大数据告诉你,你为什么会跳槽?
共 8603字,需浏览 18分钟
· 2021-04-13
本文分析数据科学家求职情况数据集,运用常见EDA方法分析每个特征情况及他们与目标变量之间的关系。使用seaborn
进行数据可视化辅助分析数据科学家们更换工作都有哪些特征。
若你需要了解本数据集中每个特征变量情况,可参见《数据科学家们更换工作都有哪些特征上》。本篇将继续探索分析,看看跳槽的人群都有哪些特征。
你打算换工作吗?
0 - Not looking for job change
1 - Looking for a job change
target = df_train['target']
percent_nan(target)
Total | % | |
---|---|---|
0.0 | 14381 | 75.1 |
1.0 | 4777 | 24.9 |
sns.countplot(x='target',
data=df_train,
palette="Set1").set_title('Train')
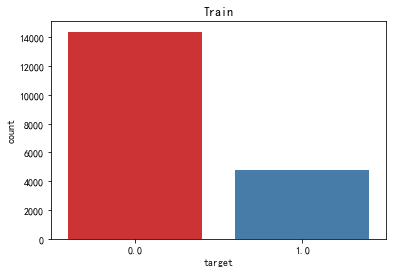
从求职人数角度看,求职人数明显低于未求职人数,仅占总人数的1/4。你是否打算跳槽?可见从事数据分析工作的工作者还算是比较稳定的职业。从正负样本数量看,此数据集为不平衡数据集。对于样本不平衡,我们有较多处理方法,不在本文范围内,下篇将为介绍不平衡数据集的常规处理方法。
人群画像--求职者们都有哪些特征
大城市更能留住人才
with sns.axes_style():
g = sns.displot(data=df_train,
x='city_development_index',
hue='target',
kind='kde',
legend=True,
height=6,
aspect=2)
g.set_ylabels(fontsize=15)
g.set_xlabels(fontsize=15)
g.set_xticklabels(fontsize=15)
g.set_yticklabels(fontsize=15)
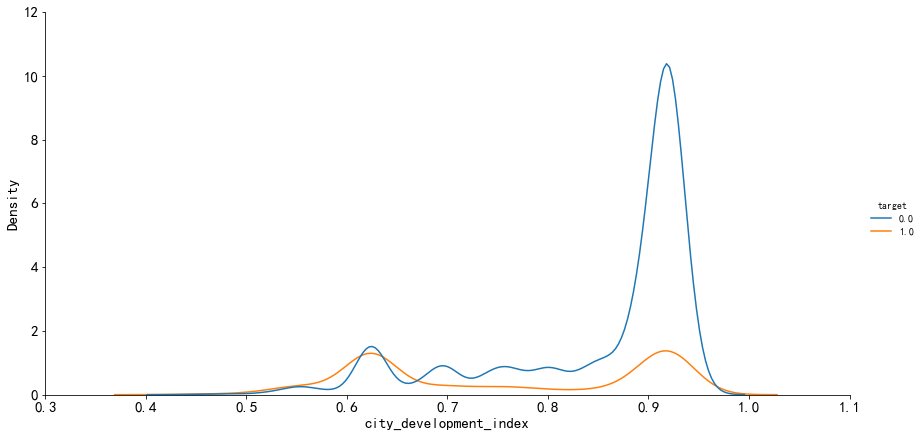
plt.figure(figsize=(10,6))
sns.boxplot(x="target",y="city_development_index",data=df_train,palette="Set2")
plt.title("Distribution of city development index",fontsize=15)
plt.xlabel("looking for job change",fontsize=15)
plt.ylabel("city_development_index",fontsize=15)
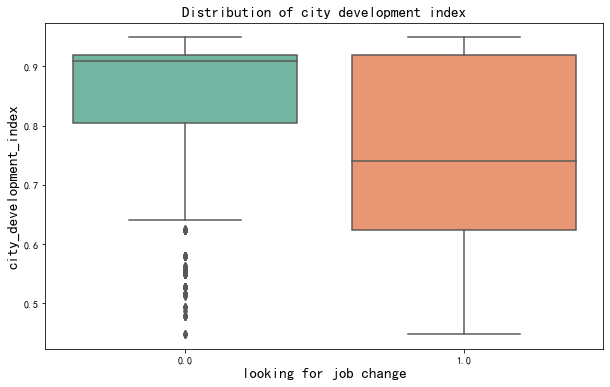
平均城市发展指数越高的城市,更换工作的人越少。也就是说大城市的人更加倾向越扎根稳定工作。在大城市中可以得到更好的发展机会,得到更高的薪酬,人们更加愿意长久地服务一家企业。
相比之下,城市发展指数较低的城市并不能够很好地留住人才。
男性女性是否有差异?
