用Python分析一下这个横行霸道的美食
大家好,欢迎来到 Crossin的编程教室 !
秋风起,蟹脚痒。又到了某样“横行霸道”的美食上餐桌的时节,那就是大闸蟹
!
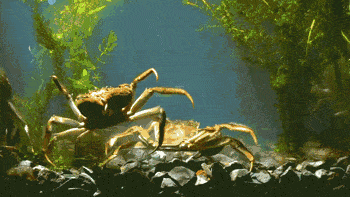
那么,大闸蟹又有哪些特点,都有哪些大闸蟹品牌值得关注呢?
今天,就跟随着本文一看究竟吧!
1. 聊聊大闸蟹
2. 数据采集
2.1. 页面分析
2.2. 采集程序
3. 数据清洗
4. 数据统计
4.1. 商品价格分布
4.2. 评论数分布
4.3. 店铺商品数分布
4.4. 好评率分布
5. 其他

1. 聊聊大闸蟹
螃蟹一般根据生活水域的不同而分为河蟹和海蟹,比如大闸蟹就是河蟹额一种,帝王蟹那种超大的就是海蟹的一类。
大闸蟹都有哪些营养价值呢?
大闸蟹营养丰富,据《本草纲目》记载:螃蟹具有舒筋益气、理胃消食、通经络、散诸热、散瘀血之功效。蟹肉味咸性寒,有清热、化瘀、滋阴之功,可治疗跌打损伤、筋伤骨折、过敏性皮炎。蟹壳煅灰,调以蜂蜜,外敷可治黄蜂蜇伤或其他无名肿毒。蟹肉也是儿童天然滋补品,经常食用可以补充优质蛋白和各种微量元素。
——百科
美味的大闸蟹!
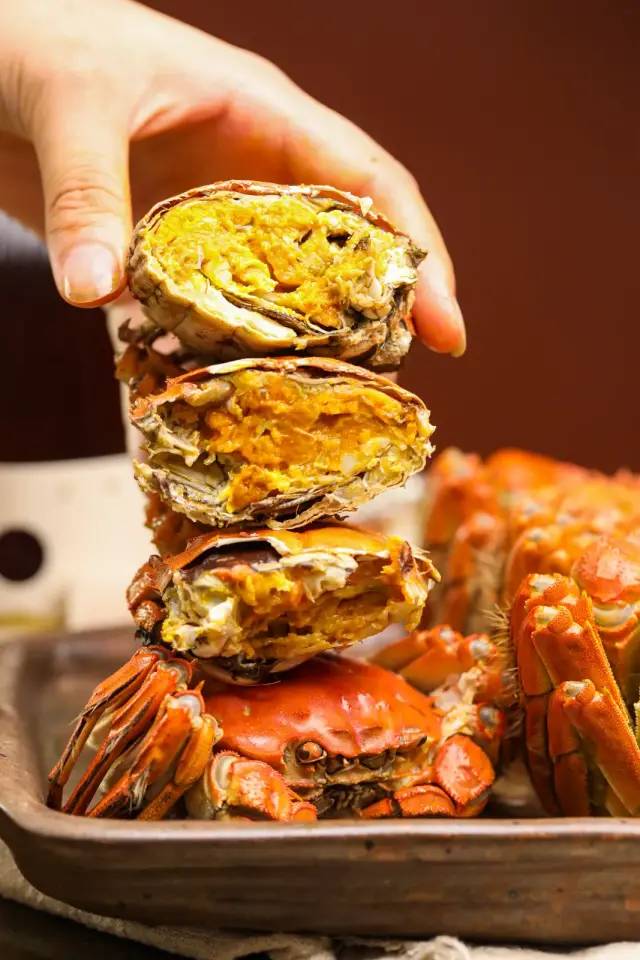
一般我们看网上的大闸蟹商品,出现较多的字眼就是鲜活、公母以及两(重量单位),那么这都是什么含义呢?
