基于Pytorch的动态卷积复现
极市平台
共 5150字,需浏览 11分钟
·
2020-09-17 15:59
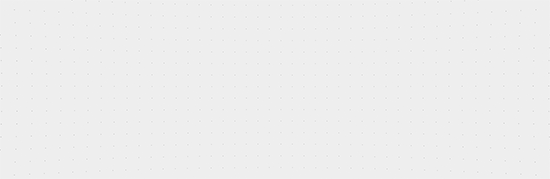
极市导读
本文重点介绍了Pytorch中卷积的实现,并为实现过程中可能出现的问题给出解决方案。文末列出了相关代码,读者可对照代码进行实践。
Dynamic Convolution: Attention over Convolution Kernels
其中包含一维,二维,三维的动态卷积;分别可以用于实现eeg的处理,正常图像的处理,医疗图像中三维脑部的处理等等。




简单回顾
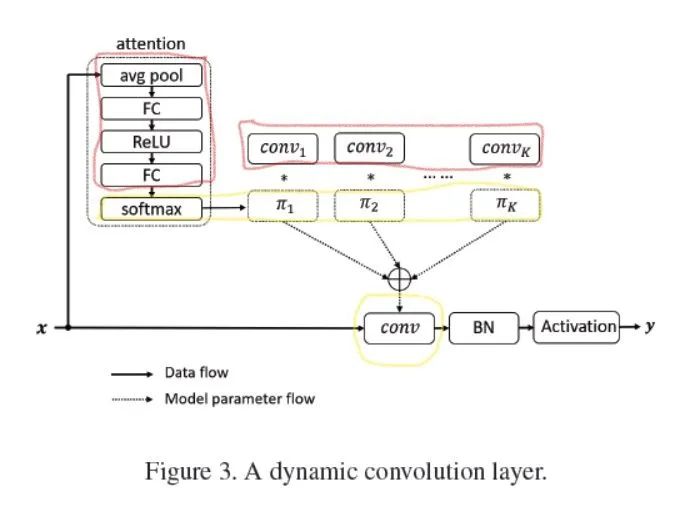








class attention2d(nn.Module):
def __init__(self, in_planes, K,):
super(attention2d, self).__init__()
self.avgpool = nn.AdaptiveAvgPool2d(1)
self.fc1 = nn.Conv2d(in_planes, K, 1,)
self.fc2 = nn.Conv2d(K, K, 1,)
def forward(self, x):
x = self.avgpool(x)
x = self.fc1(x)
x = F.relu(x)
x = self.fc2(x).view(x.size(0), -1)
return F.softmax(x, 1)

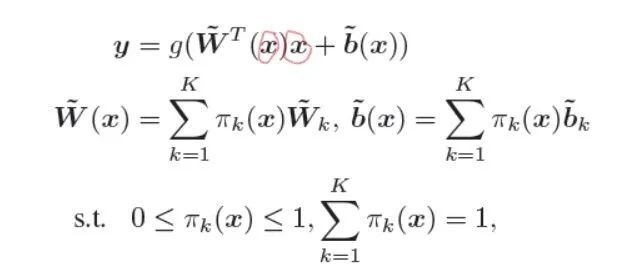




Pytorch卷积的实现














可能会出现的问题

























分组卷积以及如何通过分组卷积实现
大于1的动态卷积

































































class Dynamic_conv2d(nn.Module):
def __init__(self, in_planes, out_planes, kernel_size, stride=1, padding=0, dilation=1, groups=1, bias=True, K=4,):
super(Dynamic_conv2d, self).__init__()
assert in_planes%groups==0
self.in_planes = in_planes
self.out_planes = out_planes
self.kernel_size = kernel_size
self.stride = stride
self.padding = padding
self.dilation = dilation
self.groups = groups
self.bias = bias
self.K = K
self.attention = attention2d(in_planes, K, )
self.weight = nn.Parameter(torch.Tensor(K, out_planes, in_planes//groups, kernel_size, kernel_size), requires_grad=True)
if bias:
self.bias = nn.Parameter(torch.Tensor(K, out_planes))
else:
self.bias = None
def forward(self, x):#将batch视作维度变量,进行组卷积,因为组卷积的权重是不同的,动态卷积的权重也是不同的
softmax_attention = self.attention(x)
batch_size, in_planes, height, width = x.size()
x = x.view(1, -1, height, width)# 变化成一个维度进行组卷积
weight = self.weight.view(self.K, -1)
# 动态卷积的权重的生成, 生成的是batch_size个卷积参数(每个参数不同)
aggregate_weight = torch.mm(softmax_attention, weight).view(-1, self.in_planes, self.kernel_size, self.kernel_size)
if self.bias is not None:
aggregate_bias = torch.mm(softmax_attention, self.bias).view(-1)
output = F.conv2d(x, weight=aggregate_weight, bias=aggregate_bias, stride=self.stride, padding=self.padding,
dilation=self.dilation, groups=self.groups*batch_size)
else:
output = F.conv2d(x, weight=aggregate_weight, bias=None, stride=self.stride, padding=self.padding,
dilation=self.dilation, groups=self.groups * batch_size)
output = output.view(batch_size, self.out_planes, output.size(-2), output.size(-1))
return output
推荐阅读
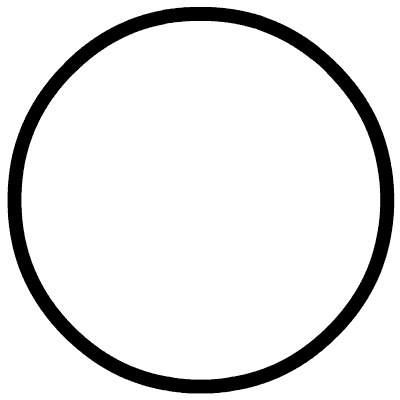
评论