def draw_countplot(hue,palette="Set1"):
plt.figure(figsize=(10,6))
ax = sns.countplot(x="target",data=df_train, hue=hue,palette=palette)
total =float(len(df_train))
plt.title("looking for Job change or not ?",fontsize=15)
plt.xlabel("looking for job change",fontsize=15)
plt.ylabel("Count",fontsize=15)
for p in ax.patches:
percentage = '{:.1f}%'.format(100 * p.get_height()/total)
x = p.get_x() + p.get_width()
y = p.get_height() + 50
ax.annotate(percentage, (x, y),ha='right',fontsize=15)
plt.show()
draw_countplot(hue="gender")
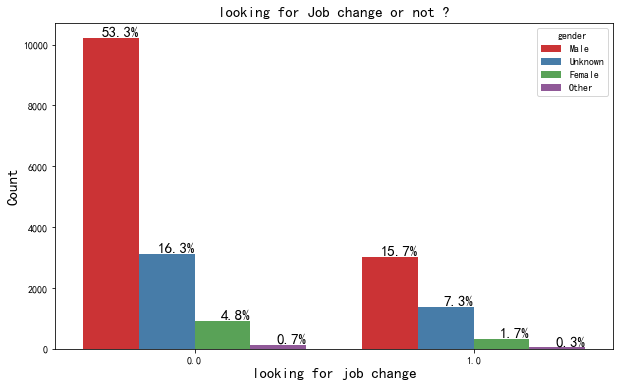
从事数据科学工作的男性居多,而女性仅占有不到10个点。 没有跳槽需求的男女比例约11%,比正在寻求工作的男女比例9.2%高出约两个百分点,或许从事数据科学工作的男性更加倾向稳定,又获取更换工作的成本更高,你是属于哪类呢? 目前约有80%的人不希望换工作。
具有相关经验的人更加倾向稳定工作
draw_countplot("relevent_experience","Set2")
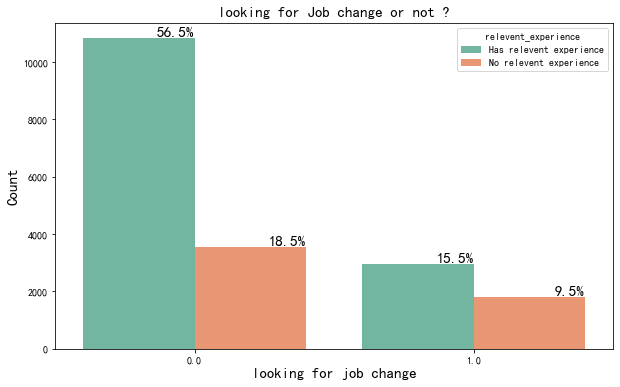
在求职者中,有相关经验和没有相关经验的人相差不大。而稳定就业人群中,大部分人都是有一定的相关经验,且较没有相关经验的人明显较多。
由此也可以看出,有相关经验的人更能够更好驾驭当前工作,更不易更换工作。
没有相关经验的人群,一般为刚毕业的应届生,而应届生离职率高达20%-30%,如此高的离职率追根究底是什么原因,是薪酬待遇不到位?是发展机会不够多?是工作强度过高?是与同事或老板相处不融洽?这是个值得深思的问题。
继续教育是离职主要原因吗?