所谓鲜活,其实就是指你网购且到你手上的大闸蟹是活的状态,毕竟到手的是死蟹再烹饪谁知道会出现啥异常问题。
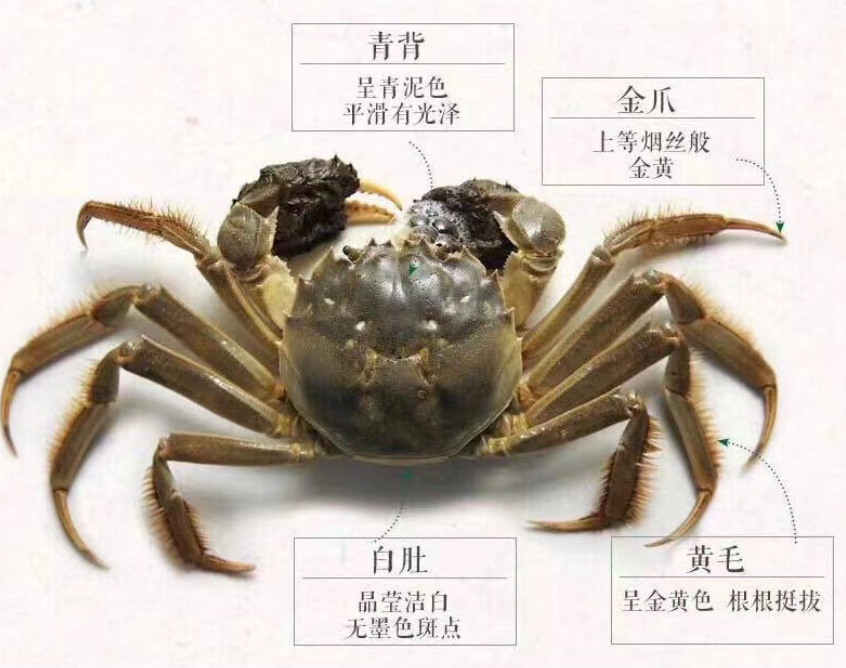
所谓公母,其实就是大闸蟹的性别雌雄,公蟹的肚脐是尖尖的,而母蟹的肚脐是圆的(毕竟要放卵)。一般建议是农历八九月里可以挑母蟹,农历九月过后(也就是国庆节后)优先选公蟹。
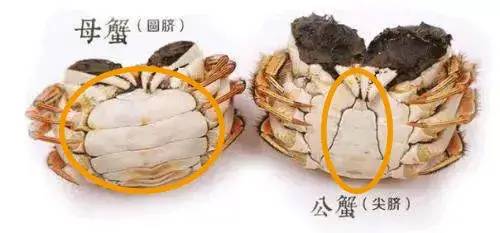
所谓两,就是重量单位指大闸蟹的体重,1两=50g
。当然了,基本上越大越肥美了!!不过,越大价格也越贵~~
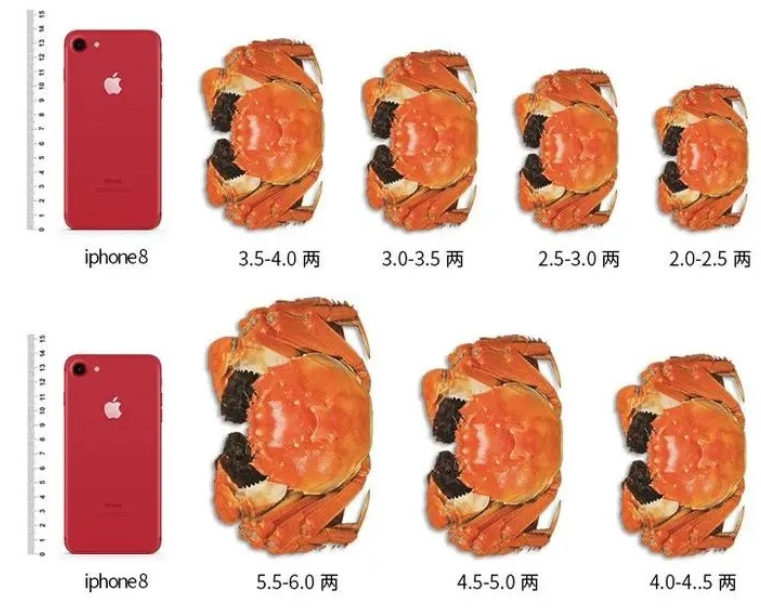
特别注意,在吃大闸蟹的时候,有四个部位不能吃,这些部位主要是有些很多寄生虫和细菌啥的。
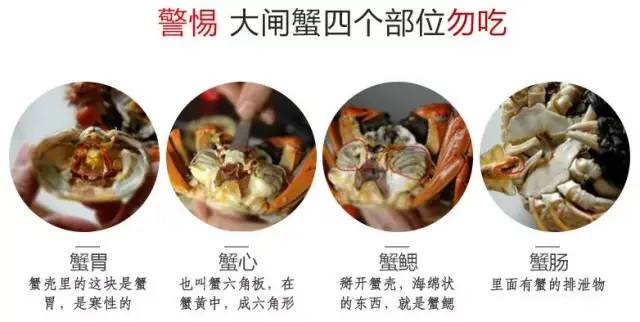
大闸蟹的简单介绍就到这了,接下来我们看看电商平台上的大闸蟹吧!

