恕我直言,你们的模型训练都还不够快
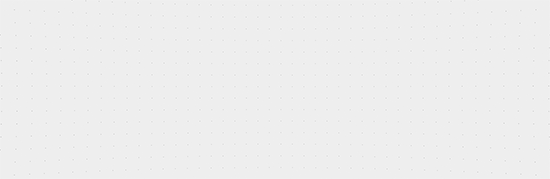
极市导读
作者基于Fairseq和LightSeq分别实现了两个单层的Transformer编码层模型,使pyTorch,Transformer,TensorFlow相关训练的速度加快。>>加入极市CV技术交流群,走在计算机视觉的最前沿
周末在家没事干,也没人约了打游戏,于是打开了gayhub闲逛,哦不,是github。
然后发现了一个挺有意思的项目:
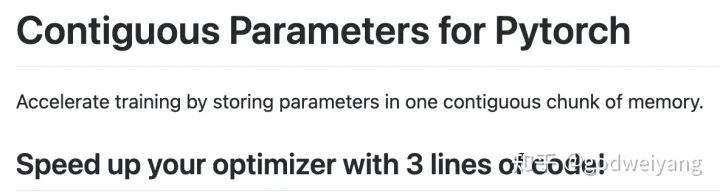
「也就是将你模型中的参数全部存储为一个连续的内存块,加速你的模型训练。」
于是我抱着试试看的心态,基于Fairseq和LightSeq分别实现了两个单层的Transformer编码层模型,简单写了一个例子试了一下。
安装
首先为了运行我这个例子,你需要安装上面提到的contiguous-params
库。然后还需要安装fairseq
和lightseq
库。
pip install contiguous-params fairseq lightseq
一个简单的例子
我这里创建了一个模型,就是单层的Transformer编码层,然后随机输入一个向量,损失函数就是输出向量的所有元素的平方均值。
然后测试了采用参数连续化前后,前向传播、反向传播、梯度更新三部分的时间消耗。
import time
from dataclasses import dataclass
import copy
import torch
from fairseq.modules.transformer_layer import TransformerEncoderLayer
from lightseq.training.ops.pytorch.transformer_encoder_layer import LSTransformerEncoderLayer
from contiguous_params import ContiguousParams
def get_time():
'''CUDA同步并获取当前时间'''
torch.cuda.synchronize(device="cuda:0")
return time.time()
def ls_config_to_fs_args(config):
'''将LightSeq的config转换为Fairseq的args'''
class Args:
encoder_embed_dim: int
encoder_ffn_embed_dim: int
encoder_attention_heads: int
dropout: float
attention_dropout: float
activation_dropout: float
encoder_normalize_before: bool
args = Args(
config.hidden_size,
config.intermediate_size,
config.nhead,
config.hidden_dropout_ratio,
config.attn_prob_dropout_ratio,
config.activation_dropout_ratio,
config.pre_layer_norm
)
return args
def train(model, inputs, masks, contiguous=False):
'''训练过程'''
model.to(device="cuda:0")
model.train()
if contiguous:
parameters = ContiguousParams(model.parameters())
opt = torch.optim.Adam(parameters.contiguous(), lr=1e-3)
else:
opt = torch.optim.Adam(model.parameters(), lr=1e-3)
fw_time, bw_time, step_time = 0, 0, 0
for epoch in range(1000):
opt.zero_grad()
start_time = get_time()
outputs = model(inputs, masks)
loss = torch.square(outputs).mean()
fw_time += get_time() - start_time
start_time = get_time()
loss.backward()
bw_time += get_time() - start_time
start_time = get_time()
opt.step()
step_time += get_time() - start_time
if epoch % 200 == 0:
print("epoch {:>3d}: loss = {:>5.3f}".format(epoch, loss))
return fw_time, bw_time, step_time
if __name__ == "__main__":
# 定义LightSeq的config
config = LSTransformerEncoderLayer.get_config(
max_batch_tokens=4096,
max_seq_len=256,
hidden_size=128,
intermediate_size=512,
nhead=16,
attn_prob_dropout_ratio=0.1,
activation_dropout_ratio=0.1,
hidden_dropout_ratio=0.1,
pre_layer_norm=True,
fp16=False,
local_rank=0
)
# 将LightSeq的config转换为Fairseq的args
args = ls_config_to_fs_args(config)
# 随机生成输入
bsz, sl = 50, 80
inputs = torch.randn(bsz, sl, config.hidden_size).to(device="cuda:0")
masks = torch.zeros(bsz, sl).to(device="cuda:0")
# 定义LightSeq模型并训练
ls_model = LSTransformerEncoderLayer(config)
ls_fw_time, ls_bw_time, ls_step_time = train(ls_model, inputs, masks)
# 定义连续化参数的LightSeq模型并训练
config_cont = copy.deepcopy(config)
ls_model_cont = LSTransformerEncoderLayer(config_cont)
ls_c_fw_time, ls_c_bw_time, ls_c_step_time = train(ls_model_cont, inputs, masks, contiguous=True)
inputs = inputs.transpose(0, 1)
masks = masks > 0.5
# 定义Fairseq模型并训练
fs_model = TransformerEncoderLayer(args)
fs_fw_time, fs_bw_time, fs_step_time = train(fs_model, inputs, masks)
# 定义连续化参数的Fairseq模型并训练
fs_model_cont = TransformerEncoderLayer(args)
fs_c_fw_time, fs_c_bw_time, fs_c_step_time = train(fs_model_cont, inputs, masks, contiguous=True)
print("LightSeq time: {:.3f}s, {:.3f}s, {:.3f}s".format(ls_fw_time, ls_bw_time, ls_step_time))
print("LightSeq (cont) time: {:.3f}s, {:.3f}s, {:.3f}s".format(ls_c_fw_time, ls_c_bw_time, ls_c_step_time))
print("Fairseq time: {:.3f}s, {:.3f}s, {:.3f}s".format(fs_fw_time, fs_bw_time, fs_step_time))
print("Fairseq (cont) time: {:.3f}s, {:.3f}s, {:.3f}s".format(fs_c_fw_time, fs_c_bw_time, fs_c_step_time))
详细讲解
这里最主要的地方就两行:
parameters = ContiguousParams(model.parameters())
opt = torch.optim.Adam(parameters.contiguous(), lr=1e-3)
首先用ContiguousParams
类封装model.parameters()
,然后将封装后的parameters.contiguous()
送进优化器中,这里送进去的就已经是连续存储的一整块参数了。
我们详细阅读ContiguousParams
的源码,可以发现实现很简单:
https://github.com/PhilJd/contiguous_pytorch_params/blob/master/contiguous_params/params.py
核心代码就是下面这个函数,注释中我都详细解释了每一步在干嘛:
def make_params_contiguous(self):
index = 0
# 遍历所有的参数
for p in self._parameters:
# 计算参数p的大小
size = p.numel()
# 在连续参数块中的对应位置赋值参数p
self._param_buffer[index:index + size] = p.data.view(-1)
# 将参数p的数值和梯度都重新指向连续参数块和连续梯度块的对应位置
p.data = self._param_buffer[index:index + size].view(p.data.shape)
p.grad = self._grad_buffer[index:index + size].view(p.data.shape)
# 连续内存块位置偏移到下一个参数
index += size
# 连续参数块的梯度设置为连续梯度块
self._param_buffer.grad = self._grad_buffer
所以在封装了原始参数之后,之后模型计算就会从连续内存块中对应位置取出数值,然后进行计算。
运行结果
我在V100显卡上运行了一下上面的例子,结果如下:
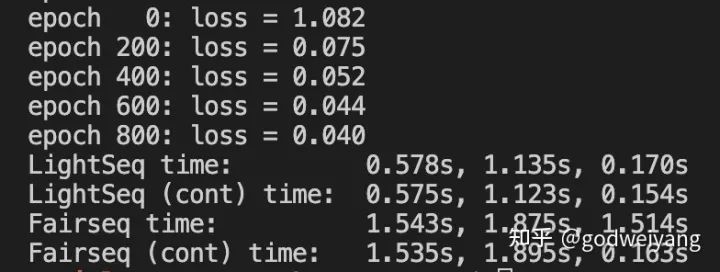
可以看出,LightSeq在采用参数连续化前后,三部分运行时间几乎没有任何变化,这主要是由于LightSeq已经在模型内部做过参数连续化了,因此速度已经很快了。
而Fairseq前后的第三部分,也就是参数更新部分时间缩减非常多,从1.5秒缩短到了0.1秒,总的训练时间几乎缩短了将近一半。
最后对比LightSeq和Fairseq可以明显发现,LightSeq的训练时间比Fairseq快非常多。主要是因为LightSeq采用了算子融合等各种技术,加速了Transformer模型的训练。
总结
所以在你的 「任意」 PyTorch模型中,都可以用上面的参数连续化技术大大加快训练速度。
而如果你的模型是Transformer类模型,那还可以直接用字节跳动开源的LightSeq训练加速引擎,更加方便。
如果你是TensorFlow爱好者,还可以直接用字节跳动开源的NeurST序列生成库进行训练,里面直接集成了LightSeq,所以训练很快。
地址
参数连续化
https://github.com/PhilJd/contiguous_pytorch_params
LightSeq
https://github.com/bytedance/lightseq
NeurST
https://github.com/bytedance/neurst
如果觉得有用,就请分享到朋友圈吧!
公众号后台回复“CVPR21检测”获取CVPR2021目标检测论文下载~

# CV技术社群邀请函 #
备注:姓名-学校/公司-研究方向-城市(如:小极-北大-目标检测-深圳)
即可申请加入极市目标检测/图像分割/工业检测/人脸/医学影像/3D/SLAM/自动驾驶/超分辨率/姿态估计/ReID/GAN/图像增强/OCR/视频理解等技术交流群
每月大咖直播分享、真实项目需求对接、求职内推、算法竞赛、干货资讯汇总、与 10000+来自港科大、北大、清华、中科院、CMU、腾讯、百度等名校名企视觉开发者互动交流~
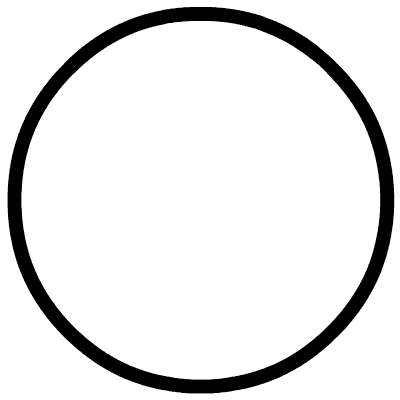