神经网络的可解释性综述!
地址|https://zhuanlan.zhihu.com/p/368755357
本文仅作为学术分享,著作权归属原作者,侵删
本文以 A Survey on Neural Network Interpretability 读后感为主,加上自身的补充,浅谈神经网络的可解释性。
本文按照以下的章节进行组织:
人工智能可解释性的背景意义 神经网络可解释性的分类 总结
01
解释(Explanations),是指需要用某种语言来描述和注解
可解释的边界(Explainable Boundary),是指可解释性能够提供解释的程度
可理解的术语(Understandable Terms),是指构成解释的基本单元
高可靠性的要求
伦理/法规的要求
作为其他科学研究的工具
02
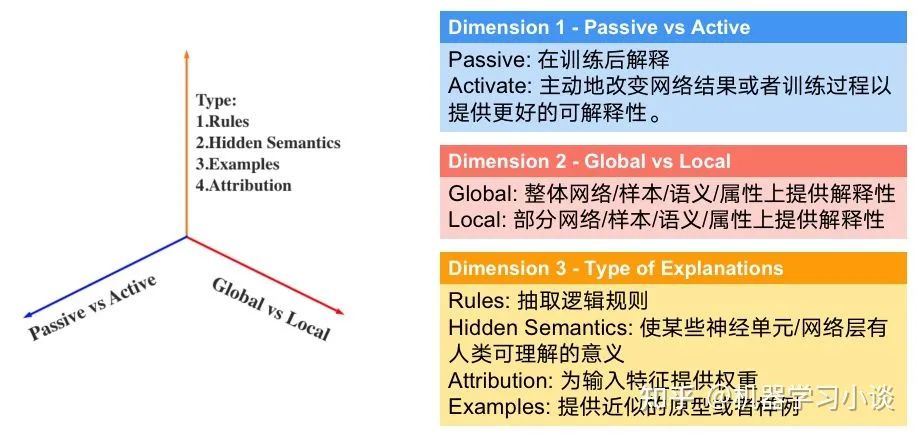
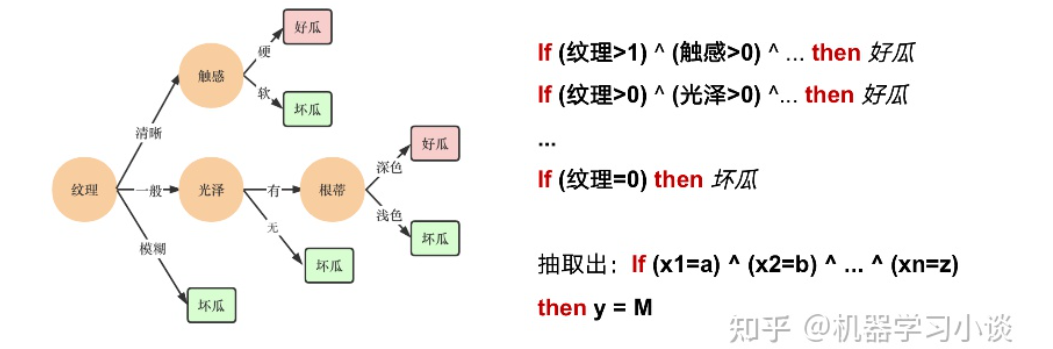
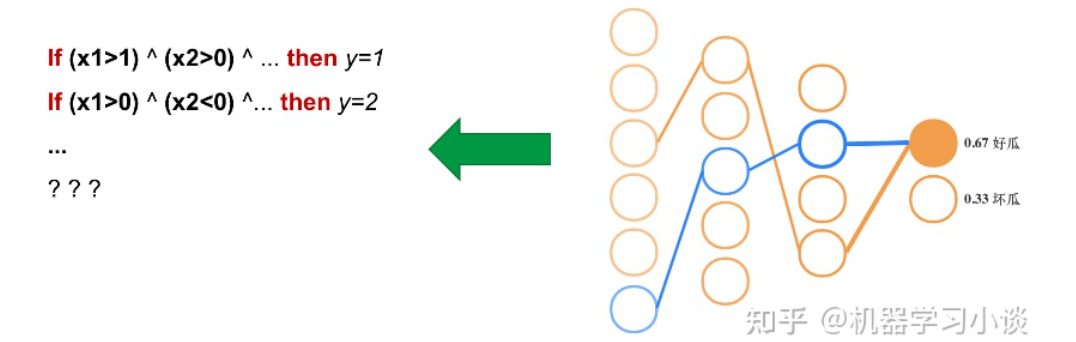
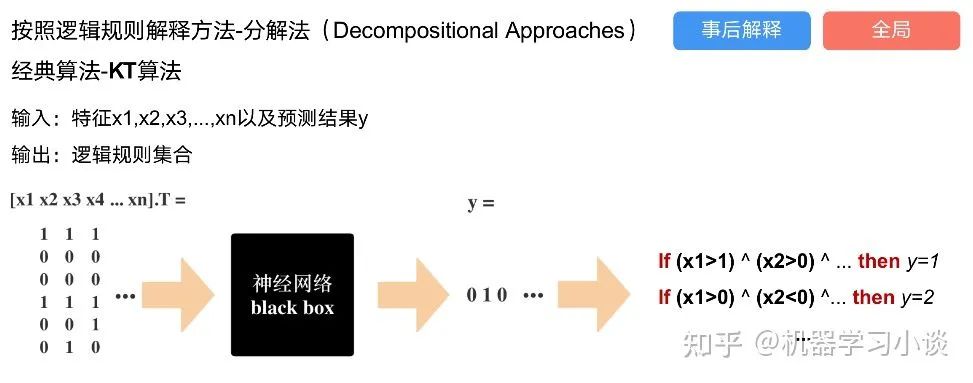

