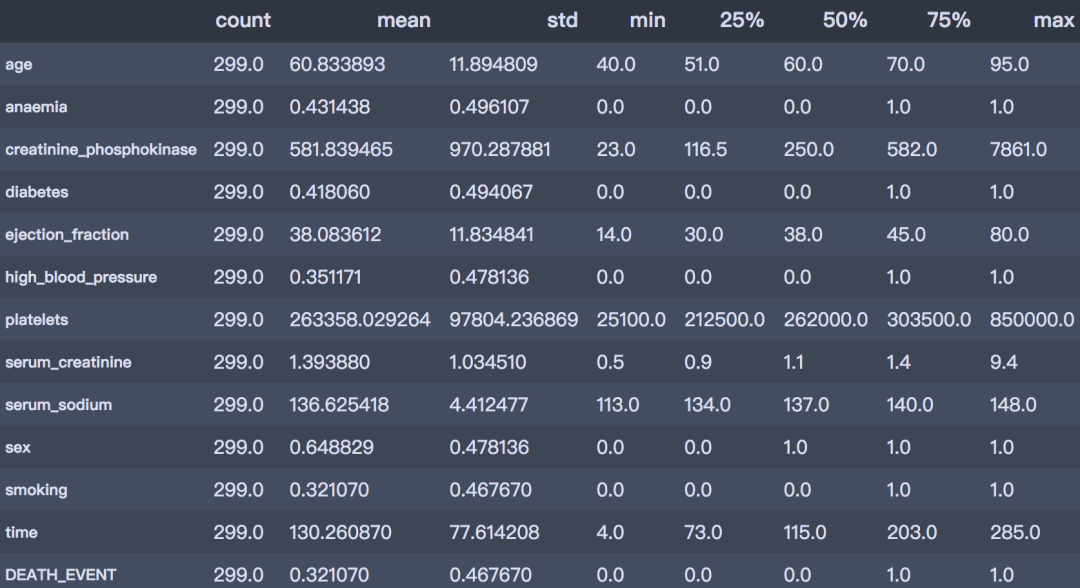
是否死亡:平均的死亡率为32%; 年龄分布:平均年龄60岁,最小40岁,最大95岁 是否有糖尿病:有41.8%患有糖尿病 是否有高血压:有35.1%患有高血压 是否抽烟:有32.1%有抽烟
# 产生数据
death_num = df['DEATH_EVENT'].value_counts()
death_num = death_num.reset_index()
# 饼图
fig = px.pie(death_num, names='index', values='DEATH_EVENT')
