一个小改动,CNN输入固定尺寸图像改为任意尺寸图像
点击上方“小白学视觉”,选择加"星标"或“置顶”
重磅干货,第一时间送达
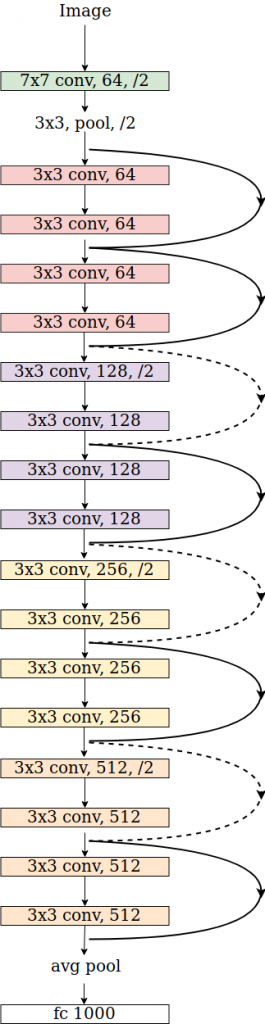
# from the torchvision's implementation of ResNet
class ResNet:
# ...
self.conv1 = nn.Conv2d(3, self.inplanes, kernel_size=7, stride=2, padding=3,
bias=False)
self.bn1 = norm_layer(self.inplanes)
self.relu = nn.ReLU(inplace=True)
self.maxpool = nn.MaxPool2d(kernel_size=3, stride=2, padding=1)
self.layer1 = self._make_layer(block, 64, layers[0])
self.layer2 = self._make_layer(block, 128, layers[1], stride=2, dilate = replace_stride_with_dilation[0])
self.layer3 = self._make_layer(block, 256, layers[2], stride=2, dilate = replace_stride_with_dilation[1])
self.layer4 = self._make_layer(block, 512, layers[3], stride=2, dilate = replace_stride_with_dilation[2])
self.avgpool = nn.AdaptiveAvgPool2d((1, 1))
self.fc = nn.Linear(512 * block.expansion, num_classes)
# ...
def _forward_impl(self, x):
# See note [TorchScript super()]
x = self.conv1(x)
x = self.bn1(x)
x = self.relu(x)
x = self.maxpool(x)
x = self.layer1(x)
x = self.layer2(x)
x = self.layer3(x)
x = self.layer4(x)
x = self.avgpool(x)
x = torch.flatten(x, 1)
x = self.fc(x)
return x
class FullyConvolutionalResnet18(models.ResNet):
def __init__(self, num_classes=1000, pretrained=False, **kwargs):
# Start with standard resnet18 defined here
super().__init__(block = models.resnet.BasicBlock, layers = [2, 2, 2, 2], num_classes = num_classes, **kwargs)
if pretrained:
state_dict = load_state_dict_from_url( models.resnet.model_urls["resnet18"], progress=True)
self.load_state_dict(state_dict)
# Replace AdaptiveAvgPool2d with standard AvgPool2d
self.avgpool = nn.AvgPool2d((7, 7))
# Convert the original fc layer to a convolutional layer.
self.last_conv = torch.nn.Conv2d( in_channels = self.fc.in_features, out_channels = num_classes, kernel_size = 1)
self.last_conv.weight.data.copy_( self.fc.weight.data.view ( *self.fc.weight.data.shape, 1, 1))
self.last_conv.bias.data.copy_ (self.fc.bias.data)
# Reimplementing forward pass.
def _forward_impl(self, x):
# Standard forward for resnet18
x = self.conv1(x)
x = self.bn1(x)
x = self.relu(x)
x = self.maxpool(x)
x = self.layer1(x)
x = self.layer2(x)
x = self.layer3(x)
x = self.layer4(x)
x = self.avgpool(x)
# Notice, there is no forward pass
# through the original fully connected layer.
# Instead, we forward pass through the last conv layer
x = self.last_conv(x)
return x
#1. 导入标准库
import torch
import torch.nn as nn
from torchvision import models
from torch.hub import load_state_dict_from_url
from PIL import Image
import cv2
import numpy as np
from matplotlib import pyplot as plt
#2. 读取ImageNet类ID到名称的映射
if __name__ == "__main__":
# Read ImageNet class id to name mapping
with open('imagenet_classes.txt') as f:
labels = [line.strip() for line in f.readlines()]
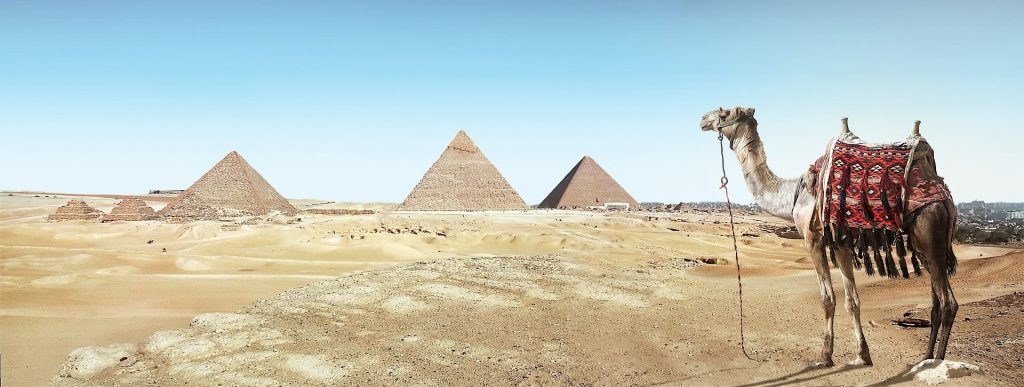
# Read image
original_image = cv2.imread('camel.jpg')
# Convert original image to RGB format
image = cv2.cvtColor(original_image, cv2.COLOR_BGR2RGB)
# Transform input image
# 1. Convert to Tensor
# 2. Subtract mean
# 3. Divide by standard deviation
transform = transforms.Compose([
transforms.ToTensor(), #Convert image to tensor.