2. 数据采集
本次采集的是京东商城里带有 中秋节标签的 大闸蟹商品信息,采集过程如下:
2.1. 页面分析
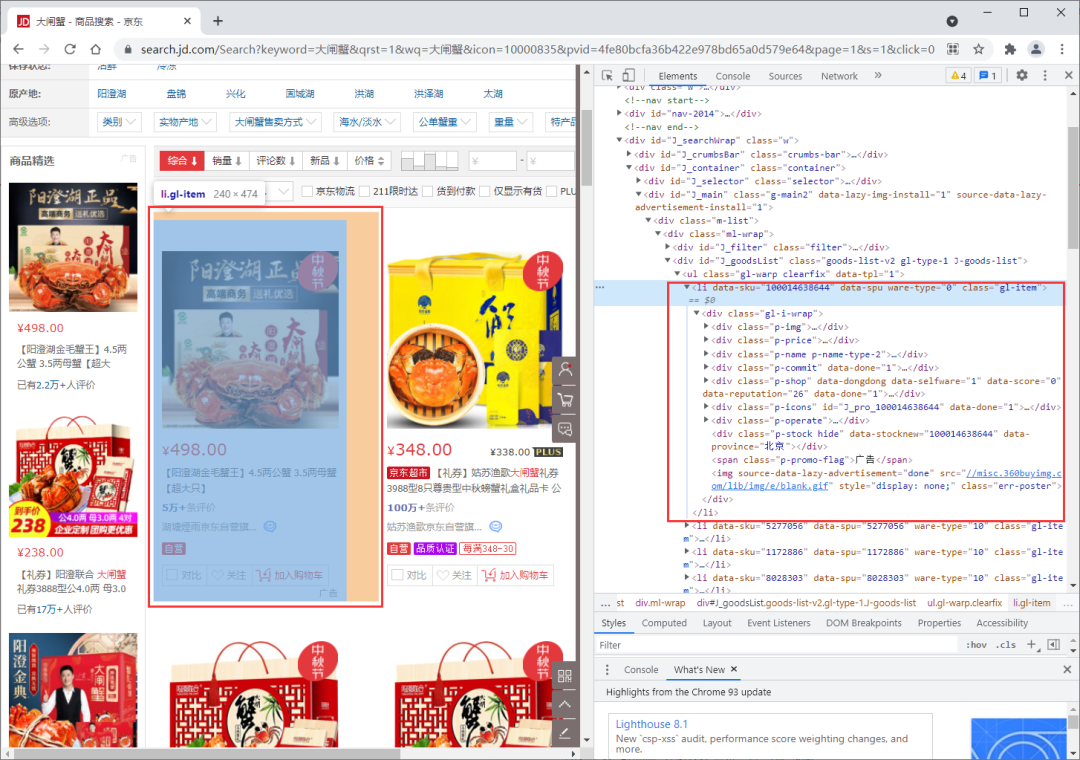
通过进行下滑操作,我们发现单纯从页面来看默认展示30个商品信息,下滑会加载另外30个,此时的网页地址不变;当我们翻页时,发现网页地址发生变化,其中page从1变成了3。于是,我们猜测其实每个页面是两页page,于是尝试手动修改page发现确实如此。最后,我们获得网页地址规律如下:
# page是变化的,其他不变
url= f'https://search.jd.com/Search?keyword=%E5%A4%A7%E9%97%B8%E8%9F%B9&qrst=1&wq=%E5%A4%A7%E9%97%B8%E8%9F%B9&icon=10000835&pvid=4fe80bcfa36b422e978bd65a0d579e64&page={page}'
当然,大家也可以将上面的地址变成基础地址+参数的形式,其中可变参数为keyword
和page
,方便进行其他商品的数据采集,这里我就不展开了。
我们通过请求这个网页地址,可以获取需要的商品信息如下:
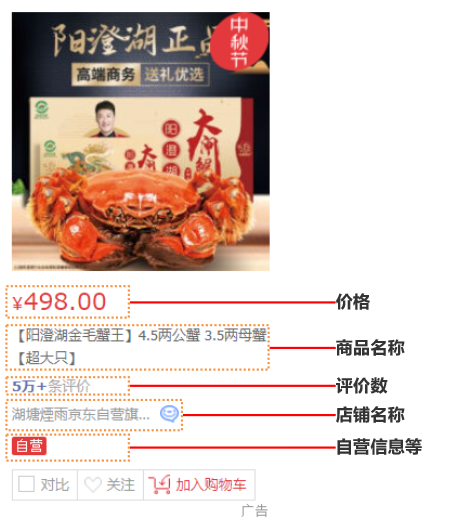
不过,实际操作中我们发现请求到的网页数据中其他信息都包含但是唯独不包含评价数信息,而评价数的信息需要点进去具体的商品页面进行采集。好在我们进到商品页面发现评价信息是以json
数据形式存在,比较好解析,而且接口api
非常明确,可以直接通过商品id
这一个参数即可进行请求获取。
2.2. 采集程序
经过对页面的分析以及一些尝试,我们最终确定了采集方法。
引入需要的库
import requests
import pandas as pd
from lxml import etree
import re
import json
headers = {
# "Accept-Encoding": "Gzip", # 使用gzip压缩传输数据让访问更快
"User-Agent": "Mozilla/5.0 (Windows NT 10.0; Win64; x64) AppleWebKit/537.36 (KHTML, like Gecko) Chrome/83.0.4103.97 Safari/537.36",
# "Cookie": cookie
}
采集页面信息
页面数据采集需要传入的参数只是page
页码即可
def get_html(page):
url= f'https://search.jd.com/Search?keyword=%E5%A4%A7%E9%97%B8%E8%9F%B9&qrst=1&wq=%E5%A4%A7%E9%97%B8%E8%9F%B9&icon=10000835&pvid=4fe80bcfa36b422e978bd65a0d579e64&page={page}'
r = requests.get(url, headers=headers, timeout=6)
return r
采集评论数据
采集评论数据只需要传商品id即可,这里需要注意的是这个接口貌似有访问时间限制或频次限制(我这边采集完是用的代理ip)
# 获取评论信息
def get_comment(productId):
# time.sleep(0.5)
url = 'https://club.jd.com/comment/skuProductPageComments.action?'
params = {
'callback': 'fetchJSON_comment98',
'productId': productId,
'score': 0,
'sortType': 6,
'page': 0,
'pageSize': 10,
'isShadowSku': 0,
'fold': 1,
}
r = requests.get(url, headers=headers, params=params, timeout=6)
comment_data = re.findall(r'fetchJSON_comment98\((.*)\)', r.text)[0]
comment_data = json.loads(comment_data)
comment_summary = comment_data['productCommentSummary']
return comment_summary
解析页面其他信息
页面显示一共41页,所以这里我手动设置的是82页,解析操作采用的是xpath
def get_data():