plt.figure(figsize=(10,6))
ax = sns.countplot(x="enrolled_university",data=df_train[df_train['target']==1],palette="Set3")
total_1 =float(len(df_train[df_train['target']==1]))
plt.title("University enrollment status of persons looking for job change",fontsize=15)
plt.xlabel("University enrollment status",fontsize=15)
plt.ylabel("count",fontsize=12)
for p in ax.patches:
percentage = '{:.1f}%'.format(100 * p.get_height()/total_1)
x = p.get_x() + p.get_width()
y = p.get_height()+ 50
ax.annotate(percentage, (x, y),ha='right',fontsize=15)
ax.set_xticklabels(df_train['enrolled_university'].unique(),fontsize=15)
ax.set_ylim(top=3300)
plt.show()
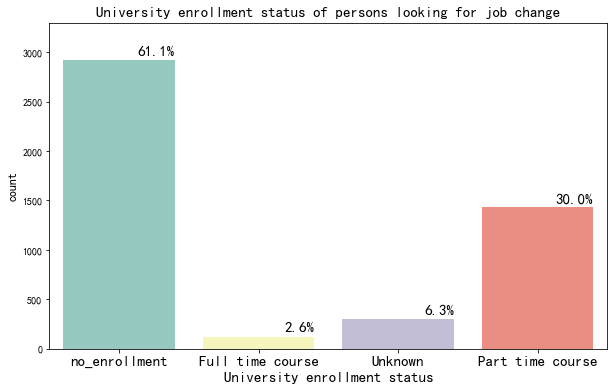
大约37%的人离职是由于离职后继续高等教育。 大约61%的人并没有没有登记需要任何继续教育。 所以,他们离职可能是由于其他原因,比如薪水问题,工作满意度等等。
跳槽的人群中的学历情况是怎样的?
g = sns.catplot(x="target",
hue='education_level',
data=df_train,
palette="Set1",
kind="count",
height=8,
aspect=1.5)
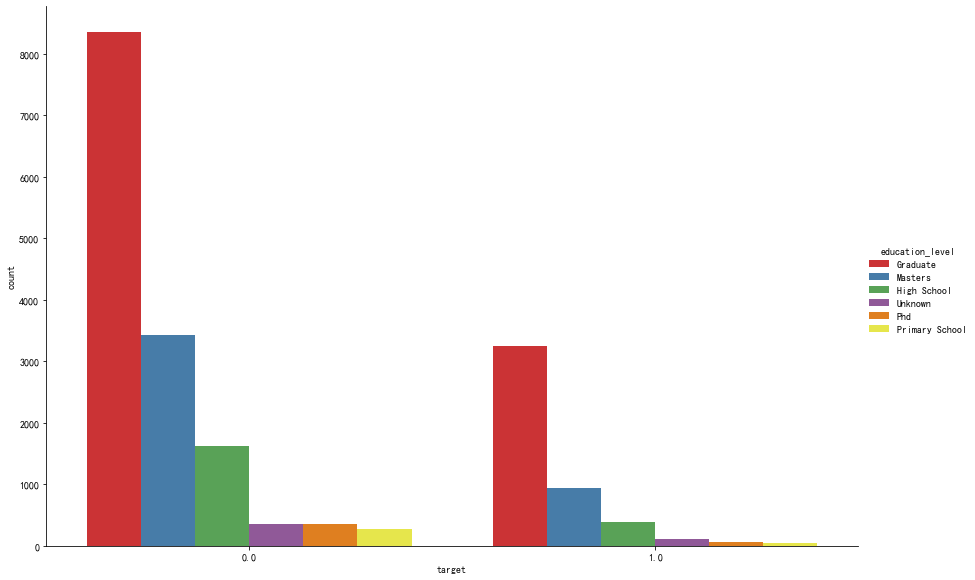
从事数据科学工作的人群中,大学本科毕业生居多,高达60.5%,而硕士研究生人数也较高,占比22.8%.