fig.update_layout(title_text='目标变量DEATH_EVENT的分布')
py.offline.plot(fig, filename='./html/目标变量DEATH_EVENT的分布.html')
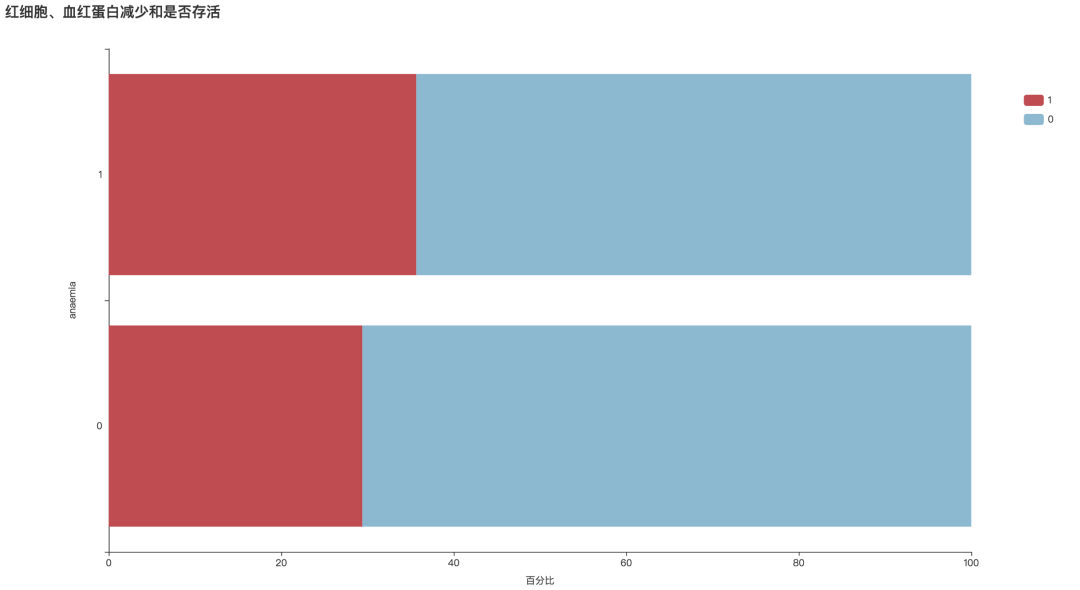
bar1 = draw_categorical_graph(df['anaemia'], df['DEATH_EVENT'], title='红细胞、血红蛋白减少和是否存活')
bar1.render('./html/红细胞血红蛋白减少和是否存活.html')
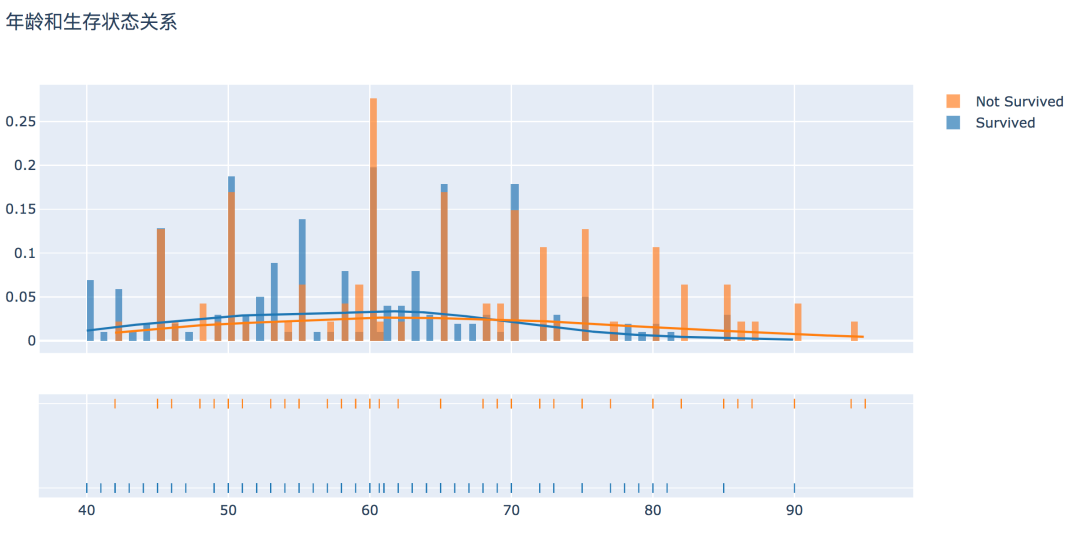