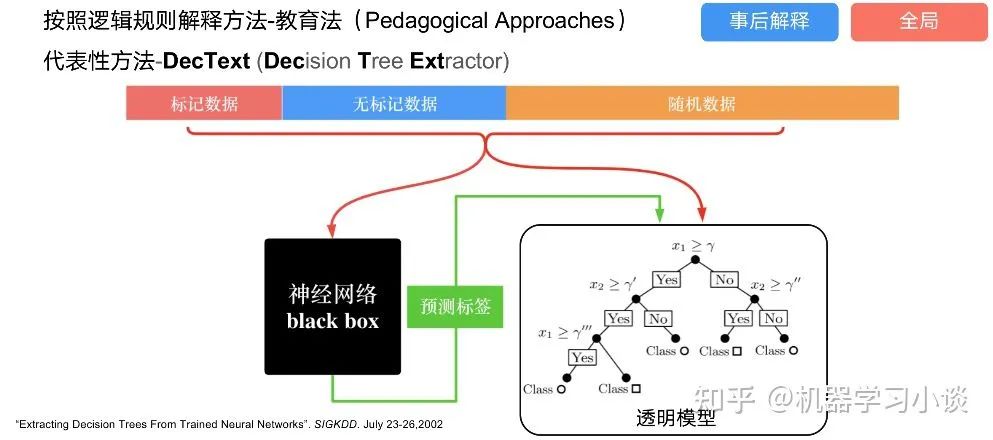
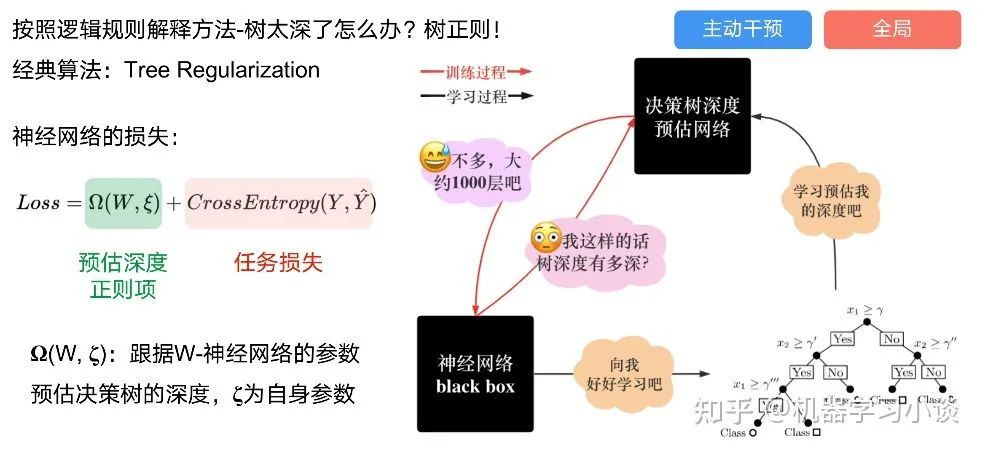
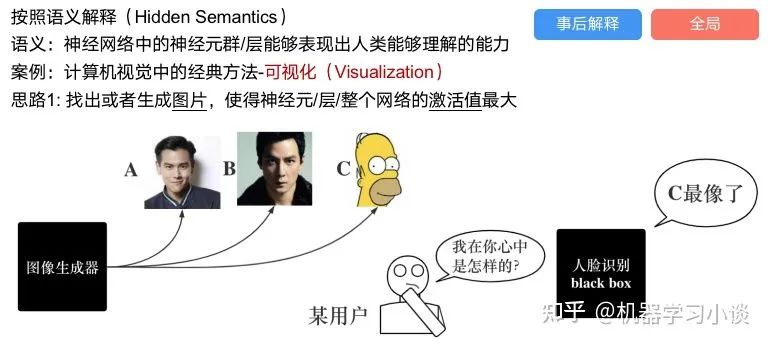
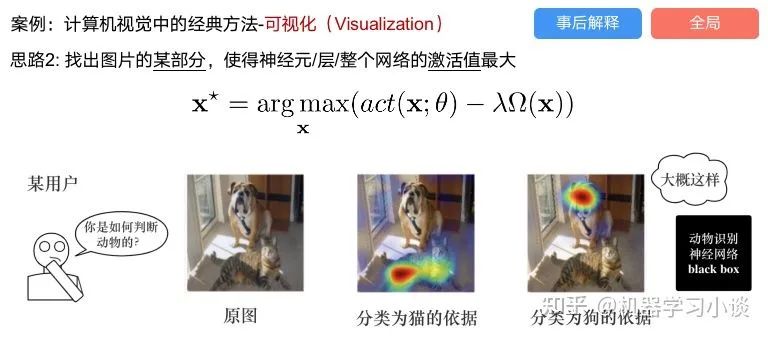
featuremap_layout
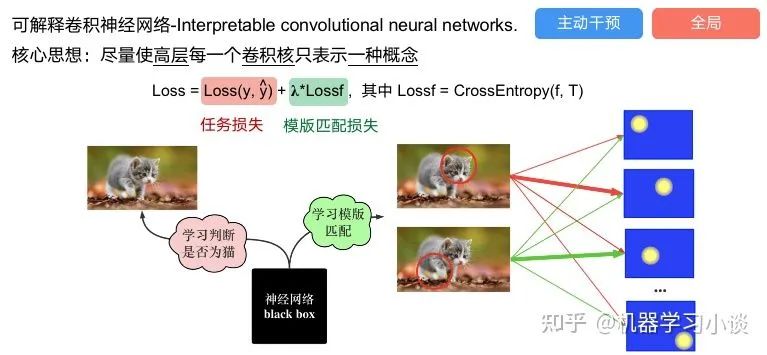
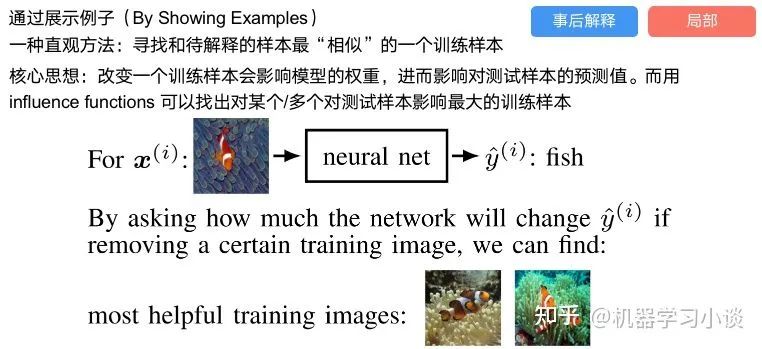
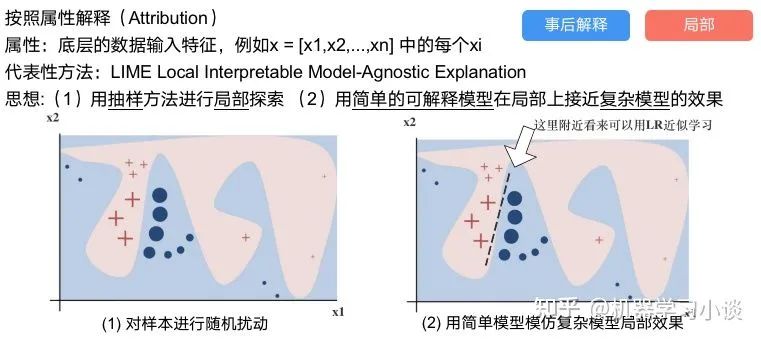
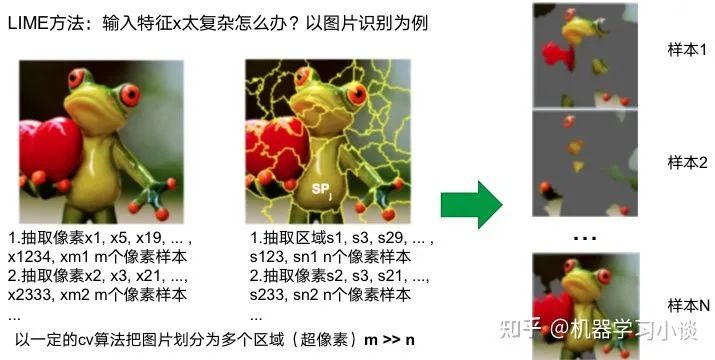
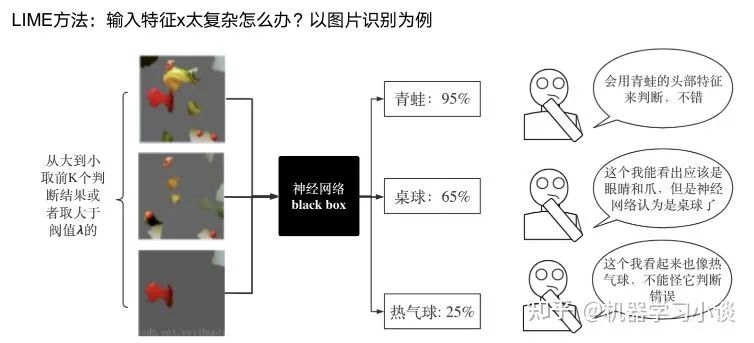
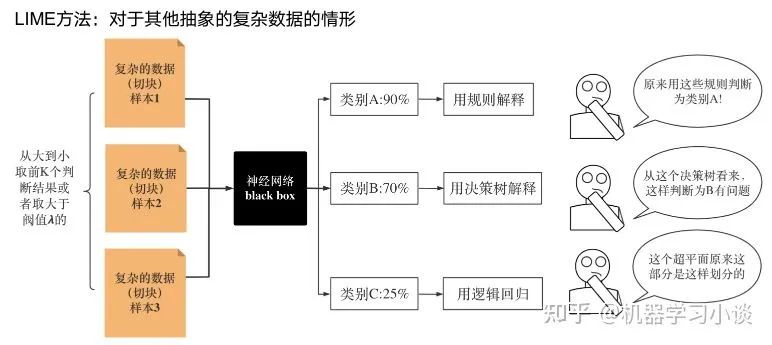
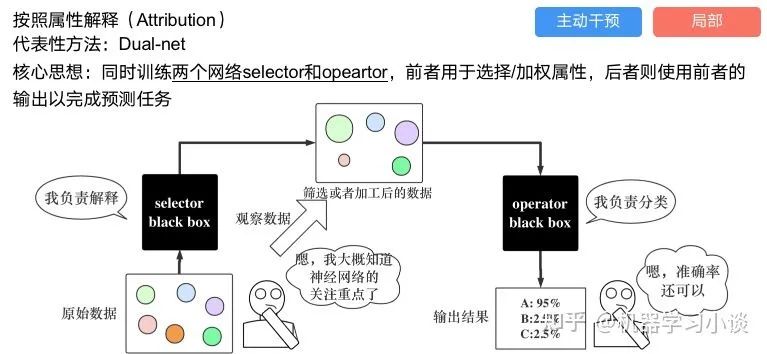
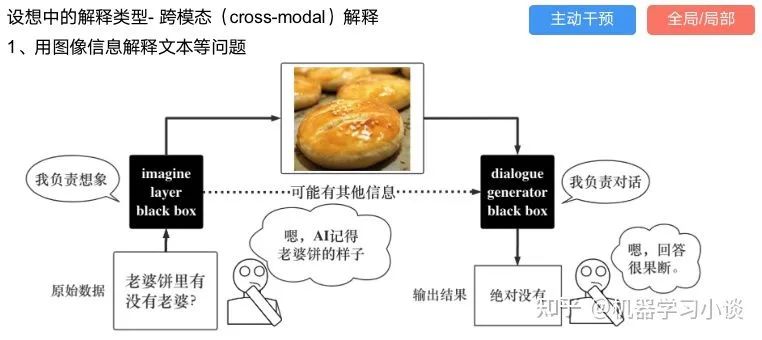
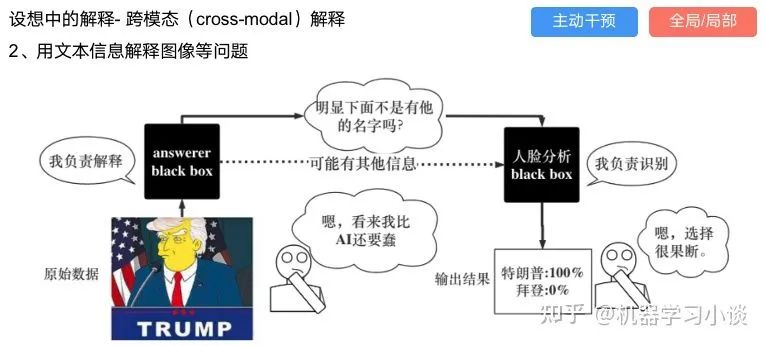
03
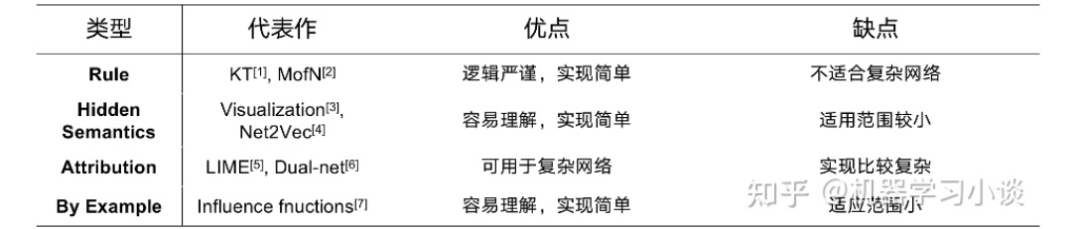
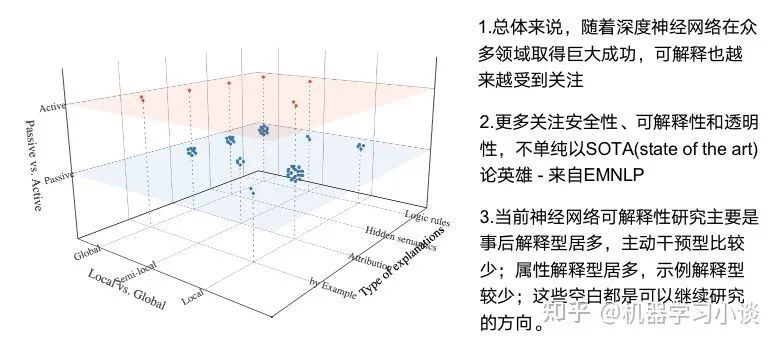
参考
^“Extracting Decision Trees From Trained Neural Networks”. SIGKDD. July 23-26,2002 https://dl.acm.org/doi/10.1145/775047.775113
^ M. Wu, S. Parbhoo, M. C. Hughes, R. Kindle, L. A. Celi, M. Zazzi, V. Roth, and F. Doshi-Velez, “Regional tree regularization for interpretability in deep neural networks.” in AAAI, 2020, pp. 6413–6421. https://arxiv.org/abs/1908.04494
^K. Simonyan, A. Vedaldi, and A. Zisserman, “Deep inside convolutional networks: Visualising image classification models and saliency maps,” arXiv preprint arXiv:1312.6034, 2013.
^Q. Zhang, Y. Nian Wu, and S.-C. Zhu, “Interpretable convolutional neural networks,” in Proceedings of the IEEE Conference on Computer Vision and Pattern Recognition, 2018.
^P. W. Koh and P. Liang, “Understanding black-box predictions via influence functions,” in Proceedings of the 34th International Conference on Machine Learning-Volume 70, 2017.
^M. T. Ribeiro, S. Singh, and C. Guestrin, “Why should i trust you?: Explaining the predictions of any classifier,” in Proceedings of the 22nd ACM SIGKDD international conference on knowledge discovery and data mining, 2016.
^M. Wojtas and K. Chen, “Feature importance ranking for deep learning,” Advances in Neural Information Processing Systems, vol. 33, 2020.
^Open Domain Dialogue Generation with Latent Images Z Yang, W Wu, H Hu, C Xu, Z Li - arXiv preprint arXiv:2004.01981, 2020 - arxiv.org https://arxiv.org/abs/2004.01981
——The End——
读者,你好!我们建立了微信学习交流群,欢迎扫码进群讨论!
微商、广告无关人员请绕道!谢谢合作!
