CVPR 2021 | “以音动人”:姿态可控的语音驱动说话人脸
点击上方“机器学习与生成对抗网络”,关注星标
获取有趣、好玩的前沿干货!
文章来源 商汤学术
摘要 · 看点
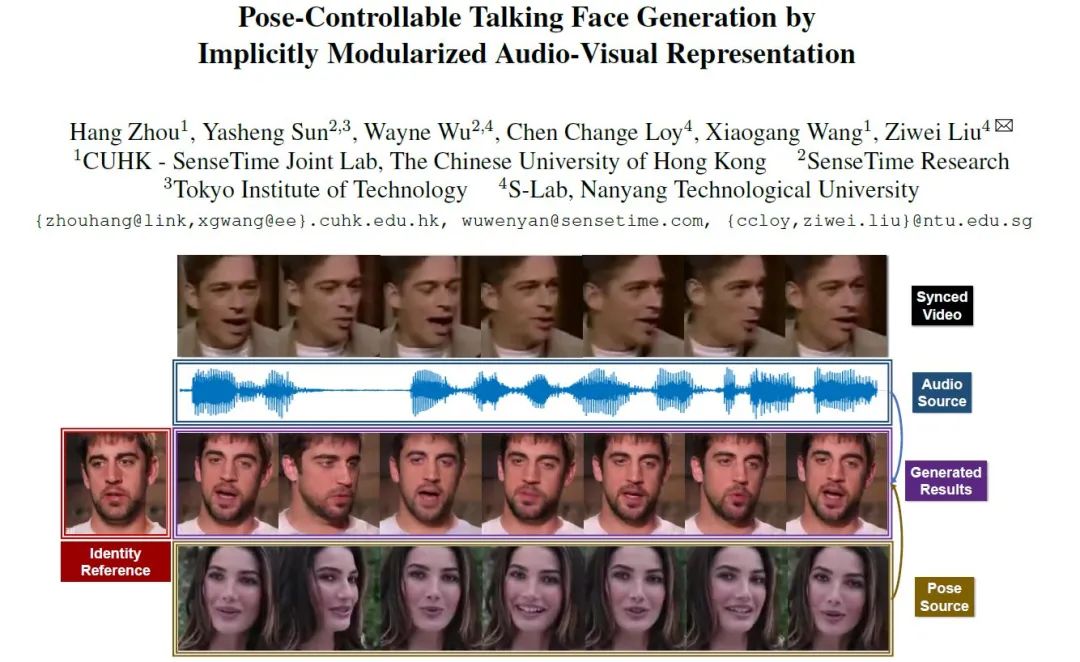
Part 1 任务背景
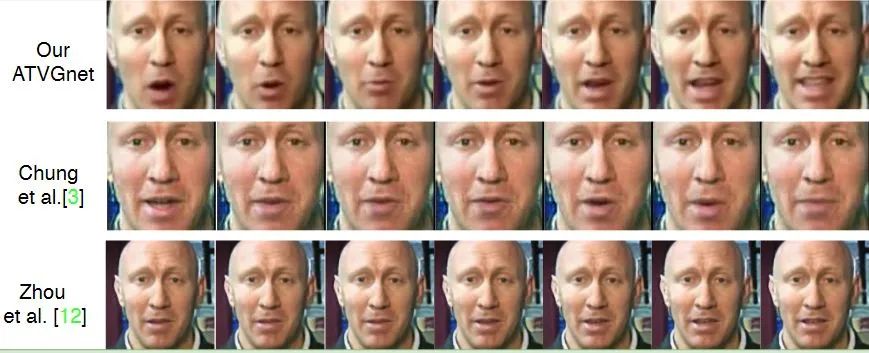
Part 2 方法介绍
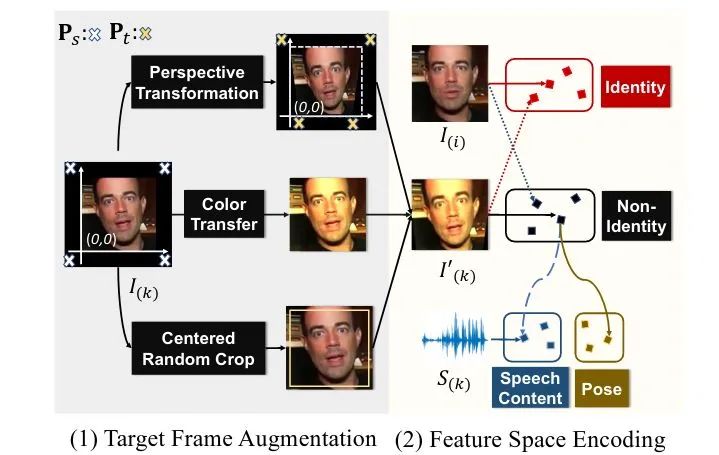






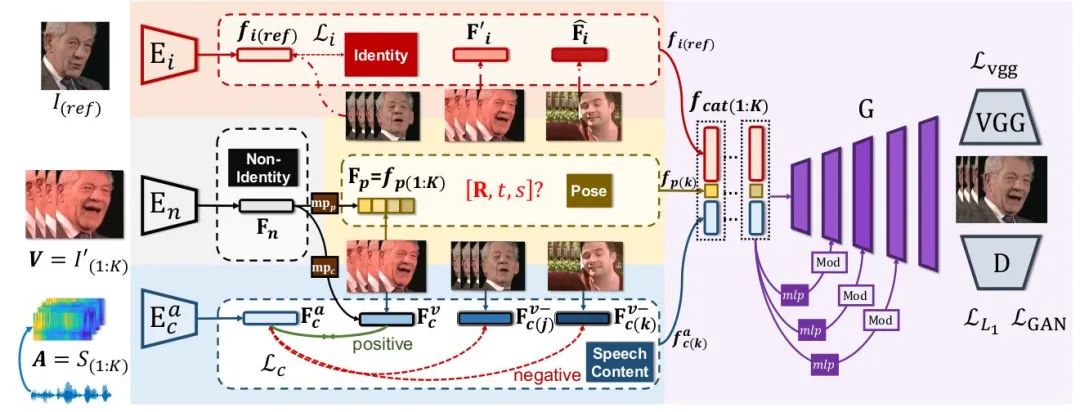


Learning Speech Content Space. 我们希望Non-Identity Space的feature经过一个mapping 映射至speech content space中。而这一latent space的学习,主要依赖音频和视频之间天然的对齐、同步信息(alignment)。在之前的工作中这已经被证明是audio-visual领域用处最广泛的自监督之一[8]。在这里我们使用语音与人脸序列之间的对齐构建contrastive loss进行对齐的约束;对齐的人脸序列和语音特征
是正样本,非对齐的
为负样本。定义两个feature之间的cos距离为
,这一约束可以表达为:

Devising Pose Code. 另一方面,我们借助3D表征中的piror knowledge。一个12维度的向量其实已经足以表达人头的姿态,包括一个9维的旋转矩阵,2维的平移和1维的尺度。所以我们使用一个额外的mapping,从Non-Identity Space中映射一个12维的Pose Code。这个维度上的设计非常重要,如何维度过大,这一latent code所表达的就可能超过pose信息,导致嘴型收到影响。
Part 3 实验结果

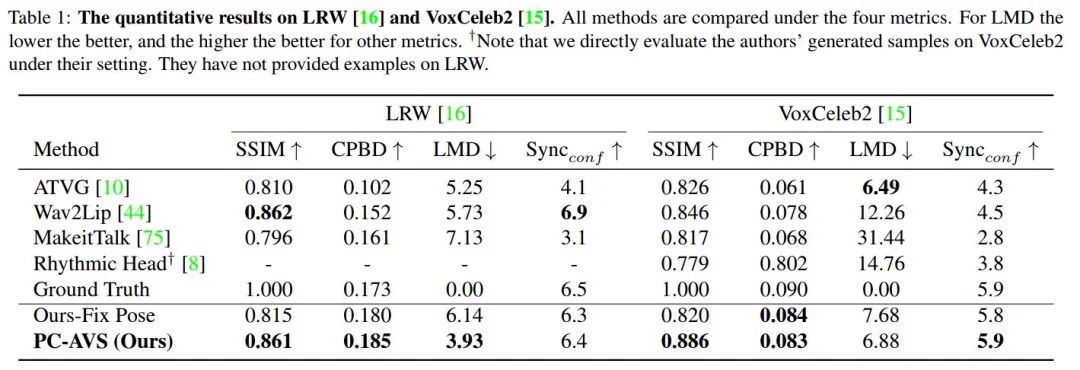
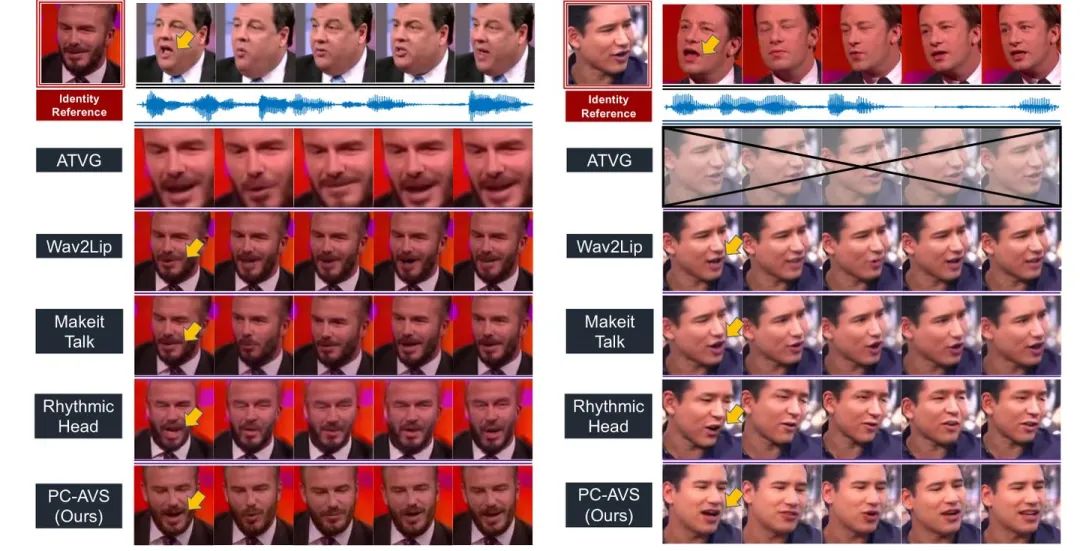
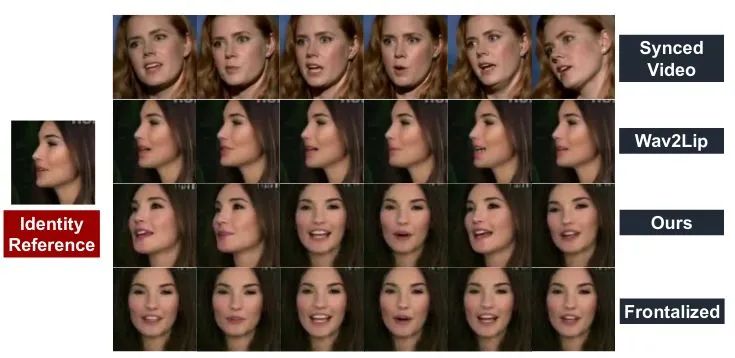
Part 4 总结
在这个工作中,我们提出了Pose-Controllable Audio-Visual System (PC-AVS),成功在语音任意说话人的setting下,生成了姿态可控的结果。综合来看我们的方法有以下几个特质值得关注:
