收藏!14 种异常检测方法总结
共 29736字,需浏览 60分钟
·
2023-07-28 07:08
点击上方“小白学视觉”,选择加"星标"或“置顶” 重磅干货,第一时间送达 ![]()
作者丨Ai,来源丨宅码
编辑丨极市平台
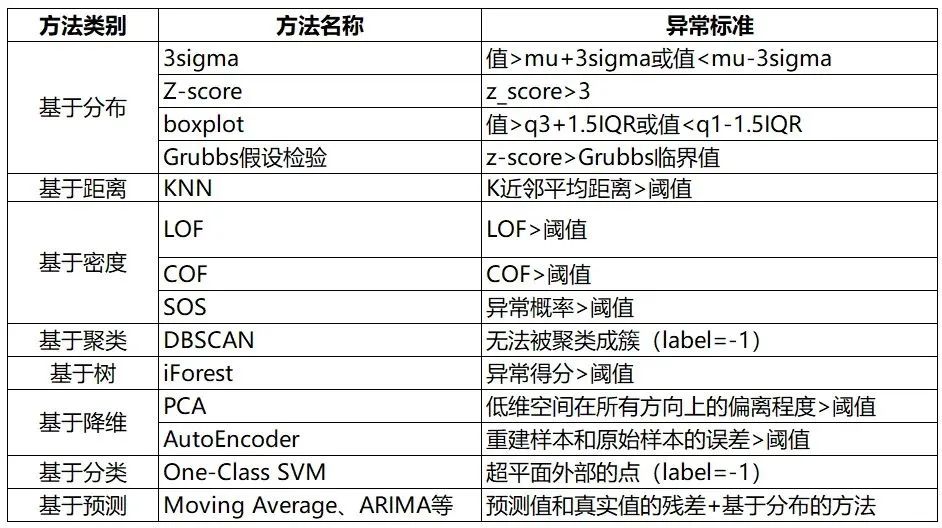
一、基于分布的方法
1. 3sigma
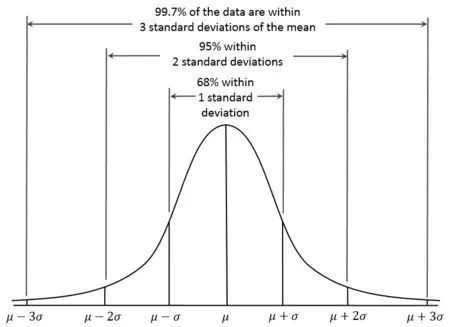
def three_sigma(s):
mu, std = np.mean(s), np.std(s)
lower, upper = mu-3*std, mu+3*std
return lower, upper
2. Z-score
def z_score(s):
z_score = (s - np.mean(s)) / np.std(s)
return z_score
3. boxplot
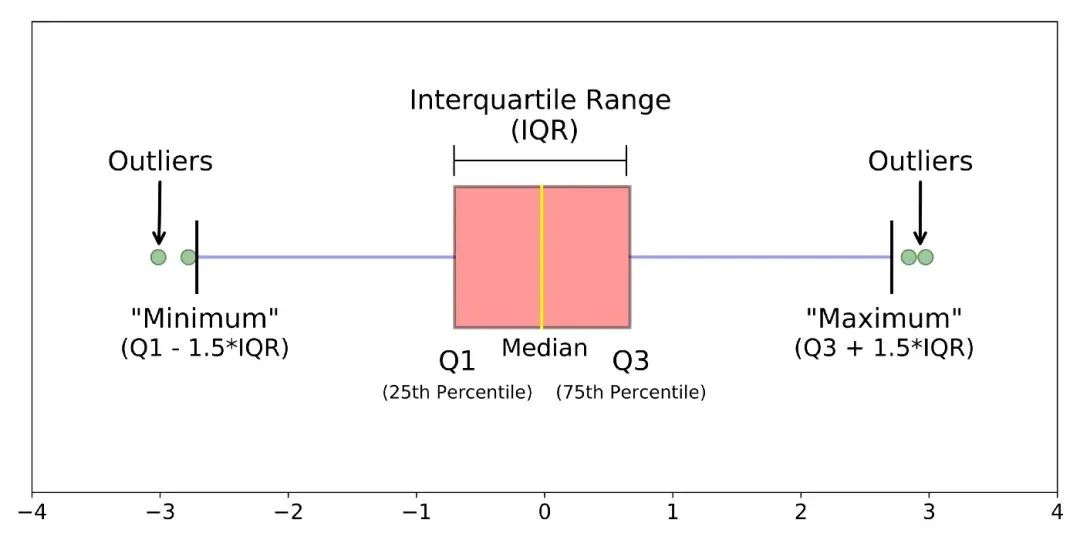
def boxplot(s):
q1, q3 = s.quantile(.25), s.quantile(.75)
iqr = q3 - q1
lower, upper = q1 - 1.5*iqr, q3 + 1.5*iqr
return lower, upper
4. Grubbs假设检验
[1] 时序预测竞赛之异常检测算法综述 - 鱼遇雨欲语与余,知乎:https://zhuanlan.zhihu.com/p/336944097 [2] 剔除异常值栅格计算器_数据分析师所需的统计学:异常检测 - weixin_39974030,CSDN:https://blog.csdn.net/weixin_39974030/article/details/112569610
-
H0: 数据集中没有异常值
-
H1: 数据集中有一个异常值
from outliers import smirnov_grubbs as grubbs
print(grubbs.test([8, 9, 10, 1, 9], alpha=0.05))
print(grubbs.min_test_outliers([8, 9, 10, 1, 9], alpha=0.05))
print(grubbs.max_test_outliers([8, 9, 10, 1, 9], alpha=0.05))
print(grubbs.max_test_indices([8, 9, 10, 50, 9], alpha=0.05))
二、基于距离的方法
1. KNN
[3] 异常检测算法之(KNN)-K Nearest Neighbors - 小伍哥聊风控,知乎:https://zhuanlan.zhihu.com/p/501691799