df = pd.DataFrame(columns=['productId', 'price', 'name', 'shop', '自营'])
for page in range(1,82):
r = get_html(page)
r_html = etree.HTML(r.text)
lis = r_html.xpath('.//li[@class="gl-item"]')
for li in lis:
item = {
"productId": li.xpath('./@data-sku')[0], # id
"price": li.xpath('./div/div[@class="p-price"]/strong/i/text()')[0], # 价格
"name": ''.join( li.xpath('./div/div[@class="p-name p-name-type-2"]/a/em/text()')) ,# 商品名
"shop": li.xpath('./div/div[@class="p-shop"]/span/a/text()')[0], # 店铺名
"自营": li.xpath('./div/div[@class="p-icons"]/i/text()'), # 自营
}
comment_summary = get_comment(item['productId'])
item['commentCount'] = comment_summary['commentCountStr']
item['goodRate'] = comment_summary['goodRate']
df = df.append(item, ignore_index=True)
print(f'\r第{page}/82页数据已经采集', end='')
最终,我们得到的数据如下:


3. 数据清洗
打开存在本地的数据文件,发现里面存在大闸蟹的一些衍生品,比如蟹八件、蟹膏等等,这些商品数据是需要删除的;此外,像name
字段里存在非字符,commentCount
字段里有+
和万
等字眼也需要替换处理;最后就是爬取过程中采集的数据可能存在重复,需要按照productId
去重等等。
数据信息
>>>df.info()
<class 'pandas.core.frame.DataFrame'>
RangeIndex: 2653 entries, 0 to 2652
Data columns (total 7 columns):
# Column Non-Null Count Dtype
--- ------ -------------- -----
0 productId 2653 non-null int64
1 price 2653 non-null float64
2 name 2653 non-null object
3 shop 2651 non-null object
4 自营 2653 non-null object
5 commentCount 2653 non-null object
6 goodRate 2653 non-null float64
dtypes: float64(2), int64(1), object(4)
memory usage: 145.2+ KB
无关数据清理
发现在name
商品名称中,都用到公、母以及两字眼,我们可以根据这个信息进行无关数据清理
>>>df = df[(df['name'].str.contains('公|母'))&(df['name'].str.contains('两'))]
>>>df.info()
<class 'pandas.core.frame.DataFrame'>
Int64Index: 1774 entries, 0 to 2272
Data columns (total 7 columns):
# Column Non-Null Count Dtype
--- ------ -------------- -----
0 productId 1774 non-null int64
1 price 1774 non-null float64
2 name 1774 non-null object
3 shop 1774 non-null object
4 自营 1774 non-null object
5 commentCount 1774 non-null object
6 goodRate 1774 non-null float64
dtypes: float64(2), int64(1), object(4)
memory usage: 110.9+ KB
一下子清理了好多!!
