垃圾邮件识别:用机器学习做中文邮件内容分类
随着微信的迅速发展,工作和生活中的交流也更多依赖于此,但是由于邮件的正式性和规范性,其仍然不可被取代。但是不管是企业内部工作邮箱,还是个人邮箱,总是收到各种各样的垃圾邮件,包括商家的广告、打折促销信息、澳门博彩邮件、理财推广信息等等,不管如何进行垃圾邮件分类,总有漏网之鱼。最重要的是,不同用户对于垃圾邮件的定义并不一致。
而且大部分用户网络安全意识比较一般,万一误点垃圾邮件上钩,或者因为垃圾邮件淹没了工作中的关键信件,则会给个人或者企业造成损失。
垃圾邮件识别一直以来都是痛点难点,虽然方法无非是基于贝叶斯学习或者是概率统计还是深度学习的方法,但是由于业务场景的多样化,垃圾邮件花样实在太多了,所以传统垃圾邮件拦截器总是有点跟不上。
因此打算针对同一数据集,逐步尝试各种方法,来进行垃圾邮件的识别分类——希望假以时日,这种定制化的垃圾邮件识别工具能大幅提升用户的邮箱使用体验。
一、整体思路总的来说,一封邮件可以分为发送人、接收人、抄送人、主题、时间、内容等要素,所以很自然的可以认为主要通过上述要素中的发送方、主题以及内容来进行垃圾邮件判断。
因此我们依次对上述要素进行分析:
垃圾邮件内容分类(通过提取垃圾邮件内容进行判断)
- 中文垃圾邮件分类- 英文垃圾邮件分类 最终,我们可以根据这三个维度进行综合评判,从而实现垃圾邮件的准确分类。本文将根据**邮件内容**进行垃圾邮件分类。
1.数据集介绍
首先我们选择这一个公开的垃圾邮件语料库。该语料库由国际文本检索会议提供,分为英文数据集(trec06p)和中文数据集(trec06c),其中所含的邮件均来源于真实邮件保留了邮件的原有格式和内容。
文件目录形式:delay和full分别是一种垃圾邮件过滤器的过滤机制,full目录下,是理想的邮件分类结果,我们可以视为研究的标签。
trec06c │ └───data │ │ 000 │ │ 001 │ │ ... │ └───215 └───delay │ │ index └───full │ │ index
2.数据加载
2.1 从eml格式中提取邮件要素并且存储成csv
由于目前数据集是存储成邮件的形式,并且通过索引进行垃圾邮件标注,所以我们先提取每一封邮件的发件人、收件人、抄送人、主题、发送时间、内容以及是否垃圾邮件标签。
mailTable=pd.DataFrame(columns=('Sender','Receiver','CarbonCopy','Subject','Date','Body','isSpam'))
# path='trec06p/full/../data/000/004'
# emlContent= emlAnayalyse(path)
# print(emlContent)
f = open('trec06c/full/index', 'r')
csvfile=open('mailChinese.csv','w',newline='',encoding='utf-8')
writer=csv.writer(csvfile)
for line in f:
str_list = line.split(" ")
print(str_list[1])
# 设置垃圾邮件的标签为0
if str_list[0] == 'spam':
label = '0'
# 设置正常邮件标签为1
elif str_list[0] == 'ham':
label = '1'
emlContent= emlAnayalyse('trec06c/full/' + str(str_list[1].split("\n")[0]))
if emlContent is not None:
writer.writerow([emlContent[0],emlContent[1],emlContent[2],emlContent[3],emlContent[4],emlContent[5],label])
其中emlAnayalyze函数利用flanker库中的mime,可以将邮件中的发件人、收件人、抄送人、主题、发送时间、内容等要素提取出来,具体可以参考我的这篇文章,然后存成csv,方便后续邮件分析。
2.2 从csv中提取邮件内容进行分类
def get_data(path):
'''
获取数据
:return: 文本数据,对应的labels
'''
maildf = pd.read_csv(path,header=None, names=['Sender','Receiver','“CarbonCopy','Subject','Date','Body','isSpam'])
filteredmaildf=maildf[maildf['Body'].notnull()]
corpus=filteredmaildf['Body']
labels=filteredmaildf['isSpam']
corpus=list(corpus)
labels=list(labels)
return corpus, labels
通过get_data函数读取csv格式数据,并且提取出内容不为空的数据,和对应的标签。

可以看到一共有40348个数据。
from sklearn.model_selection import train_test_split
# 对数据进行划分
train_corpus, test_corpus, train_labels, test_labels = train_test_split(corpus, labels,
test_size=0.3, random_state=0)
然后通过 sklearn.model_selection库中的train_test_split函数划分训练集、验证集。
# 进行归一化
norm_train_corpus = normalize_corpus(train_corpus)
norm_test_corpus = normalize_corpus(test_corpus)
然后通过normalize_corpus函数对数据进行预处理。
def textParse(text):
listOfTokens=jieba.lcut(text)
newList=[re.sub(r'\W*','',s) for s in listOfTokens]
filtered_text=[tok for tok in newList if len(tok)>0]
return filtered_text
def remove_stopwords(tokens):
filtered_tokens = [token for token in tokens if token not in stopword_list]
filtered_text = ' '.join(filtered_tokens)
return filtered_text
def normalize_corpus(corpus, tokenize=False):
normalized_corpus = []
for text in corpus:
filtered_text = textParse(filtered_text)
filtered_text = remove_stopwords(filtered_text)
normalized_corpus.append(filtered_text)
return normalized_corpus
里面包括textParse、remove_stopwords这两个数据预处理操作。
textParse函数先通过jieba进行分词,然后去除无用字符。
remove_stopwords函数先是加载stop_words.txt停用词表,然后去除停用词。
从而实现数据预处理。
2.3 构建词向量
# 词袋模型特征
bow_vectorizer, bow_train_features = bow_extractor(norm_train_corpus)
bow_test_features = bow_vectorizer.transform(norm_test_corpus)
# tfidf 特征
tfidf_vectorizer, tfidf_train_features = tfidf_extractor(norm_train_corpus)
tfidf_test_features = tfidf_vectorizer.transform(norm_test_corpus)
其中bow_extractor,tfidf_extractor两个函数分别将训练集转化为词袋模型特征和tfidf特征。
from sklearn.feature_extraction.text import CountVectorizer
def bow_extractor(corpus, ngram_range=(1, 1)):
vectorizer = CountVectorizer(min_df=1, ngram_range=ngram_range)
features = vectorizer.fit_transform(corpus)
return vectorizer, features
from sklearn.feature_extraction.text import TfidfTransformer
def tfidf_transformer(bow_matrix):
transformer = TfidfTransformer(norm='l2',
smooth_idf=True,
use_idf=True)
tfidf_matrix = transformer.fit_transform(bow_matrix)
return transformer, tfidf_matrix
from sklearn.feature_extraction.text import TfidfVectorizer
def tfidf_extractor(corpus, ngram_range=(1, 1)):
vectorizer = TfidfVectorizer(min_df=1,
norm='l2',
smooth_idf=True,
use_idf=True,
ngram_range=ngram_range)
features = vectorizer.fit_transform(corpus)
return vectorizer, features
2.4 训练模型以及评估
对如上两种不同的向量表示法,分别训练贝叶斯分类器、逻辑回归分类器、支持向量机分类器,从而验证效果。
from sklearn.naive_bayes import MultinomialNB
from sklearn.linear_model import SGDClassifier
from sklearn.linear_model import LogisticRegression
mnb = MultinomialNB()
svm = SGDClassifier(loss='hinge', n_iter_no_change=100)
lr = LogisticRegression()
# 基于词袋模型的多项朴素贝叶斯
print("基于词袋模型特征的贝叶斯分类器")
mnb_bow_predictions = train_predict_evaluate_model(classifier=mnb,
train_features=bow_train_features,
train_labels=train_labels,
test_features=bow_test_features,
test_labels=test_labels)
# 基于词袋模型特征的逻辑回归
print("基于词袋模型特征的逻辑回归")
lr_bow_predictions = train_predict_evaluate_model(classifier=lr,
train_features=bow_train_features,
train_labels=train_labels,
test_features=bow_test_features,
test_labels=test_labels)
# 基于词袋模型的支持向量机方法
print("基于词袋模型的支持向量机")
svm_bow_predictions = train_predict_evaluate_model(classifier=svm,
train_features=bow_train_features,
train_labels=train_labels,
test_features=bow_test_features,
test_labels=test_labels)
joblib.dump(svm, 'svm_bow.pkl')
# 基于tfidf的多项式朴素贝叶斯模型
print("基于tfidf的贝叶斯模型")
mnb_tfidf_predictions = train_predict_evaluate_model(classifier=mnb,
train_features=tfidf_train_features,
train_labels=train_labels,
test_features=tfidf_test_features,
test_labels=test_labels)
# 基于tfidf的逻辑回归模型
print("基于tfidf的逻辑回归模型")
lr_tfidf_predictions=train_predict_evaluate_model(classifier=lr,
train_features=tfidf_train_features,
train_labels=train_labels,
test_features=tfidf_test_features,
test_labels=test_labels)
# 基于tfidf的支持向量机模型
print("基于tfidf的支持向量机模型")
svm_tfidf_predictions = train_predict_evaluate_model(classifier=svm,
train_features=tfidf_train_features,
train_labels=train_labels,
test_features=tfidf_test_features,
test_labels=test_labels)
输出结果如下所示
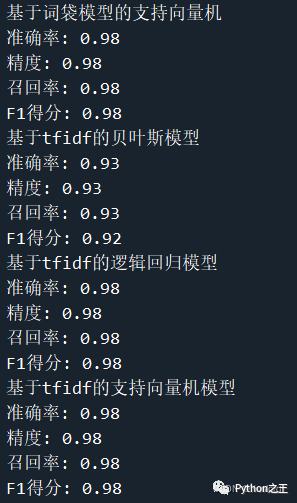
通过针对邮件内容,并且转化为两种不同的词向量进行不同模型的训练,从而得到基于tfidf的支持向量机模型效果最好,可以达到98%的准确率。
原文:https://blog.csdn.net/kobepaul123/article/details/122868530