而大学本科学历人群比具有硕士学历的人群更易跳槽。学历较高,意味着有相对较高的机会拿到更高的待遇,对工作满意度更高,更易受公司重视。当然,这都不是绝对的。
在本单位服务一年的人更易跳槽
plt.figure(figsize=(10,6))
ax = sns.countplot(x="last_new_job",data=df_train[df_train['target']==1])
total_1 =float(len(df_train[df_train['target']==1]))
plt.title("Difference in years between current job and previous job",fontsize=15)
plt.xlabel("Difference in years",fontsize=15)
plt.ylabel("count",fontsize=15)
for p in ax.patches:
percentage = '{:.1f}%'.format(100 * p.get_height()/total_1)
x = p.get_x() + p.get_width()
y = p.get_height()+50
ax.annotate(percentage, (x, y),ha='right',fontsize=15)
ax.set_ylim(top=2300)
plt.show()
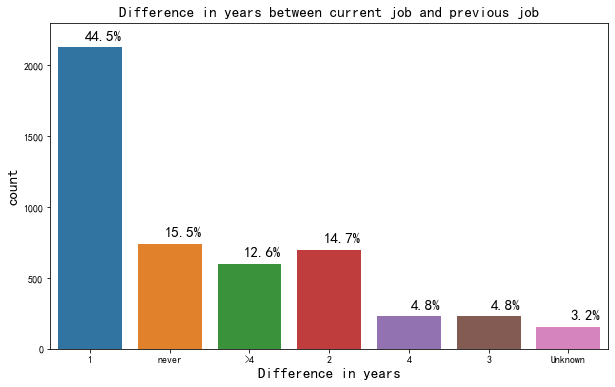
与上一份工作相差一年的人占比高达44.5%,即此工作不到一年的人更易寻求更换工作。一年基本成为一个时限,大部分人在一个单位工作能坚持一年,其稳定性将会更好。
理工科人群更易选择跳槽
major_discipline = df_train[df_train['target'] == 1]['major_discipline']
values = major_discipline.value_counts()
labels = values.keys()
fig,ax = plt.subplots(figsize=(8,8))
plt.pie(x = values, labels = labels , autopct="%.2f%%",pctdistance=0.8,explode =[0.1,0,0,0,0,0,0])
plt.title('looking for job change (major discipline wise)', fontsize=18)
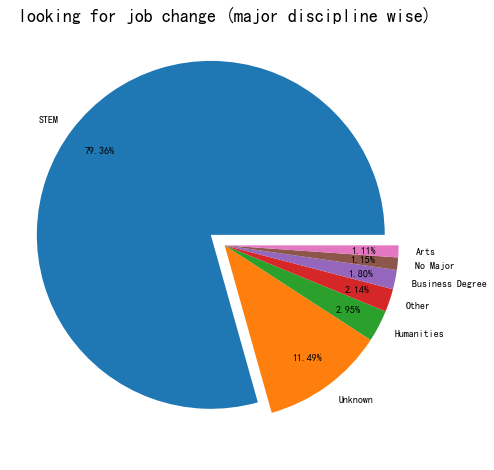
跳槽人群中,理工科占比约80%。作为一个理工科,更加倾向技术含量较高的工作,但大部分岗位并不能满足他们但需求。再加上理工科的岗位较其他学科的岗位更多,选择面更加广泛,这也助长了很多从业者跳槽的意愿。
毕业后工作多少年是跳槽高峰期?