# 产生数据
surv = df[df['DEATH_EVENT'] == 0]['age']
not_surv = df[df['DEATH_EVENT'] == 1]['age']
hist_data = [surv, not_surv]
group_labels = ['Survived', 'Not Survived']
# 直方图
fig = ff.create_distplot(hist_data, group_labels, bin_size=0.5)
fig.update_layout(title_text='年龄和生存状态关系')
py.offline.plot(fig, filename='./html/年龄和生存状态关系.html')
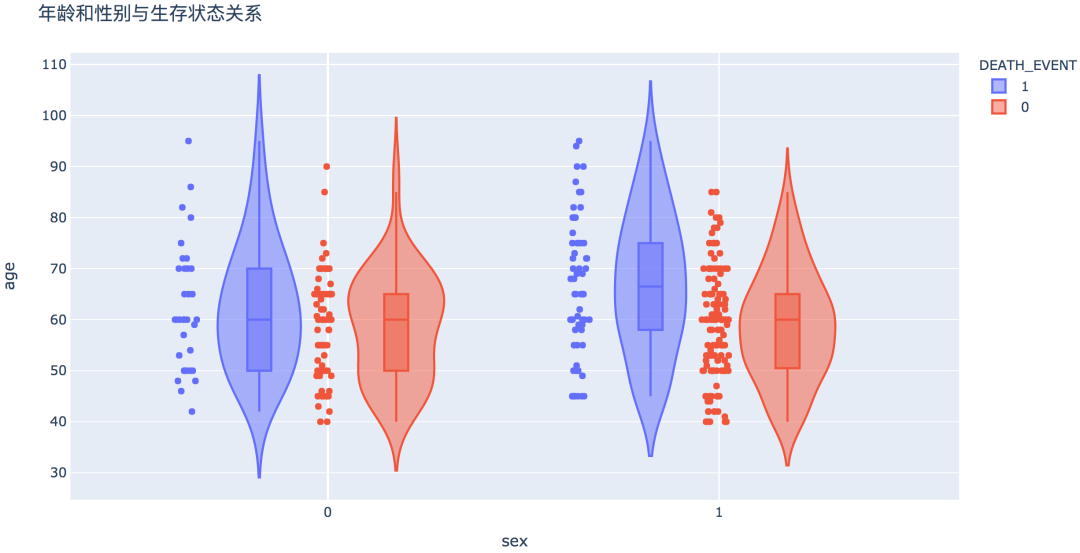
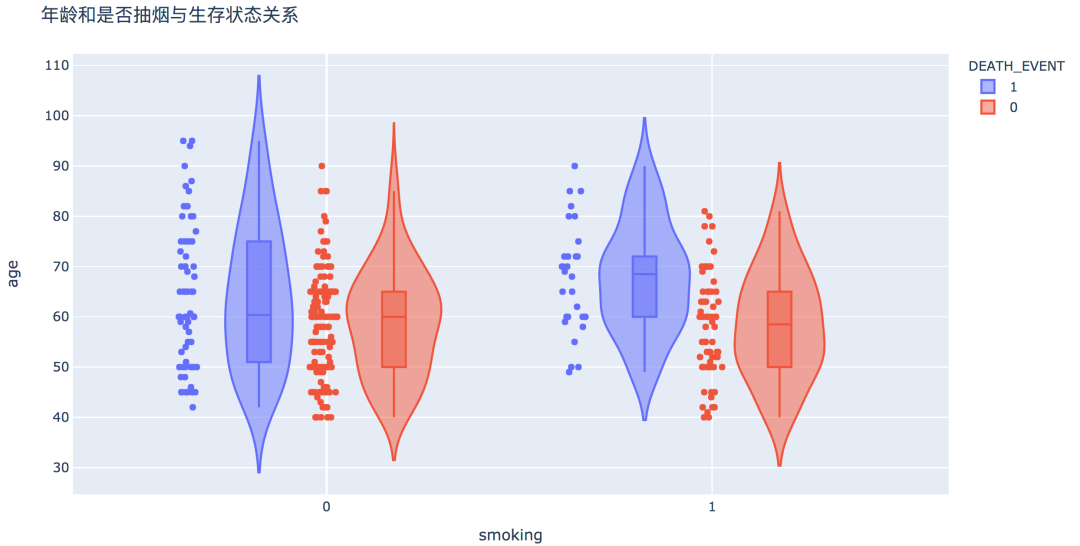
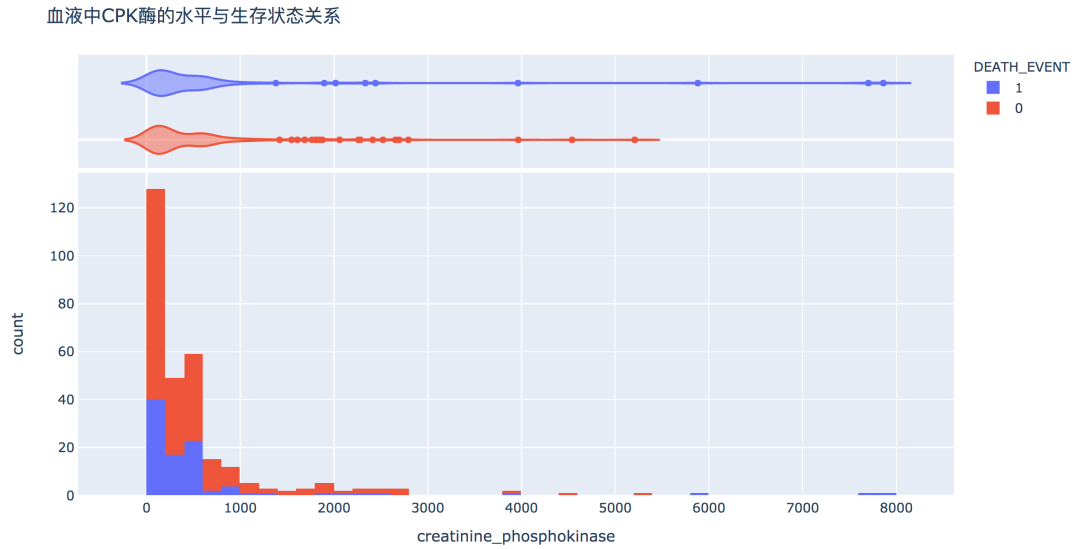
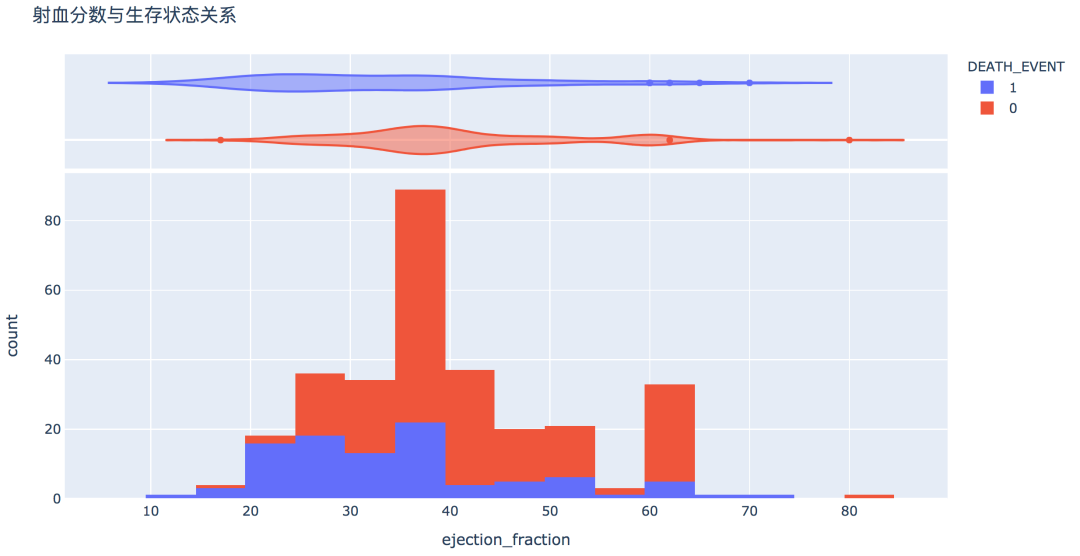
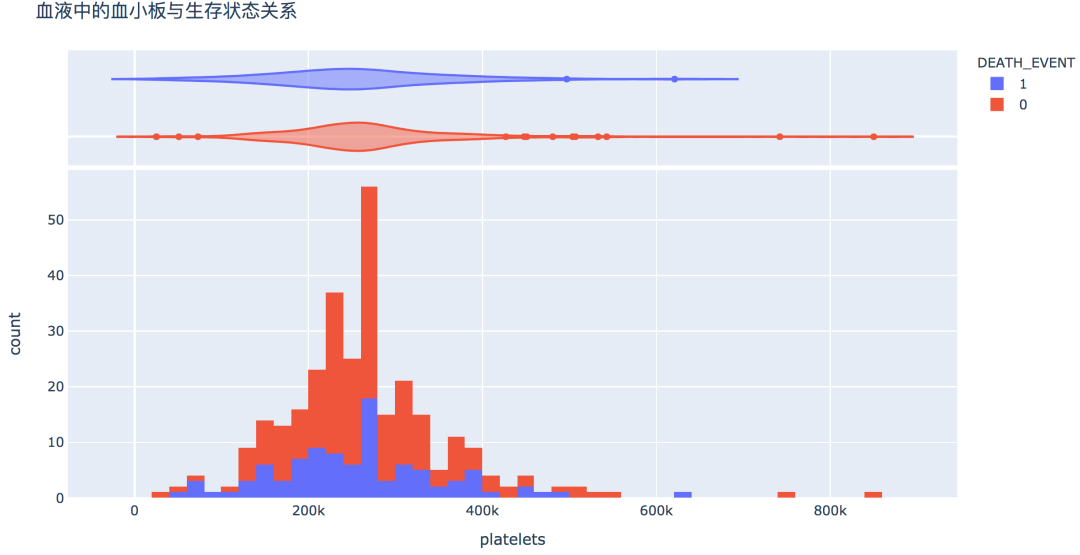
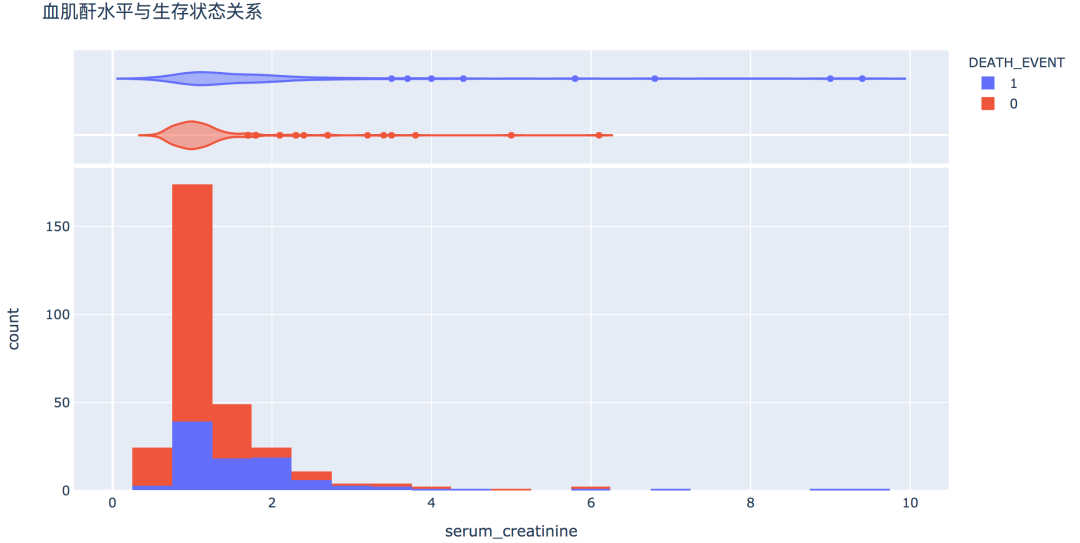
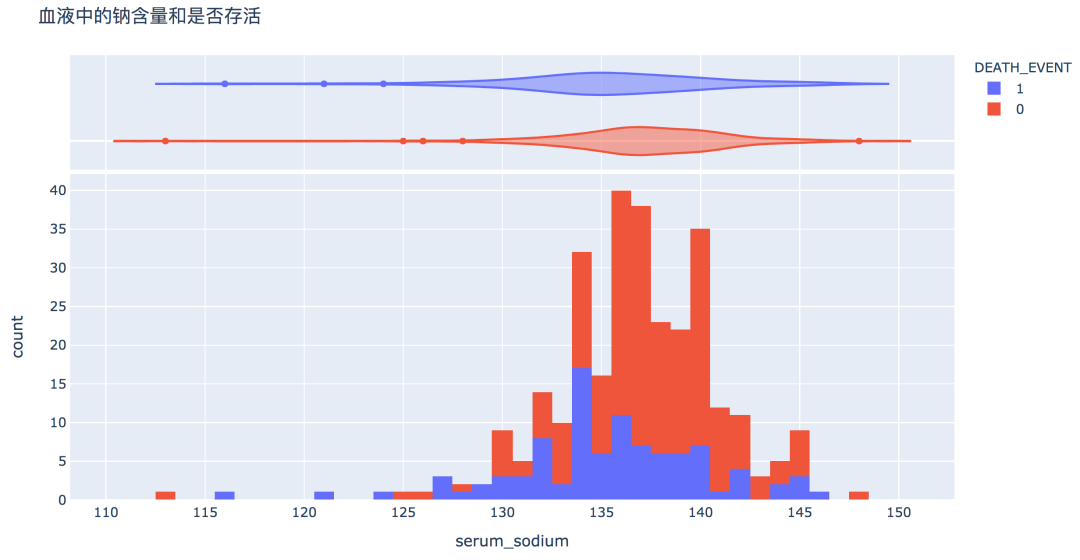