特殊字符处理
df.name = df.name.str.replace(r'\s','',regex=True)
df.commentCount = df.commentCount.str.replace('+','',regex=True).str.replace('万','0000',regex=True)
df.head()

重复数据删除
>>>df.drop_duplicates(subset='productId', inplace=True)
>>>df.info()
<class 'pandas.core.frame.DataFrame'>
Int64Index: 1546 entries, 0 to 2272
Data columns (total 7 columns):
# Column Non-Null Count Dtype
--- ------ -------------- -----
0 productId 1546 non-null int64
1 price 1546 non-null float64
2 name 1546 non-null object
3 shop 1546 non-null object
4 自营 1546 non-null object
5 commentCount 1546 non-null object
6 goodRate 1546 non-null float64
dtypes: float64(2), int64(1), object(4)
memory usage: 96.6+ KB
又清理了不少!!
数据类型转换
我们发现,在各字段数据类型中,commentCount
评论数居然还是数字类型,那就转化一下吧。
df.commentCount = df['commentCount'].astype('int')
数据清洗完毕,我们开始做简单的统计分析展示吧!

4. 数据统计
以下,我们将从商品价格分布、评论数分布、店铺商品数分布和好评率进行统计展示,同时我们也可以将根据商品名称进行解析出公母以及重量相关数据再做探索!
4.1. 商品价格分布
# 直方图
df.price.plot.hist(stacked=True, bins=20)
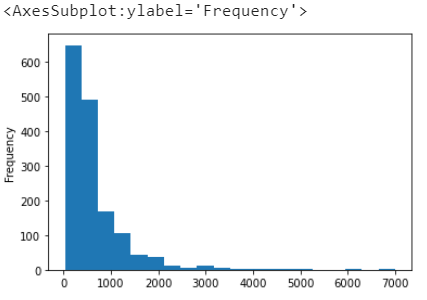
可以看到,大部分价格在1000以内,超过600/1546件商品价格在300以内。
# 箱线图
df[['price']].boxplot(sym="r+")
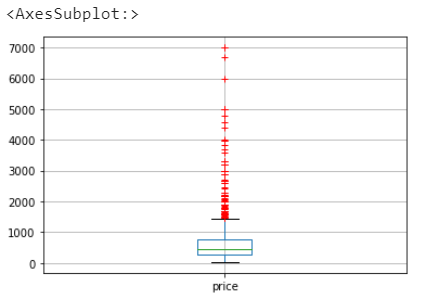
我们按照0-100
,100-200
,200-300
,300-600
,600-1000
,1000+
分类看看,可以发现300-600
区间的商品占比最多!!
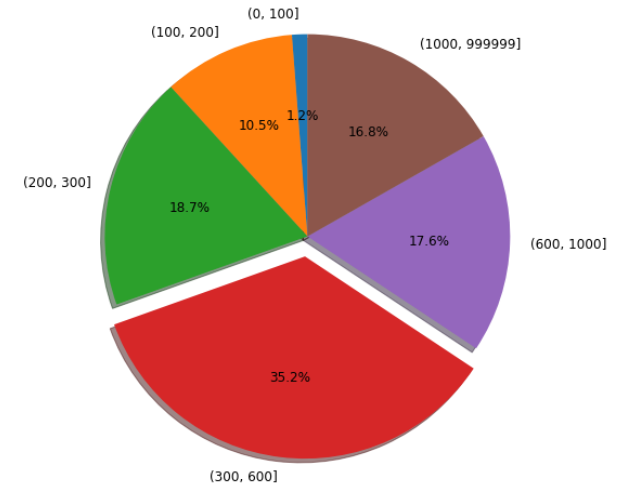
# 绘图代码
import matplotlib.pyplot as plt
from matplotlib import font_manager as fm
bins= [0,100,200,300,600,1000,999999]
price_Num = df['price'].groupby(pd.cut(df.price, bins= bins)).count().to_frame('数量')
labels = price_Num.index
sizes = price_Num['数量']
explode = (0, 0, 0, 0.1, 0, 0)
fig1, ax1 = plt.subplots(figsize=(10,8))
patches, texts, autotexts = ax1.pie(sizes, explode=explode, labels=labels, autopct='%1.1f%%',
shadow=True, startangle=90)
ax1.axis('equal')
# 重新设置字体大小
proptease = fm.FontProperties()
proptease.set_size('large')
plt.setp(autotexts, fontproperties=proptease)
plt.setp(texts, fontproperties=proptease)
plt.show()
商品最贵的几件
可以看到最贵的大闸蟹基本都是重量级的,来自诚蟹一品,不过销量应该一般,毕竟评论数少。不过是真的大公的都有7两多,母的都是5-6两,而常规卖的基本都是4两左右价格400左右!!