order_experience = df_train[df_train['target']==1].experience.value_counts().index
plt.figure(figsize=(10,6))
ax = sns.countplot(x="experience",data=df_train[df_train['target']==1], order=order_experience)
total_1 =float(len(df_train[df_train['target']==1]))
plt.title("Work experience")
plt.xlabel("Work experience")
for p in ax.patches:
percentage = '{:.1f}%'.format(100 * p.get_height()/total_1)
x = p.get_x() + p.get_width()
y = p.get_height()
ax.annotate(percentage, (x, y),ha='center')
plt.show()
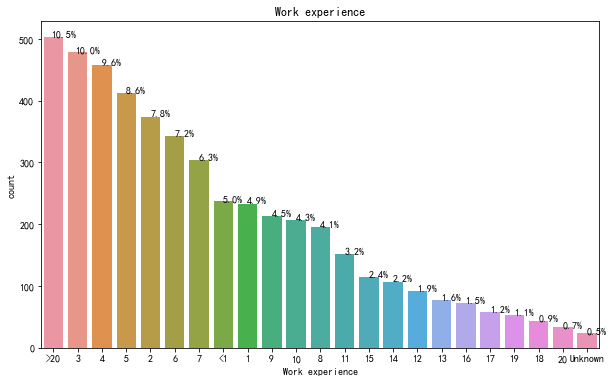
有3、4、5、2和>20经验的人更有可能寻求换工作。工作大于20年基本面临退休。
跳槽高峰期在工作3-5年,在大部分招聘岗位的招聘需求也是在这个年限。除了市场需求大外,另一个重要原因是,此年限内的上班族在职场里,无论是专业知识、工作激情、还是经验阅历相比于近年限段的人群,都是属于高峰期。
培训时长与目标变量
sns.displot(data=df_train,
x='training_hours',
hue='target',
kind='kde',
height=6,
aspect=1.5)
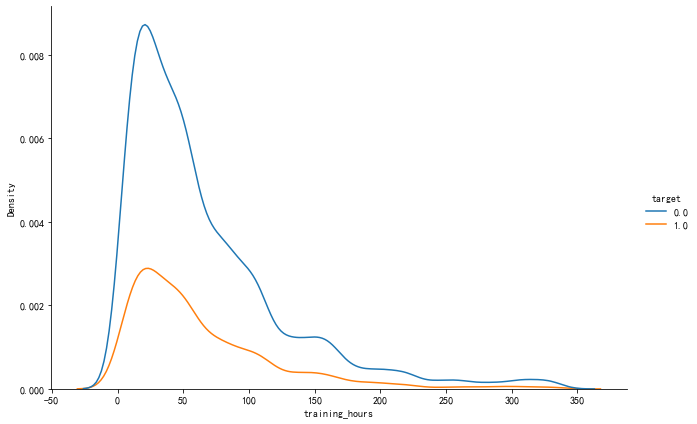
plt.figure(figsize=(10,6))
sns.boxplot(x="target",y="training_hours",data=df_train,palette="Set3")
plt.title("Distribution of training hours",fontsize=15)
plt.xlabel("looking for job change",fontsize=15)
plt.ylabel("training_hours",fontsize=15)
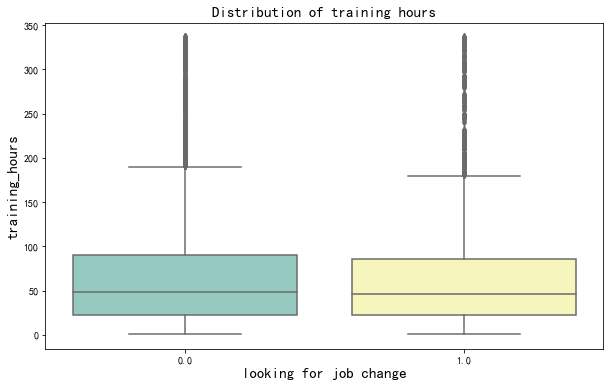
对于找工作的人来说,平均完成的培训时间几乎是一样的。这意味着训练时间在这里没有影响。
pandas_profiling
教你一行代码生成数据分析报告。
import pandas_profiling as pp
report = pp.ProfileReport(train)
report.to_file('report.html')
得到的报告,基本包含本篇所分析的每个特征变量及变量之间的关系。如下👇所示。
相关阅读: