通过关键点进行小目标检测的轻量开源库
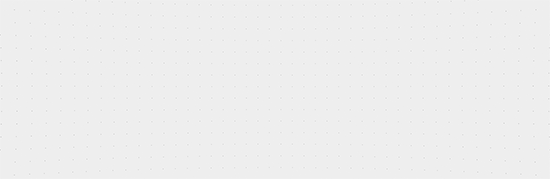
极市导读
关键点是一种应用广泛的技术,在人脸识别、人体姿态检测、车牌识别等方面均有较好表现。本文采用回归和heatmap两种方式回归关键点,尝试定位红外小目标,形成了一个适合新手学习的基础项目。
1 数据来源
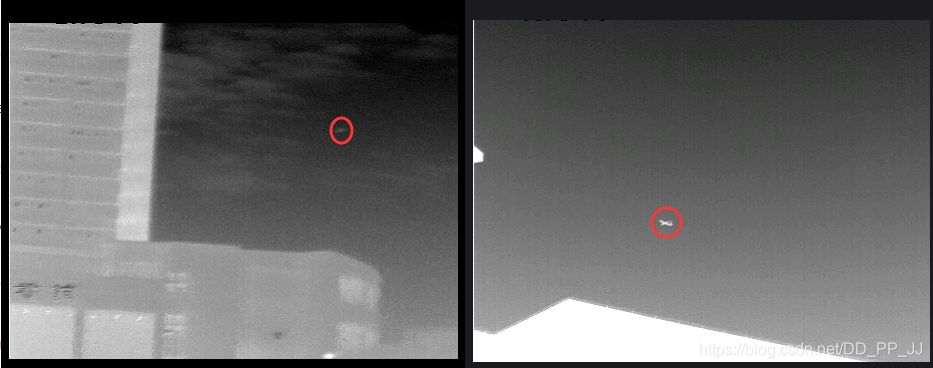
1
0.42 0.596
2 回归确定关键点
2.1 数据加载
- data
- images
- 1.jpg
- 2.jpg
- ...
- labels
- 1.txt
- 2.txt
- ...
class KeyPointDatasets(Dataset):
def __init__(self, root_dir="./data", transforms=None):
super(KeyPointDatasets, self).__init__()
self.img_path = os.path.join(root_dir, "images")
# self.txt_path = os.path.join(root_dir, "labels")
self.img_list = glob.glob(os.path.join(self.img_path, "*.jpg"))
self.txt_list = [item.replace(".jpg", ".txt").replace(
"images", "labels") for item in self.img_list]
if transforms is not None:
self.transforms = transforms
def __getitem__(self, index):
img = self.img_list[index]
txt = self.txt_list[index]
img = cv2.imread(img)
if self.transforms:
img = self.transforms(img)
label = []
with open(txt, "r") as f:
for i, line in enumerate(f):
if i == 0:
# 第一行
num_point = int(line.strip())
else:
x1, y1 = [(t.strip()) for t in line.split()]
# range from 0 to 1
x1, y1 = float(x1), float(y1)
tmp_label = (x1, y1)
label.append(tmp_label)
return img, torch.tensor(label[0])
def __len__(self):
return len(self.img_list)
@staticmethod
def collect_fn(batch):
imgs, labels = zip(*batch)
return torch.stack(imgs, 0), torch.stack(labels, 0)
2.2 网络模型
import torch
import torch.nn as nn
class KeyPointModel(nn.Module):
def __init__(self):
super(KeyPointModel, self).__init__()
self.conv1 = nn.Conv2d(3, 6, 3, 1, 1)
self.bn1 = nn.BatchNorm2d(6)
self.relu1 = nn.ReLU(True)
self.maxpool1 = nn.MaxPool2d((2, 2))
self.conv2 = nn.Conv2d(6, 12, 3, 1, 1)
self.bn2 = nn.BatchNorm2d(12)
self.relu2 = nn.ReLU(True)
self.maxpool2 = nn.MaxPool2d((2, 2))
self.gap = nn.AdaptiveMaxPool2d(1)
self.classifier = nn.Sequential(
nn.Linear(12, 2),
nn.Sigmoid()
)
def forward(self, x):
x = self.conv1(x)
x = self.bn1(x)
x = self.relu1(x)
x = self.maxpool1(x)
x = self.conv2(x)
x = self.bn2(x)
x = self.relu2(x)
x = self.maxpool2(x)
x = self.gap(x)
x = x.view(x.shape[0], -1)
return self.classifier(x)
def train(model, epoch, dataloader, optimizer, criterion):
model.train()
for itr, (image, label) in enumerate(dataloader):
bs = image.shape[0]
output = model(image)
loss = criterion(output, label)
optimizer.zero_grad()
loss.backward()
optimizer.step()
if itr % 4 == 0:
print("epoch:%2d|step:%04d|loss:%.6f" % (epoch, itr, loss.item()/bs))
vis.plot_many_stack({"train_loss": loss.item()*100/bs})
total_epoch = 300
bs = 10
########################################
transforms_all = transforms.Compose([
transforms.ToPILImage(),
transforms.Resize((360,480)),
transforms.ToTensor(),
transforms.Normalize(mean=[0.4372, 0.4372, 0.4373],
std=[0.2479, 0.2475, 0.2485])
])
datasets = KeyPointDatasets(root_dir="./data", transforms=transforms_all)
data_loader = DataLoader(datasets, shuffle=True,
batch_size=bs, collate_fn=datasets.collect_fn)
model = KeyPointModel()
optimizer = torch.optim.Adam(model.parameters(), lr=3e-4)
# criterion = torch.nn.SmoothL1Loss()
criterion = torch.nn.MSELoss()
scheduler = torch.optim.lr_scheduler.StepLR(optimizer,