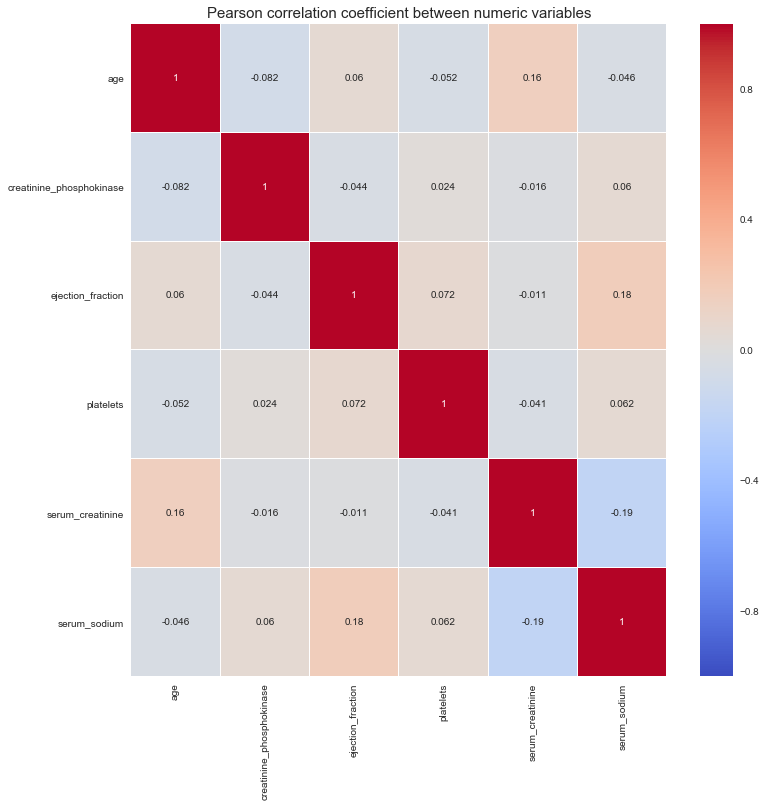
num_df = df[['age', 'creatinine_phosphokinase', 'ejection_fraction', 'platelets',
'serum_creatinine', 'serum_sodium']]
plt.figure(figsize=(12, 12))
sns.heatmap(num_df.corr(), vmin=-1, cmap='coolwarm', linewidths=0.1, annot=True)
plt.title('Pearson correlation coefficient between numeric variables', fontdict={'fontsize': 15})
plt.show()
# 划分X和y
X = df.drop('DEATH_EVENT', axis=1)
y = df['DEATH_EVENT']
from feature_selection import Feature_select
fs = Feature_select(num_method='anova', cate_method='kf')
X_selected = fs.fit_transform(X, y)
X_selected.head()
2020 17:19:49 INFO attr select success!