我们的方法不借助预定义的结构信息,仅使用一个图像重建的pipeline,成功定义了一个对人脸pose的表征。 由style-based generator平衡的训练模式让唇形生成收到更契合的重建约束,从而提升了唇形对齐的准确度。 我们实现了任意说话人脸下的自由人头姿态控制,使生成的结果更加真实。 我们的模型在极端情况下有很好的鲁棒性,并且实现了转正的说话人脸生成。
相关链接
Paper 地址:https://arxiv.org/abs/2104.11116
Github:https://github.com/Hangz-nju-cuhk/Talking-Face_PC-AVS
Project Page:https://hangz-nju-cuhk.github.io/projects/PC-AVS
招聘信息
商汤科技-数字人研发团队现正招聘全职AI研究员和工程师,以及研发实习生。我们的研究成果不仅在各大顶级视觉会议上发表,更在大量实际产品中落地。研发方向包括但不限于:图像/视频生成,人脸/人体重建,多模态学习,语音分析等。对我们的研究感兴趣的小伙伴,可以投递简历至xusu@sensetime.com.
References
#What comprises a good talking-head video generation?: A Survey and Benchmark https://arxiv.org/abs/2005.03201
#Joon Son Chung, Amir Jamaludin, and Andrew Zisserman. You said that? In BMVC, 2017. https://arxiv.org/abs/1705.02966
#Hang Zhou, Yu Liu, Ziwei Liu, Ping Luo, and Xiaogang Wang. Talking face generation by adversarially disentangled audio-visual representation. In Proceedings of the AAAI ConConference on Artificial Intelligence (AAAI), 2019. https://arxiv.org/abs/1807.07860
#Lele Chen, Ross K Maddox, Zhiyao Duan, and Chenliang Xu. Hierarchical cross-modal talking face generation with dynamic pixel-wise loss. In Proceedings of the IEEE Conference on Computer Vision and Pattern Recognition (CVPR), 2019. https://www.cs.rochester.edu/u/lchen63/cvpr2019.pdf
#Yang Zhou, Xintong Han, Eli Shechtman, Jose Echevarria, Evangelos Kalogerakis, and Dingzeyu Li. Makeittalk: Speaker-aware talking head animation. SIGGRAPH ASIA, 2020. https://arxiv.org/abs/2004.12992
#Lele Chen, Guofeng Cui, Celong Liu, Zhong Li, Ziyi Kou, Yi Xu, and Chenliang Xu. Talking-head generation with rhythmic head motion. European Conference on Computer Vision (ECCV), 2020. https://www.cs.rochester.edu/u/lchen63/eccv2020-arxiv.pdf
#Egor Burkov, Igor Pasechnik, Artur Grigorev, and Victor Lem-pitsky. Neural head reenactment with latent pose descriptors. In Proceedings of the IEEE Conference on Computer Visionand Pattern Recognition (CVPR), 2020. https://openaccess.thecvf.com/content_CVPR_2020/papers/Burkov_Neural_Head_Reenactment_with_Latent_Pose_Descriptors_CVPR_2020_paper.pdf
#Joon Son Chung and Andrew Zisserman. Out of time: auto-mated lip sync in the wild. In ACCV Workshop, 2016. https://www.robots.ox.ac.uk/~vgg/publications/2016/Chung16a/chung16a.pdf
#Tero Kar
猜您喜欢:
附下载 |《TensorFlow 2.0 深度学习算法实战》
附下载 | 超100篇!CVPR 2020最全GAN论文梳理汇总!
评论