from pyod.models.knn import KNN
# 初始化检测器clf
clf = KNN( method='mean', n_neighbors=3, )
clf.fit(X_train)
# 返回训练数据上的分类标签 (0: 正常值, 1: 异常值)
y_train_pred = clf.labels_
# 返回训练数据上的异常值 (分值越大越异常)
y_train_scores = clf.decision_scores_
三、基于密度的方法
1. Local Outlier Factor (LOF)
[4] 一文读懂异常检测 LOF 算法(Python代码)- 东哥起飞,知乎:https://zhuanlan.zhihu.com/p/448276009
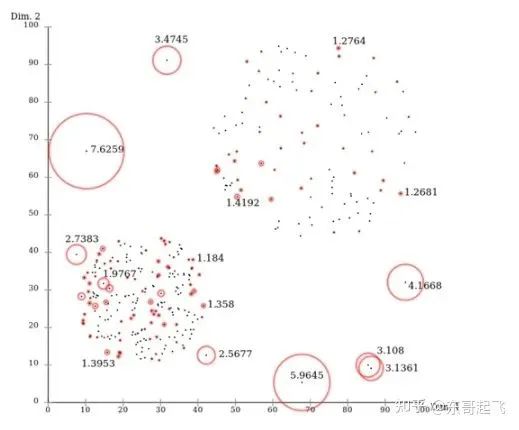
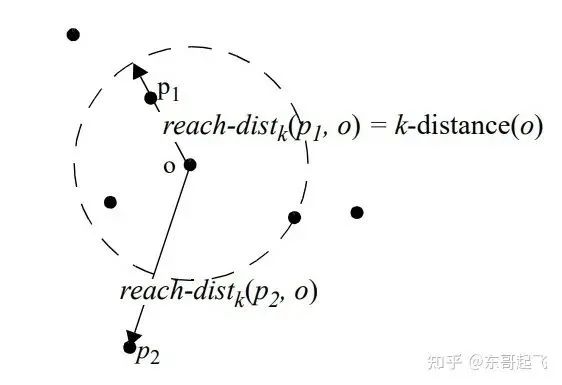
-
对于每个数据点,计算它与其他所有点的距离,并按从近到远排序;
-
对于每个数据点,找到它的K-Nearest-Neighbor,计算LOF得分。
from sklearn.neighbors import LocalOutlierFactor as LOF
X = [[-1.1], [0.2], [100.1], [0.3]]
clf = LOF(n_neighbors=2)
res = clf.fit_predict(X)
print(res)
print(clf.negative_outlier_factor_)
2. Connectivity-Based Outlier Factor (COF)
[5] Nowak-Brzezińska, A., & Horyń, C. (2020). Outliers in rules-the comparision of LOF, COF and KMEANS algorithms. *Procedia Computer Science*, *176*, 1420-1429. [6] 機器學習_學習筆記系列(98):基於連接異常因子分析(Connectivity-Based Outlier Factor) - 劉智皓 (Chih-Hao Liu)
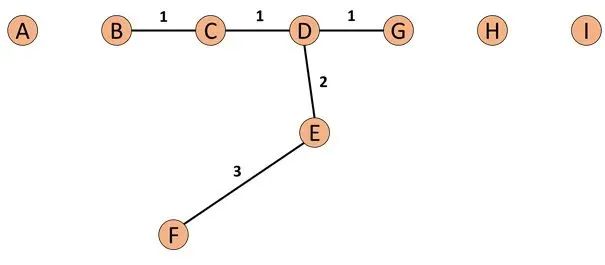
# https://zhuanlan.zhihu.com/p/362358580
from pyod.models.cof import COF
cof = COF(contamination = 0.06, ## 异常值所占的比例