After select attr: ['serum_creatinine', 'serum_sodium', 'ejection_fraction', 'age', 'time']
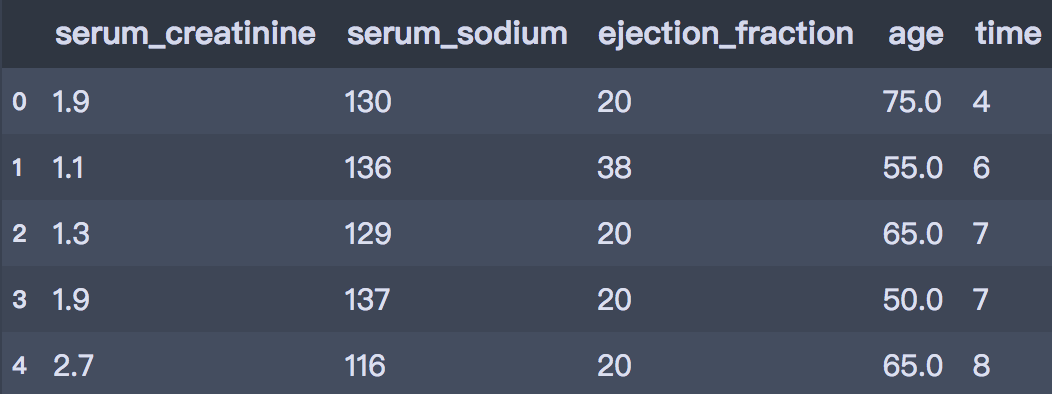
# 划分训练集和测试集
Features = X_selected.columns
X = df[Features]
y = df["DEATH_EVENT"]
X_train, X_test, y_train, y_test = train_test_split(X, y, test_size=0.2, stratify=y,
random_state=2020)
# 标准化
scaler = StandardScaler()
scaler_Xtrain = scaler.fit_transform(X_train)
scaler_Xtest = scaler.fit_transform(X_test)
lr = LogisticRegression()
lr.fit(scaler_Xtrain, y_train)
test_pred = lr.predict(scaler_Xtest)
# F1-score
print("F1_score of LogisticRegression is : ", round(f1_score(y_true=y_test, y_pred=test_pred),2))
# DecisionTreeClassifier
clf = DecisionTreeClassifier(criterion='gini', max_depth=5, random_state=1)
clf.fit(X_train, y_train)
test_pred = clf.predict(X_test)
# F1-score
print("F1_score of DecisionTreeClassifier is : ", round(f1_score(y_true=y_test, y_pred=test_pred),2))
# 绘图
plt.figure(figsize=(10, 7))
plot_confusion_matrix(clf, X_test, y_test, cmap='Blues')
plt.title("DecisionTreeClassifier - Confusion Matrix", fontsize=15)
plt.xticks(range(2), ["Heart Not Failed","Heart Fail"], fontsize=12)
plt.yticks(range(2), ["Heart Not Failed","Heart Fail"], fontsize=12)
plt.show()
F1_score of DecisionTreeClassifier is : 0.61
720x504 with 0 Axes>
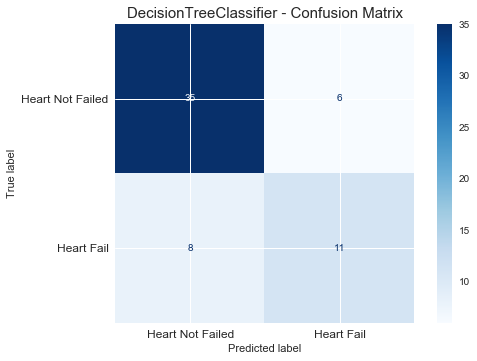
parameters = {'splitter':('best','random'),
'criterion':("gini","entropy"),
"max_depth":[*range(1, 20)],
}
clf = DecisionTreeClassifier(random_state=1)
GS = GridSearchCV(clf, param_grid=parameters, cv=10, scoring='f1', n_jobs=-1)
GS.fit(X_train, y_train)
print(GS.best_params_)
print(GS.best_score_)
{'criterion': 'entropy', 'max_depth': 3, 'splitter': 'best'}
0.7638956305132776
test_pred = GS.best_estimator_.predict(X_test)
# F1-score
print("F1_score of DecisionTreeClassifier is : ", round(f1_score(y_true=y_test, y_pred=test_pred),2))
# 绘图
plt.figure(figsize=(10, 7))
plot_confusion_matrix(GS, X_test, y_test, cmap='Blues')