# 单元格数据全显示
pd.set_option('display.max_colwidth',1000)
df.nlargest(5,'price',keep='first')

4.2. 评论数分布
大部分的商品评论数集中在200以下
,有5
个商品的评论数超过10万。不过,我们基本可以认定像这种10万+
评论数的商品基本都是买的最多的!
bins= [0,100,200,500,1000,5000,10000,100000,9999999]
comment_Num = df['commentCount'].groupby(pd.cut(df.commentCount, bins= bins)).count().to_frame('数量')
labels = list(comment_Num.index)[:7]
labels.extend(['10万+'])
comment_Num = df['commentCount'].groupby(pd.cut(df.commentCount, bins= bins, labels=labels)).count().to_frame('数量')
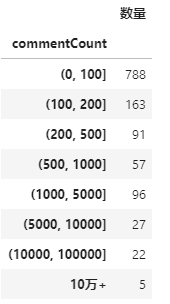
买的人最多的基本都是公蟹4两左右+母蟹3两左右
的8只
组合装,价格在200-400
之间,属于大众消费品吧!
df.nlargest(5,'commentCount',keep='first')

这些商品基本也是你在京东搜索的时候出现在综合推荐前几位的吧!
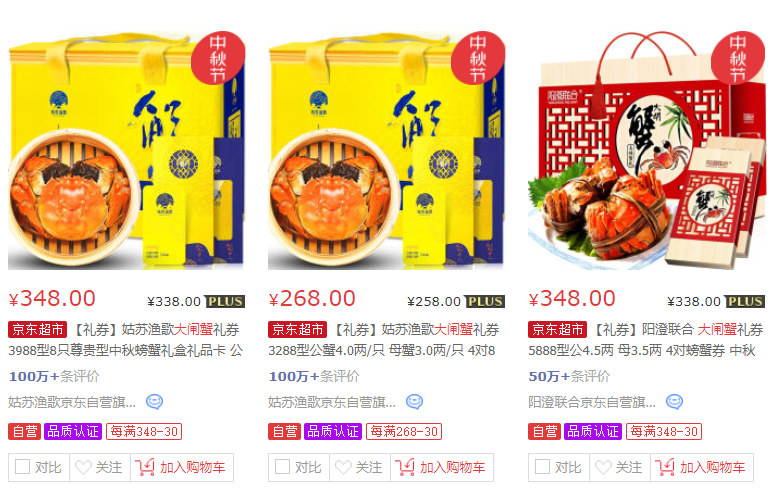
4.3. 店铺商品数分布
诚蟹一品旗舰店是商品数最多的,高达79款,不过整体销量一般,感觉看前面他们家高达6000块以上的礼品盒,大概只做高端吧!
相比之下,姑苏渔歌京东自营旗舰店的商品数量多且销量也多。
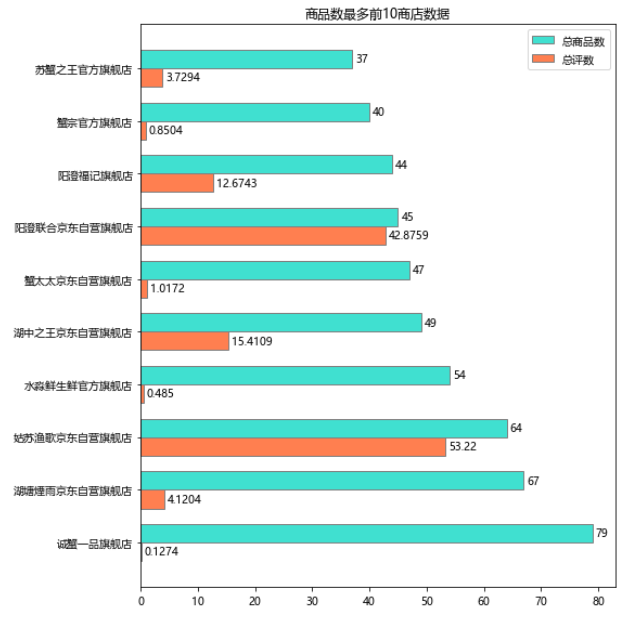
import matplotlib.pyplot as plt
import numpy as np
plt.rcParams['font.sans-serif'] = ['Microsoft YaHei']
plt.rcParams['axes.unicode_minus'] = False
shopNum1= df.groupby('shop').agg(总商品数=('productId','count'),
总评数=('commentCount',sum)
).sort_values(by='总商品数', ascending=False).head(10)
# 设置柱状图颜色
colors = ['turquoise', 'coral']
labels = shopNum1.index
y1 = shopNum1.总商品数
y2 = shopNum1.总评数 / 10000
x = np.arange(len(labels))
width = 0.35
fig, ax = plt.subplots(figsize=(8,8))
rects1 = ax.barh(x + width/2, y1, width, label='总商品数', color=colors[0], edgecolor='grey')
rects2 = ax.barh(x - width/2, y2, width, label='总评数', color=colors[1], edgecolor='grey')
ax.set_title('商品数最多前10商店数据')
y_pos = np.arange(len(labels))
ax.set_yticks(y_pos)
ax.set_yticklabels(labels)
ax.legend()
# 显示数据标签
ax.bar_label(rects1, padding=3)
ax.bar_label(rects2, padding=3)
fig.tight_layout()
plt.show()
我们再看看销量高的店铺都有哪些!
可以看到,三家自营店:今锦上生鲜
京东自营旗舰店、姑苏渔歌
京东自营旗舰店、阳澄联合
京东自营旗舰店 销量遥遥领先!
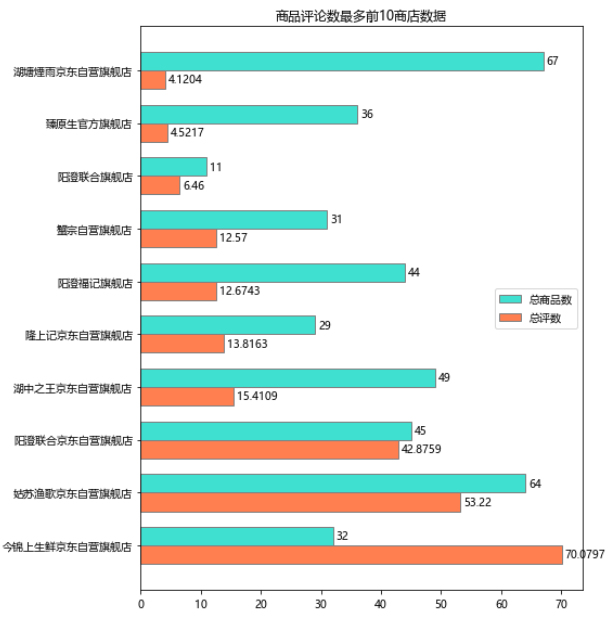
4.4. 好评率分布
只看评价数超过1万
的商品共27件
,有一半商品好评率都在98%
以上,相对来说整体都不错,买就买销量多且好评率高的吧,就是比较稳!
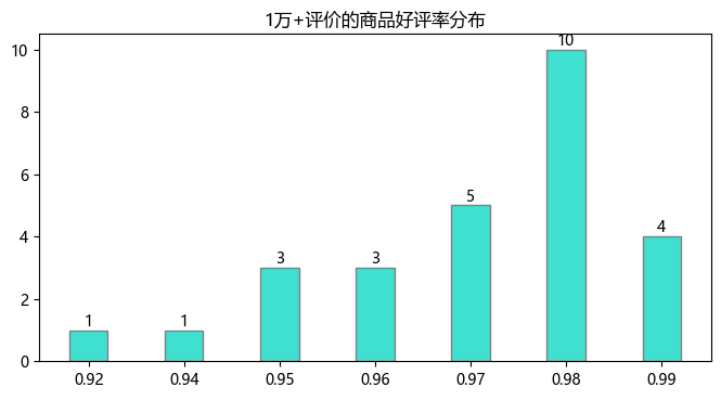
import matplotlib.pyplot as plt
# 中文及负数显示
plt.rcParams['font.sans-serif'] = ['Microsoft YaHei']
plt.rcParams['axes.unicode_minus'] = False
# 创建画布
fig, ax = plt.subplots(figsize=(8, 4), dpi=100)
# 案例数据
data = goodRateNum.数量
# 作图参数
index = goodRateNum.index.astype('str')
bar_width = 0.4
# 设置柱状图颜色
colors = ['turquoise']
# 柱状图
bar = plt.bar(index, data, bar_width, color=colors[0], edgecolor='grey')
# 设置标题
ax.set_title('1万+评价的商品好评率分布', fontsize=12)
# 显示数据标签
ax.bar_label(bar, label_type='edge')
plt.show()
关于按照商品名称中的大闸蟹重量来进行深度探索,大家可以自行试试哦!

5. 其他
其实,如果你想更深一步了解不同商品的 用户评价,可以参考 2.2.采集程序
中对评价信息的部分,这部分做循环然后就可获取全部的评论数据,然后再进行对应数据分析。
关于京东大闸蟹,通过商品名称我们可以得到以下热词云图:
基本都是公蟹
、母蟹
和礼券
等关键字咯。。。
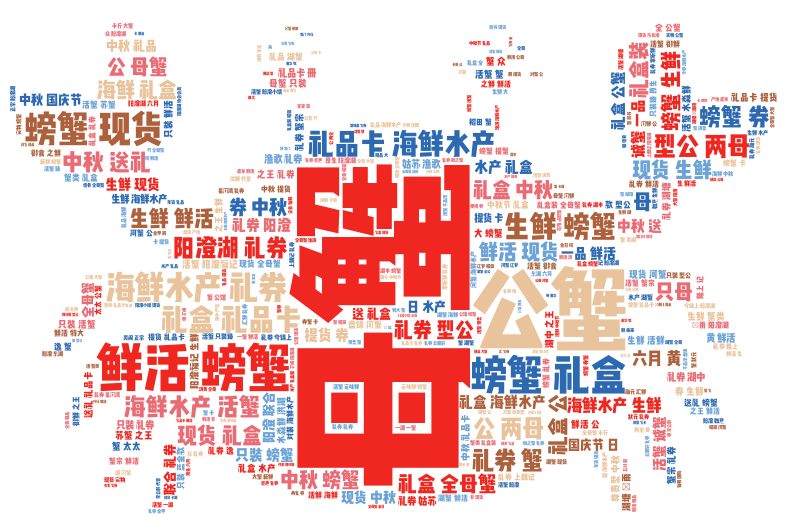
看来大家还是普遍都更喜欢母蟹啊!
以上,就是本次全部内容。要是喜欢,就请我吃个大闸蟹呗!
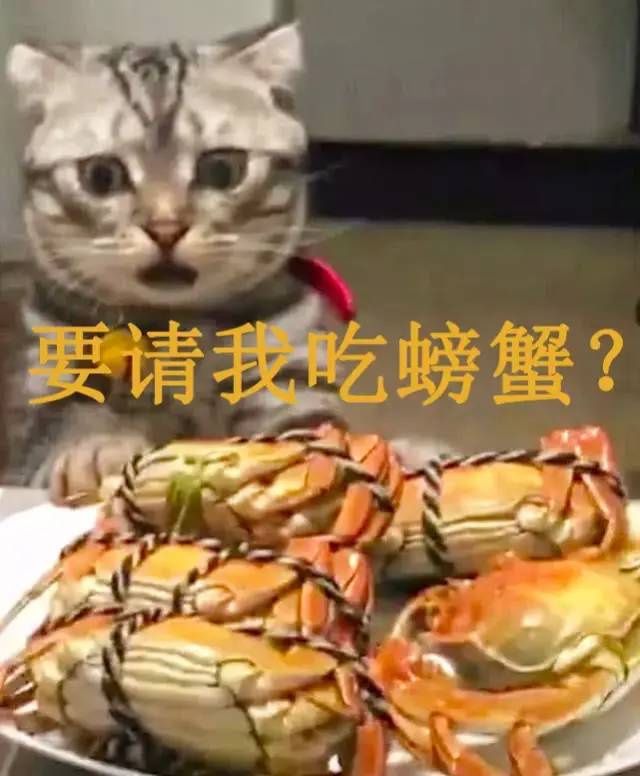
如需获取本期案例数据及ipynb
演示文件,请在公众号 Crossin的编程教室 后台回复关键字 大闸蟹
作者:道才
_往期文章推荐_