n_neighbors = 20, ## 近邻数量
)
cof_label = cof.fit_predict(iris.values) # 鸢尾花数据
print("检测出的异常值数量为:",np.sum(cof_label == 1))
3. Stochastic Outlier Selection (SOS)
[7] 异常检测之SOS算法 - 呼广跃,知乎:https://zhuanlan.zhihu.com/p/34438518
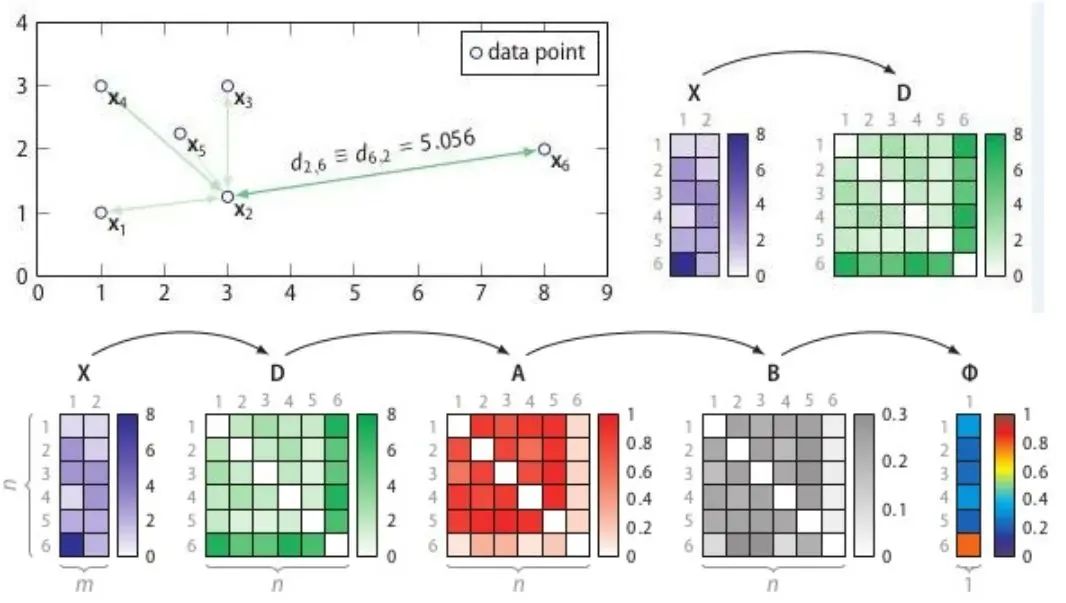
-
计算相异度矩阵D;
-
计算关联度矩阵A;
-
计算关联概率矩阵B;
-
算出异常概率向量。
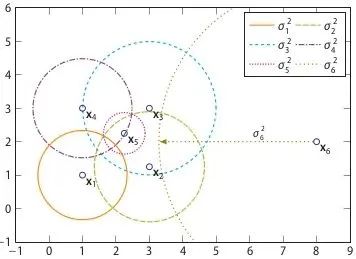
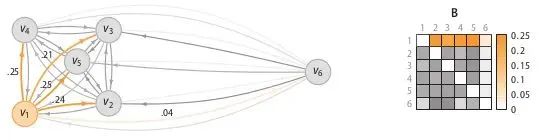
# Ref: https://github.com/jeroenjanssens/scikit-sos
import pandas as pd
from sksos import SOS
iris = pd.read_csv("http://bit.ly/iris-csv")
X = iris.drop("Name", axis=1).values
detector = SOS()
iris["score"] = detector.predict(X)
iris.sort_values("score", ascending=False).head(10)
四、基于聚类的方法
1. DBSCAN
-
输入:数据集,邻域半径Eps,邻域中数据对象数目阈值MinPts;
-
输出:密度联通簇。
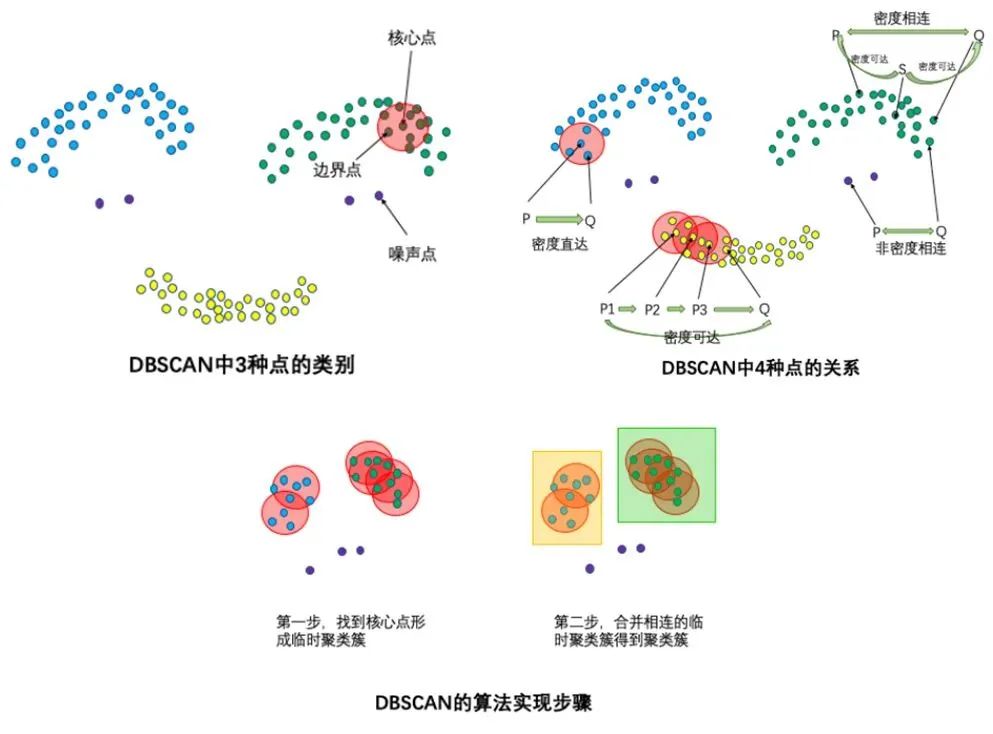
-
从数据集中任意选取一个数据对象点p;
-
如果对于参数Eps和MinPts,所选取的数据对象点p为核心点,则找出所有从p密度可达的数据对象点,形成一个簇;
-
如果选取的数据对象点 p 是边缘点,选取另一个数据对象点;
-
重复以上2、3步,直到所有点被处理。
# Ref: https://zhuanlan.zhihu.com/p/515268801
from sklearn.cluster import DBSCAN
import numpy as np
X = np.array([[1, 2], [2, 2], [2, 3],
[8, 7], [8, 8], [25, 80]])
clustering = DBSCAN(eps=3, min_samples=2).fit(X)
clustering.labels_
array([ 0, 0, 0, 1, 1, -1])