transforms.Normalize(
mean=[0.485, 0.456, 0.406], # Subtract mean
std=[0.229, 0.224, 0.225] # Divide by standard deviation
)])
image = transform(image)
image = image.unsqueeze(0)
# Load modified resnet18 model with pretrained ImageNet weights
model = FullyConvolutionalResnet18(pretrained=True).eval()
with torch.no_grad():
# Perform inference.
# Instead of a 1x1000 vector, we will get a
# 1x1000xnxm output ( i.e. a probabibility map
# of size n x m for each 1000 class,
# where n and m depend on the size of the image.)
preds = model(image)
preds = torch.softmax(preds, dim=1)
print('Response map shape : ', preds.shape)
# Find the class with the maximum score in the n x m output map
pred, class_idx = torch.max(preds, dim=1)
print(class_idx)
row_max, row_idx = torch.max(pred, dim=1)
col_max, col_idx = torch.max(row_max, dim=1)
predicted_class = class_idx[0, row_idx[0, col_idx], col_idx]
# Print top predicted class
print('Predicted Class : ', labels[predicted_class], predicted_class)
Response map shape : torch.Size([1, 1000, 3, 8])
tensor([[[977, 977, 977, 977, 977, 978, 354, 437],
[978, 977, 980, 977, 858, 970, 354, 461],
[977, 978, 977, 977, 977, 977, 354, 354]]])
Predicted Class : Arabian camel, dromedary, Camelus dromedarius tensor([354])
# Find the n x m score map for the predicted class
score_map = preds[0, predicted_class, :, :].cpu().numpy()
score_map = score_map[0]
# Resize score map to the original image size
score_map = cv2.resize(score_map, (original_image.shape[1], original_image.shape[0]))
# Binarize score map
_, score_map_for_contours = cv2.threshold(score_map, 0.25, 1, type=cv2.THRESH_BINARY)
score_map_for_contours = score_map_for_contours.astype(np.uint8).copy()
# Find the countour of the binary blob
contours, _ = cv2.findContours(score_map_for_contours, mode=cv2.RETR_EXTERNAL, method=cv2.CHAIN_APPROX_SIMPLE)
# Find bounding box around the object.
rect = cv2.boundingRect(contours[0])
# Apply score map as a mask to original image
score_map = score_map - np.min(score_map[:])
score_map = score_map / np.max(score_map[:])
接下来,我们将响应图与原始图像相乘并显示边界框。
score_map = cv2.cvtColor(score_map, cv2.COLOR_GRAY2BGR)
masked_image = (original_image * score_map).astype(np.uint8)
# Display bounding box
cv2.rectangle(masked_image, rect[:2], (rect[0] + rect[2], rect[1] + rect[3]), (0, 0, 255), 2)
# Display images
cv2.imshow("Original Image", original_image)
cv2.imshow("scaled_score_map", score_map)
cv2.imshow("activations_and_bbox", masked_image)
cv2.waitKey(0)
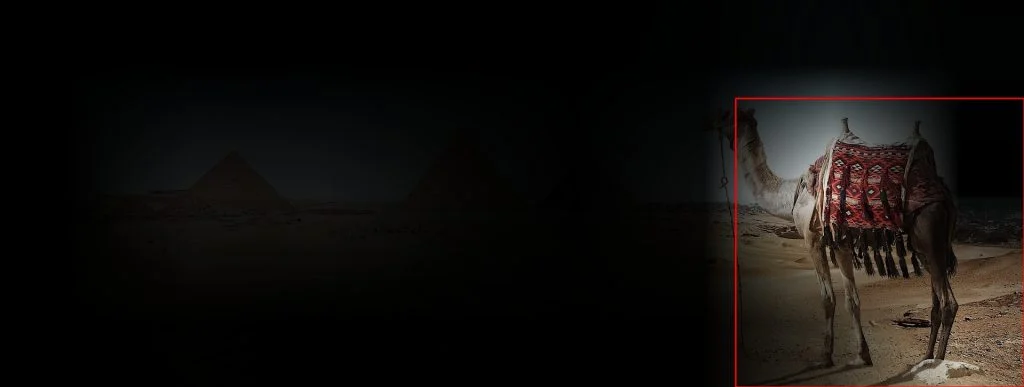
交流群
欢迎加入公众号读者群一起和同行交流,目前有SLAM、三维视觉、传感器、自动驾驶、计算摄影、检测、分割、识别、医学影像、GAN、算法竞赛等微信群(以后会逐渐细分),请扫描下面微信号加群,备注:”昵称+学校/公司+研究方向“,例如:”张三 + 上海交大 + 视觉SLAM“。请按照格式备注,否则不予通过。添加成功后会根据研究方向邀请进入相关微信群。请勿在群内发送广告,否则会请出群,谢谢理解~
评论