plt.title("DecisionTreeClassifier - Confusion Matrix", fontsize=15)
plt.xticks(range(2), ["Heart Not Failed","Heart Fail"], fontsize=12)
plt.yticks(range(2), ["Heart Not Failed","Heart Fail"], fontsize=12)
plt.show()
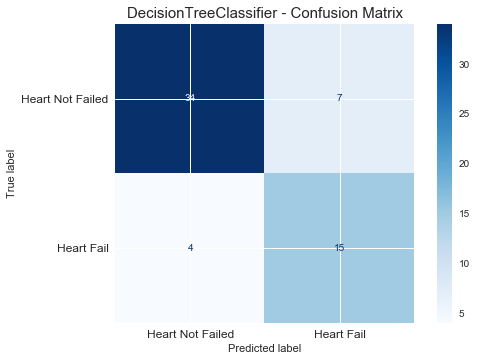
# RandomForestClassifier
rfc = RandomForestClassifier(n_estimators=1000, random_state=1)
parameters = {'max_depth': np.arange(2, 20, 1) }
GS = GridSearchCV(rfc, param_grid=parameters, cv=10, scoring='f1', n_jobs=-1)
GS.fit(X_train, y_train)
print(GS.best_params_)
print(GS.best_score_)
test_pred = GS.best_estimator_.predict(X_test)
# F1-score
print("F1_score of RandomForestClassifier is : ", round(f1_score(y_true=y_test, y_pred=test_pred),2))
{'max_depth': 3}
0.791157747481277
F1_score of RandomForestClassifier is : 0.53
gbl = GradientBoostingClassifier(n_estimators=1000, random_state=1)
parameters = {'max_depth': np.arange(2, 20, 1) }
GS = GridSearchCV(gbl, param_grid=parameters, cv=10, scoring='f1', n_jobs=-1)
GS.fit(X_train, y_train)
print(GS.best_params_)
print(GS.best_score_)
# 测试集
test_pred = GS.best_estimator_.predict(X_test)
# F1-score
print("F1_score of GradientBoostingClassifier is : ", round(f1_score(y_true=y_test, y_pred=test_pred),2))
{'max_depth': 3}
0.7288420428900305
F1_score of GradientBoostingClassifier is : 0.65
lgb_clf = lightgbm.LGBMClassifier(boosting_type='gbdt', random_state=1)
parameters = {'max_depth': np.arange(2, 20, 1) }
GS = GridSearchCV(lgb_clf, param_grid=parameters, cv=10, scoring='f1', n_jobs=-1)
GS.fit(X_train, y_train)
print(GS.best_params_)
print(GS.best_score_)
# 测试集
test_pred = GS.best_estimator_.predict(X_test)
# F1-score
print("F1_score of LGBMClassifier is : ", round(f1_score(y_true=y_test, y_pred=test_pred),2))
{'max_depth': 2}
0.780378102289867
F1_score of LGBMClassifier is : 0.74
LogisticRegression:0.63 DecisionTree Classifier:0.73 Random Forest Classifier: 0.53 GradientBoosting Classifier: 0.65 LGBM Classifier: 0.74

评论