# 0,,0,,0:表示前三个样本被分为了一个群
# 1, 1:中间两个被分为一个群
# -1:最后一个为异常点,不属于任何一个群
五、基于树的方法
1. Isolation Forest (iForest)
[8] 异常检测算法 -- 孤立森林(Isolation Forest)剖析 - 风控大鱼,知乎:https://zhuanlan.zhihu.com/p/74508141 [9] 孤立森林(isolation Forest)-一个通过瞎几把乱分进行异常检测的算法 - 小伍哥聊风控,知乎:https://zhuanlan.zhihu.com/p/484495545 [10] 孤立森林阅读 - Mark_Aussie,博文:https://blog.csdn.net/MarkAustralia/article/details/120181899
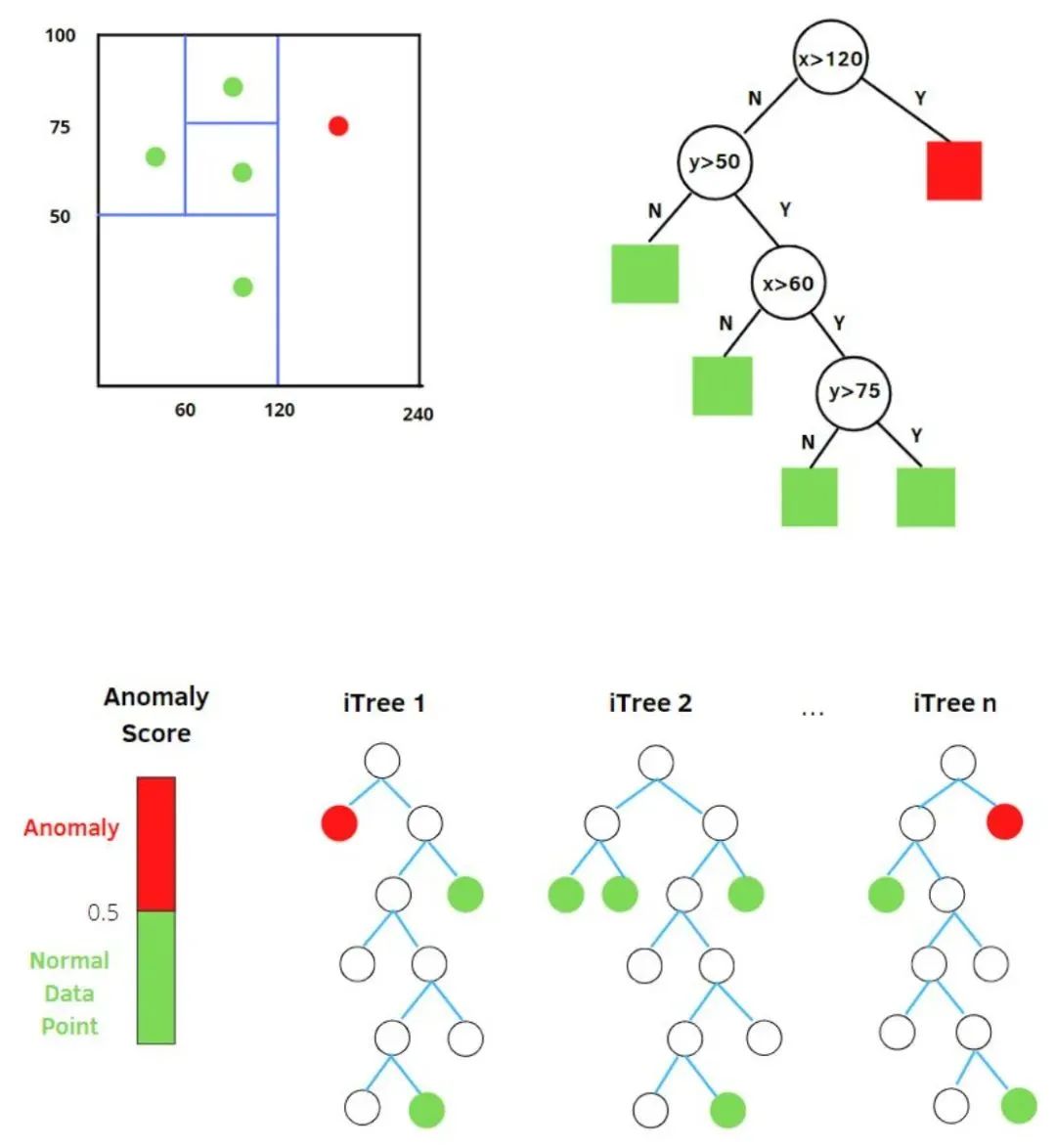
-
h(x):为样本在iTree上的PathLength;
-
E(h(x)):为样本在t棵iTree的PathLength的均值;
-
: 为 个样本构建一个二叉搜索树BST中的末成功搜索平均路径长度 (均值h(x)对外部节点终端的估计等同于BST中的末成功搜索)。 是对样本x的路径长度 进行标准化处理。 是调和数, 可使用 (欧拉常数) 估算。
# Ref:https://zhuanlan.zhihu.com/p/484495545
from sklearn.datasets import load_iris
from sklearn.ensemble import IsolationForest
data = load_iris(as_frame=True)
X,y = data.data,data.target
df = data.frame
# 模型训练
iforest = IsolationForest(n_estimators=100, max_samples='auto',
contamination=0.05, max_features=4,
bootstrap=False, n_jobs=-1, random_state=1)
# fit_predict 函数 训练和预测一起 可以得到模型是否异常的判断,-1为异常,1为正常
df['label'] = iforest.fit_predict(X)
# 预测 decision_function 可以得出 异常评分
df['scores'] = iforest.decision_function(X)
六、基于降维的方法
1. Principal Component Analysis (PCA)
[11] 机器学习-异常检测算法(三):Principal Component Analysis - 刘腾飞,知乎:https://zhuanlan.zhihu.com/p/29091645 [12] Anomaly Detection异常检测--PCA算法的实现 - CC思SS,知乎:https://zhuanlan.zhihu.com/p/48110105
-
特征向量:反应了原始数据方差变化程度的不同方向;
-
特征值:数据在对应方向上的方差大小。
-
考虑在前k个特征向量方向上的偏差:前k个特征向量往往直接对应原始数据里的某几个特征,在前几个特征向量方向上偏差比较大的数据样本,往往就是在原始数据中那几个特征上的极值点。
-
考虑后r个特征向量方向上的偏差:后r个特征向量通常表示某几个原始特征的线性组合,线性组合之后的方差比较小反应了这几个特征之间的某种关系。在后几个特征方向上偏差比较大的数据样本,表示它在原始数据里对应的那几个特征上出现了与预计不太一致的情况。
# Ref: [https://zhuanlan.zhihu.com/p/48110105](https://zhuanlan.zhihu.com/p/48110105)
from sklearn.decomposition import PCA
pca = PCA()
pca.fit(centered_training_data)
transformed_data = pca.transform(training_data)
y = transformed_data
# 计算异常分数
lambdas = pca.singular_values_
M = ((y*y)/lambdas)
# 前k个特征向量和后r个特征向量
q = 5
print "Explained variance by first q terms: ", sum(pca.explained_variance_ratio_[:q])
q_values = list(pca.singular_values_ < .2)
r = q_values.index(True)
# 对每个样本点进行距离求和的计算
major_components = M[:,range(q)]
minor_components = M[:,range(r, len(features))]
major_components = np.sum(major_components, axis=1)
minor_components = np.sum(minor_components, axis=1)
# 人为设定c1、c2阈值
components = pd.DataFrame({'major_components': major_components,
'minor_components': minor_components})
c1 = components.quantile(0.99)['major_components']
c2 = components.quantile(0.99)['minor_components']
# 制作分类器
def classifier(major_components, minor_components):
major = major_components > c1
minor = minor_components > c2
return np.logical_or(major,minor)
results = classifier(major_components=major_components, minor_components=minor_components)
2. AutoEncoder
[13] 利用Autoencoder进行无监督异常检测(Python) - SofaSofa.io,知乎:https://zhuanlan.zhihu.com/p/46188296 [14] 自编码器AutoEncoder解决异常检测问题(手把手写代码) - 数据如琥珀,知乎:https://zhuanlan.zhihu.com/p/260882741
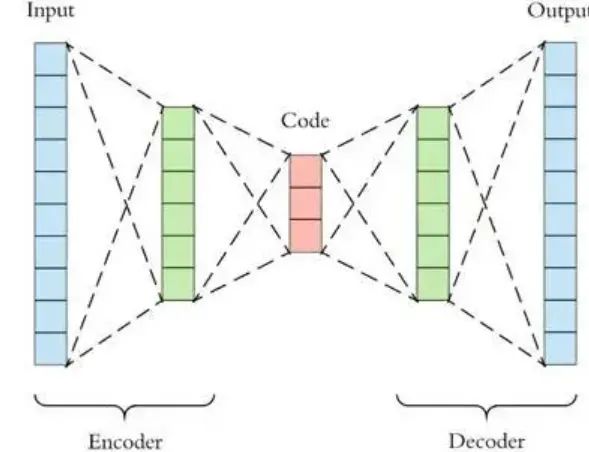
# Ref: [https://zhuanlan.zhihu.com/p/260882741](https://zhuanlan.zhihu.com/p/260882741)
import tensorflow as tf
from keras.models import Sequential
from keras.layers import Dense
# 标准化数据
scaler = preprocessing.MinMaxScaler()
X_train = pd.DataFrame(scaler.fit_transform(dataset_train),
columns=dataset_train.columns,
index=dataset_train.index)
# Random shuffle training data
X_train.sample(frac=1)
X_test = pd.DataFrame(scaler.transform(dataset_test),
columns=dataset_test.columns,
index=dataset_test.index)
tf.random.set_seed(10)
act_func = 'relu'
# Input layer:
model=Sequential()
# First hidden layer, connected to input vector X.
model.add(Dense(10,activation=act_func,
kernel_initializer='glorot_uniform',
kernel_regularizer=regularizers.l2(0.0),
input_shape=(X_train.shape[1],)
)
)
model.add(Dense(2,activation=act_func,
kernel_initializer='glorot_uniform'))
model.add(Dense(10,activation=act_func,
kernel_initializer='glorot_uniform'))
model.add(Dense(X_train.shape[1],
kernel_initializer='glorot_uniform'))
model.compile(loss='mse',optimizer='adam')
print(model.summary())
# Train model for 100 epochs, batch size of 10:
NUM_EPOCHS=100
BATCH_SIZE=10
history=model.fit(np.array(X_train),np.array(X_train),
batch_size=BATCH_SIZE,
epochs=NUM_EPOCHS,
validation_split=0.05,
verbose = 1)
plt.plot(history.history['loss'],
'b',
label='Training loss')
plt.plot(history.history['val_loss'],
'r',
label='Validation loss')
plt.legend(loc='upper right')
plt.xlabel('Epochs')
plt.ylabel('Loss, [mse]')
plt.ylim([0,.1])
plt.show()
# 查看训练集还原的误差分布如何,以便制定正常的误差分布范围
X_pred = model.predict(np.array(X_train))
X_pred = pd.DataFrame(X_pred,
columns=X_train.columns)
X_pred.index = X_train.index
scored = pd.DataFrame(index=X_train.index)
scored['Loss_mae'] = np.mean(np.abs(X_pred-X_train), axis = 1)
plt.figure()
sns.distplot(scored['Loss_mae'],
bins = 10,
kde= True,
color = 'blue')
plt.xlim([0.0,.5])
# 误差阈值比对,找出异常值
X_pred = model.predict(np.array(X_test))
X_pred = pd.DataFrame(X_pred,
columns=X_test.columns)
X_pred.index = X_test.index
threshod = 0.3
scored = pd.DataFrame(index=X_test.index)
scored['Loss_mae'] = np.mean(np.abs(X_pred-X_test), axis = 1)
scored['Threshold'] = threshod
scored['Anomaly'] = scored['Loss_mae'] > scored['Threshold']
scored.head()
七、基于分类的方法
1. One-Class SVM
[15] Python机器学习笔记:One Class SVM - zoukankan,博文:http://t.zoukankan.com/wj-1314-p-10701708.html [16] 单类SVM: SVDD - 张义策,知乎:https://zhuanlan.zhihu.com/p/65617987
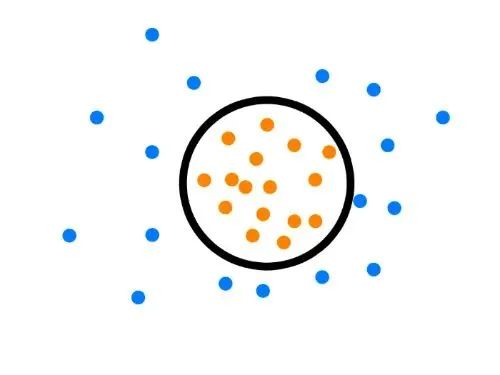
from sklearn import svm
# fit the model
clf = svm.OneClassSVM(nu=0.1, kernel='rbf', gamma=0.1)
clf.fit(X)
y_pred = clf.predict(X)
n_error_outlier = y_pred[y_pred == -1].size
八、基于预测的方法
[17] 【TS技术课堂】时间序列异常检测 - 时序人,文章:https://mp.weixin.qq.com/s/9TimTB_ccPsme2MNPuy6uA
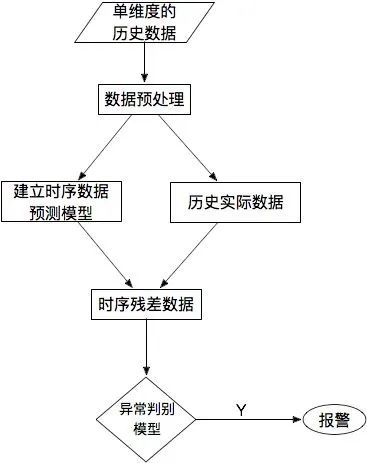
九、总结
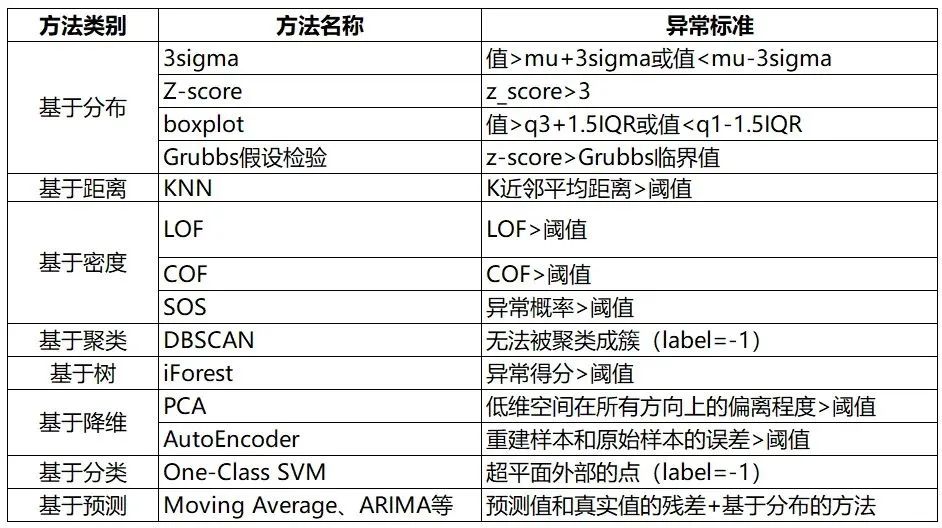
下载1:OpenCV-Contrib扩展模块中文版教程
在「小白学视觉」公众号后台回复:扩展模块中文教程,即可下载全网第一份OpenCV扩展模块教程中文版,涵盖扩展模块安装、SFM算法、立体视觉、目标跟踪、生物视觉、超分辨率处理等二十多章内容。
下载2:Python视觉实战项目52讲 在「小白学视觉」公众号后台回复:Python视觉实战项目,即可下载包括图像分割、口罩检测、车道线检测、车辆计数、添加眼线、车牌识别、字符识别、情绪检测、文本内容提取、面部识别等31个视觉实战项目,助力快速学校计算机视觉。
下载3:OpenCV实战项目20讲 在「小白学视觉」公众号后台回复:OpenCV实战项目20讲,即可下载含有20个基于OpenCV实现20个实战项目,实现OpenCV学习进阶。
交流群
欢迎加入公众号读者群一起和同行交流,目前有SLAM、三维视觉、传感器、自动驾驶、计算摄影、检测、分割、识别、医学影像、GAN、算法竞赛等微信群(以后会逐渐细分),请扫描下面微信号加群,备注:”昵称+学校/公司+研究方向“,例如:”张三 + 上海交大 + 视觉SLAM“。请按照格式备注,否则不予通过。添加成功后会根据研究方向邀请进入相关微信群。请勿在群内发送广告,否则会请出群,谢谢理解~