step_size=30,
gamma=0.1)
for epoch in range(total_epoch):
train(model, epoch, data_loader, optimizer, criterion)
loss = test(model, epoch, data_loader, criterion)
if epoch % 10 == 0:
torch.save(model.state_dict(),
"weights/epoch_%d_%.3f.pt" % (epoch, loss*1000))
2.4 测试结果
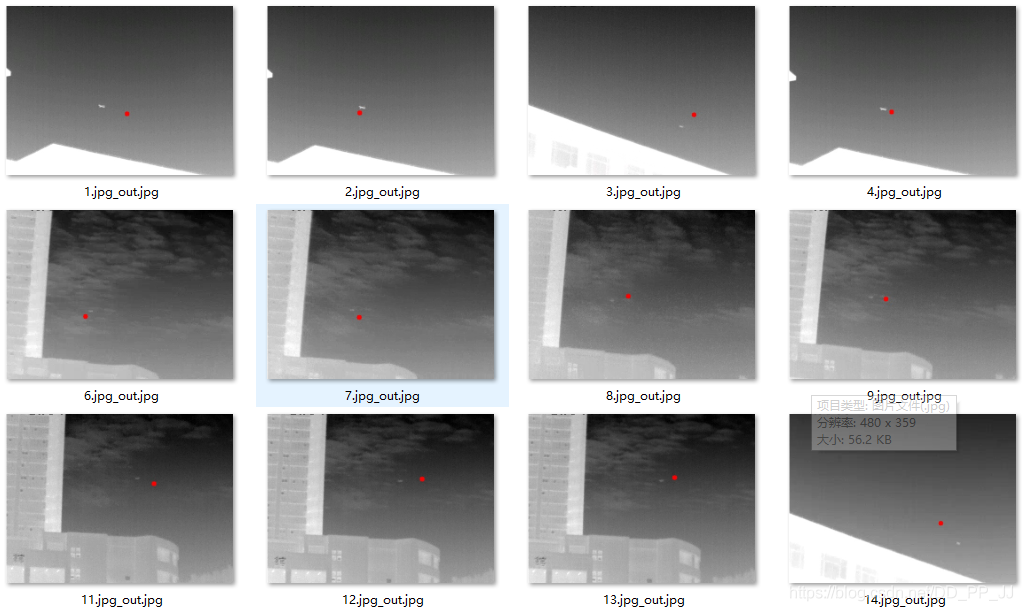
3 heatmap确定关键点
class KeyPointDatasets(Dataset):
def __init__(self, root_dir="./data", transforms=None):
super(KeyPointDatasets, self).__init__()
self.down_ratio = 1
self.img_w = 480 // self.down_ratio
self.img_h = 360 // self.down_ratio
self.img_path = os.path.join(root_dir, "images")
self.img_list = glob.glob(os.path.join(self.img_path, "*.jpg"))
self.txt_list = [item.replace(".jpg", ".txt").replace(
"images", "labels") for item in self.img_list]
if transforms is not None:
self.transforms = transforms
def __getitem__(self, index):
img = self.img_list[index]
txt = self.txt_list[index]
img = cv2.imread(img)
if self.transforms:
img = self.transforms(img)
label = []
with open(txt, "r") as f:
for i, line in enumerate(f):
if i == 0:
# 第一行
num_point = int(line.strip())
else:
x1, y1 = [(t.strip()) for t in line.split()]
# range from 0 to 1
x1, y1 = float(x1), float(y1)
cx, cy = x1 * self.img_w, y1 * self.img_h
heatmap = np.zeros((self.img_h, self.img_w))
draw_umich_gaussian(heatmap, (cx, cy), 30)
return img, torch.tensor(heatmap).unsqueeze(0)
def __len__(self):
return len(self.img_list)
@staticmethod
def collect_fn(batch):
imgs, labels = zip(*batch)
return torch.stack(imgs, 0), torch.stack(labels, 0)
def gaussian2D(shape, sigma=1):
m, n = [(ss - 1.) / 2. for ss in shape]
y, x = np.ogrid[-m:m + 1, -n:n + 1]
h = np.exp(-(x * x + y * y) / (2 * sigma * sigma))
h[h < np.finfo(h.dtype).eps * h.max()] = 0
# 限制最小的值
return h
def draw_umich_gaussian(heatmap, center, radius, k=1):
diameter = 2 * radius + 1
gaussian = gaussian2D((diameter, diameter), sigma=diameter / 6)
# 一个圆对应内切正方形的高斯分布
x, y = int(center[0]), int(center[1])
width, height = heatmap.shape
left, right = min(x, radius), min(width - x, radius + 1)
top, bottom = min(y, radius), min(height - y, radius + 1)
masked_heatmap = heatmap[y - top:y + bottom, x - left:x + right]
masked_gaussian = gaussian[radius - top:radius +
bottom, radius - left:radius + right]
if min(masked_gaussian.shape) > 0 and min(masked_heatmap.shape) > 0: # TODO debug
np.maximum(masked_heatmap, masked_gaussian * k, out=masked_heatmap)
# 将高斯分布覆盖到heatmap上,取最大,而不是叠加
return heatmap
3.2 网络结构
class SematicEmbbedBlock(nn.Module):
def __init__(self, high_in_plane, low_in_plane, out_plane):
super(SematicEmbbedBlock, self).__init__()
self.conv3x3 = nn.Conv2d(high_in_plane, out_plane, 3, 1, 1)
self.upsample = nn.UpsamplingBilinear2d(scale_factor=2)
self.conv1x1 = nn.Conv2d(low_in_plane, out_plane, 1)
def forward(self, high_x, low_x):
high_x = self.upsample(self.conv3x3(high_x))
low_x = self.conv1x1(low_x)
return high_x * low_x
class KeyPointModel(nn.Module):
"""
downsample ratio=2
"""
def __init__(self):
super(KeyPointModel, self).__init__()
self.conv1 = nn.Conv2d(3, 6, 3, 1, 1)
self.bn1 = nn.BatchNorm2d(6)
self.relu1 = nn.ReLU(True)
self.maxpool1 = nn.MaxPool2d((2, 2))
self.conv2 = nn.Conv2d(6, 12, 3, 1, 1)
self.bn2 = nn.BatchNorm2d(12)
self.relu2 = nn.ReLU(True)
self.maxpool2 = nn.MaxPool2d((2, 2))
self.conv3 = nn.Conv2d(12, 20, 3, 1, 1)
self.bn3 = nn.BatchNorm2d(20)
self.relu3 = nn.ReLU(True)
self.maxpool3 = nn.MaxPool2d((2, 2))
self.conv4 = nn.Conv2d(20, 40, 3, 1, 1)
self.bn4 = nn.BatchNorm2d(40)
self.relu4 = nn.ReLU(True)
self.seb1 = SematicEmbbedBlock(40, 20, 20)
self.seb2 = SematicEmbbedBlock(20, 12, 12)
self.seb3 = SematicEmbbedBlock(12, 6, 6)
self.heatmap = nn.Conv2d(6, 1, 1)
def forward(self, x):
x1 = self.conv1(x)
x1 = self.bn1(x1)
x1 = self.relu1(x1)
m1 = self.maxpool1(x1)
x2 = self.conv2(m1)
x2 = self.bn2(x2)
x2 = self.relu2(x2)
m2 = self.maxpool2(x2)
x3 = self.conv3(m2)
x3 = self.bn3(x3)
x3 = self.relu3(x3)
m3 = self.maxpool3(x3)
x4 = self.conv4(m3)
x4 = self.bn4(x4)
x4 = self.relu4(x4)
up1 = self.seb1(x4, x3)
up2 = self.seb2(up1, x2)
up3 = self.seb3(up2, x1)
out = self.heatmap(up3)
return out
datasets = KeyPointDatasets(root_dir="./data", transforms=transforms_all)
data_loader = DataLoader(datasets, shuffle=True,
batch_size=bs, collate_fn=datasets.collect_fn)
model = KeyPointModel()
if torch.cuda.is_available():
model = model.cuda()
optimizer = torch.optim.Adam(model.parameters(), lr=3e-3)
criterion = torch.nn.MSELoss() # compute_loss
scheduler = torch.optim.lr_scheduler.StepLR(optimizer,
step_size=30,
gamma=0.1)
for epoch in range(total_epoch):
train(model, epoch, data_loader, optimizer, criterion, scheduler)
loss = test(model, epoch, data_loader, criterion)
if epoch % 5 == 0:
torch.save(model.state_dict(),
"weights/epoch_%d_%.3f.pt" % (epoch, loss*10000))
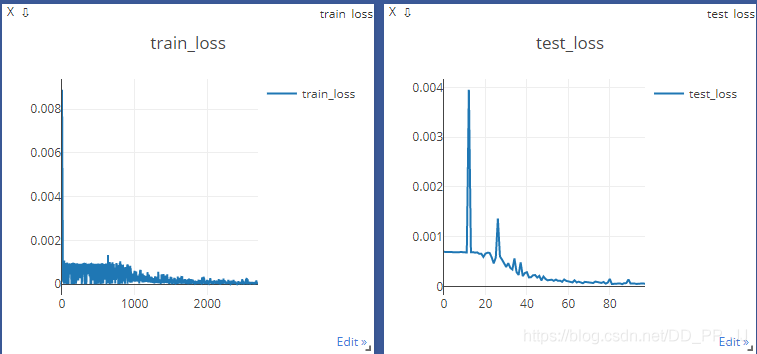
3.4 测试过程
for iter, (image, label) in enumerate(dataloader):
# print(image.shape)
bs = image.shape[0]
hm = model(image)
hm = _nms(hm)
hm = hm.detach().numpy()
for i in range(bs):
hm = hm[i]
hm = np.maximum(hm, 0)
hm = hm/np.max(hm)
hm = normalization(hm)
hm = np.uint8(255 * hm)
hm = hm[0]
# heatmap = torch.sigmoid(heatmap)
# hm = cv2.cvtColor(hm, cv2.COLOR_RGB2BGR)
hm = cv2.applyColorMap(hm, cv2.COLORMAP_JET)
cv2.imwrite("./test_output/output_%d_%d.jpg" % (iter, i), hm)
cv2.waitKey(0)
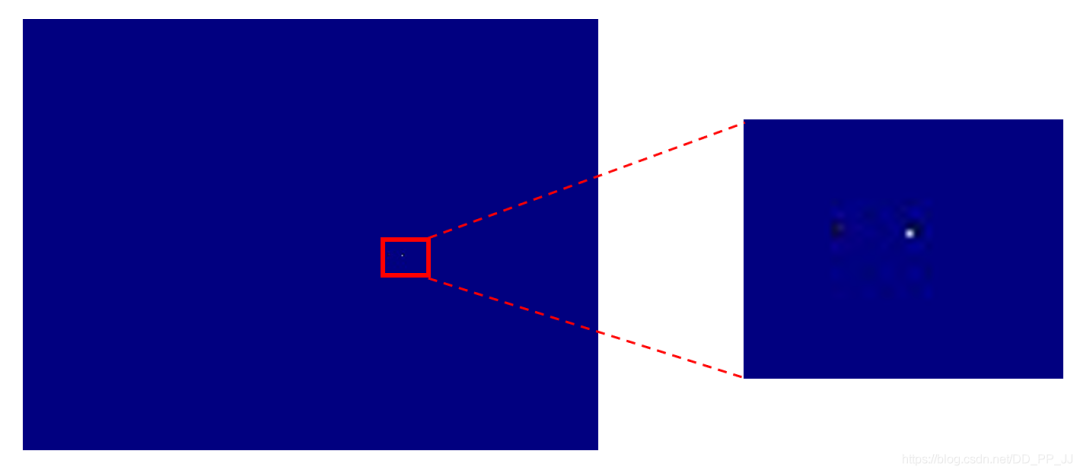
3.5 可视化
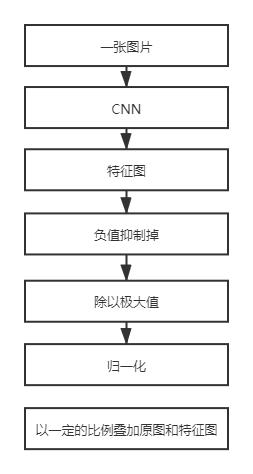
def normalization(data):
_range = np.max(data) - np.min(data)
return (data - np.min(data)) / _range
heatmap = model(img_tensor_list)
heatmap = heatmap.squeeze().cpu()
for i in range(bs):
img_path = img_list[i]
img = cv2.imread(img_path)
img = cv2.resize(img, (480, 360))
single_map = heatmap[i]
hm = single_map.detach().numpy()
hm = np.maximum(hm, 0)
hm = hm/np.max(hm)
hm = normalization(hm)
hm = np.uint8(255 * hm)
hm = cv2.applyColorMap(hm, cv2.COLORMAP_JET)
hm = cv2.resize(hm, (480, 360))
superimposed_img = hm * 0.2 + img
coord_x, coord_y = landmark_coord[i]
cv2.circle(superimposed_img, (int(coord_x), int(coord_y)), 2, (0, 0, 0), thickness=-1)
cv2.imwrite("./output2/%s_out.jpg" % (img_name_list[i]), superimposed_img)
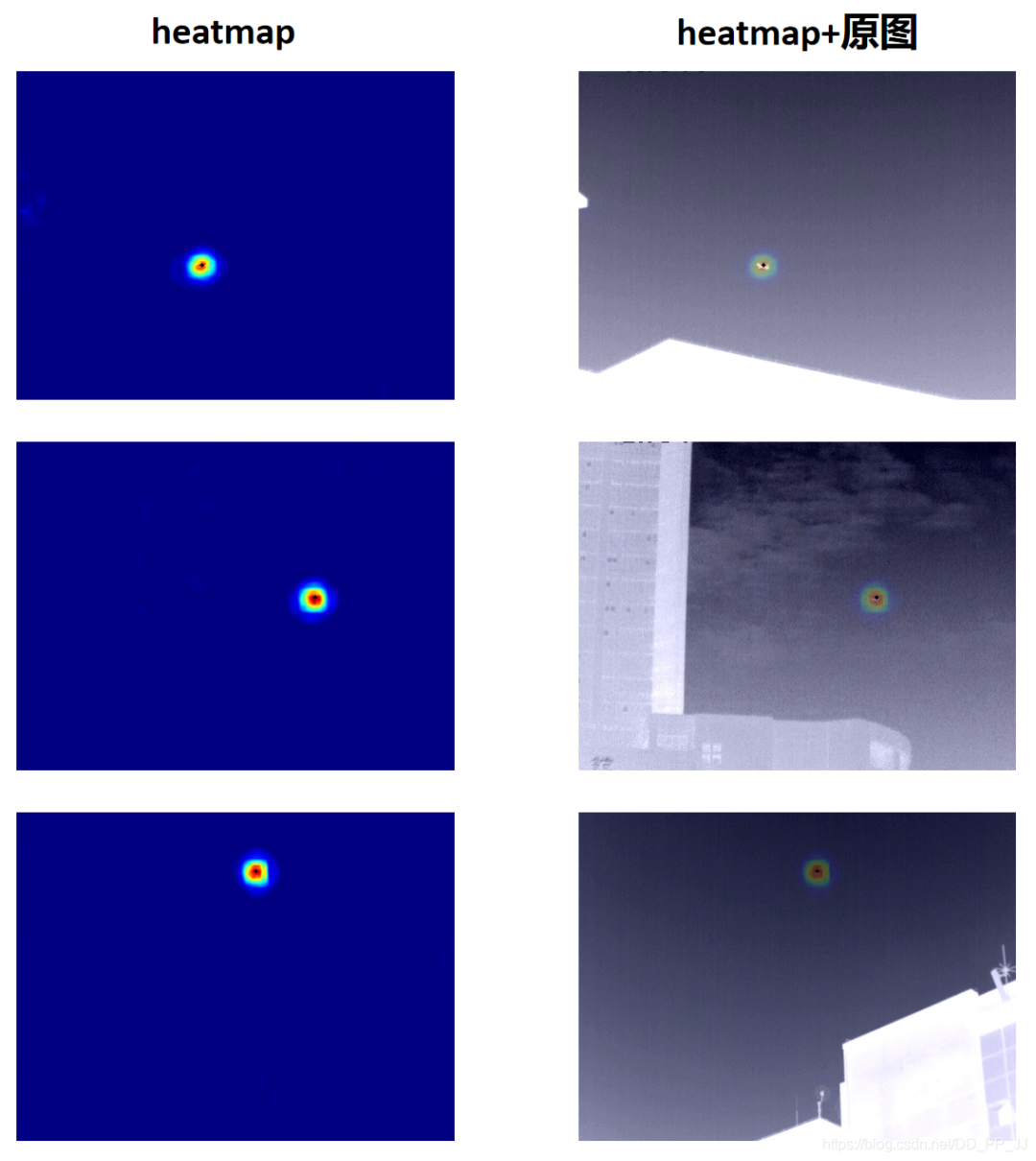
4 总结
推荐阅读
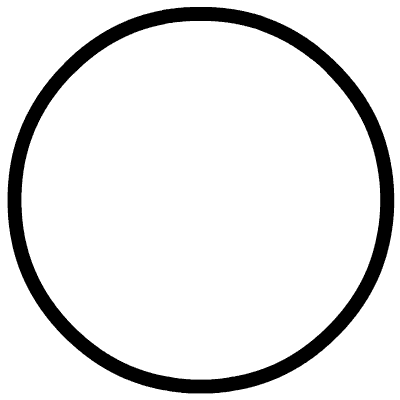
评论