Swin Transformer的继任者(上)
点蓝色字关注“机器学习算法工程师”
设为星标,干货直达!
近期,随着PVT和Swin Transformer的成功,让我们看到了将ViT应用在dense prediction的backbone的巨大前景。PVT的核心是金字塔结构,同时通过对attention的keys和values进行downsample来进一步减少计算量,但是其计算复杂度依然和图像大小()的平成正比。而Swin Transformer在金字塔结构基础上提出了window attention,这其实本质上是一种local attention,并通过shifted window来建立cross-window的关系,其计算复杂度和图像大小()成正比。基于local attention的模型计算复杂低,但是也丧失了global attention的全局感受野建模能力。近期,在Swin Transformer之后也有一些基于local attention的工作,它们从不同的方面来提升模型的全局建模能力。
Twins
美团提出的Twins思路比较简单,那就是将local attention和global attention结合在一起。Twins主体也采用金字塔结构,但是每个stage中交替地采用LSA(Locally-grouped self-attention)和GSA(Global sub-sampled attention),这里的LSA其实就是Swin Transformer中的window attention,而GSA就是PVT中采用的对keys和values进行subsapmle的MSA。LSA用来提取局部特征,而GSA用来实现全局感受野:
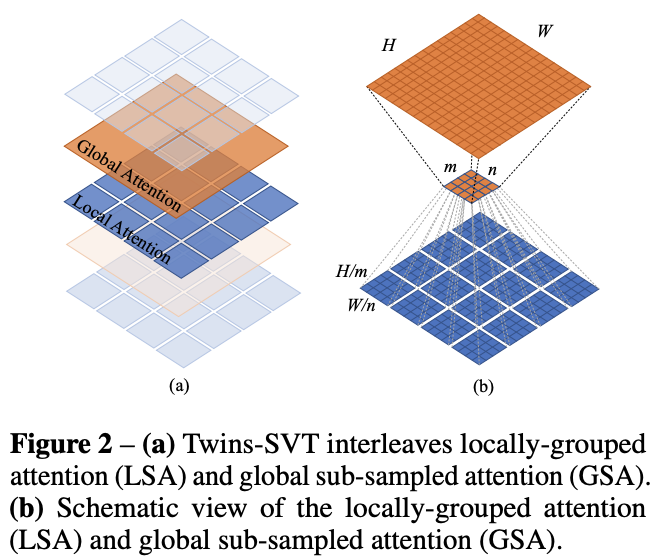
此外,Twins还引入了美团之前论文CPVT提出的PEG(position encoding generator)来进行位置编码,具体是在每个stage的第一个transfomer encoder后插入一个PEG(具体实现上是一个3x3的depth-wise conv)。如果将PVT中的位置编码用PEG替换(称为Twins-PCPVT),那么模型效果也有一个明显的提升。
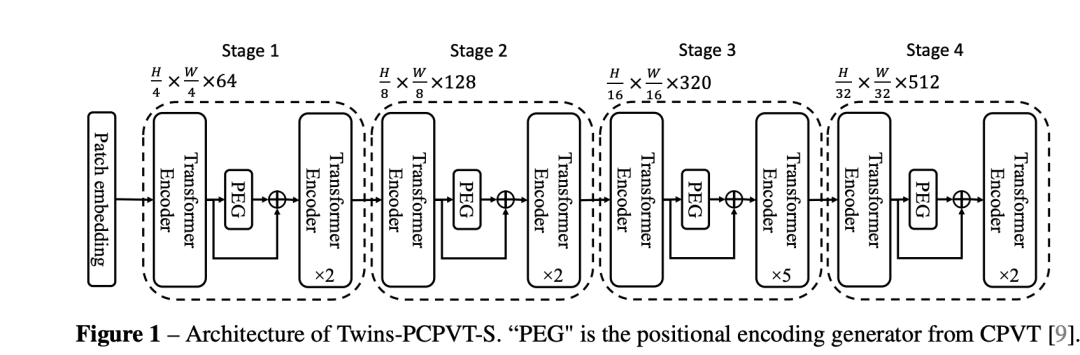
同样地,用了PEG后,可以将window attention中的相对位置编码也去掉了(相比Swin Transformer),最终的模型称为Twins-SVT。在224x224输入的ImageNet数据集上,可以看到Twins-SVT分类效果超过了Swin,而且模型参数和计算量均更低。
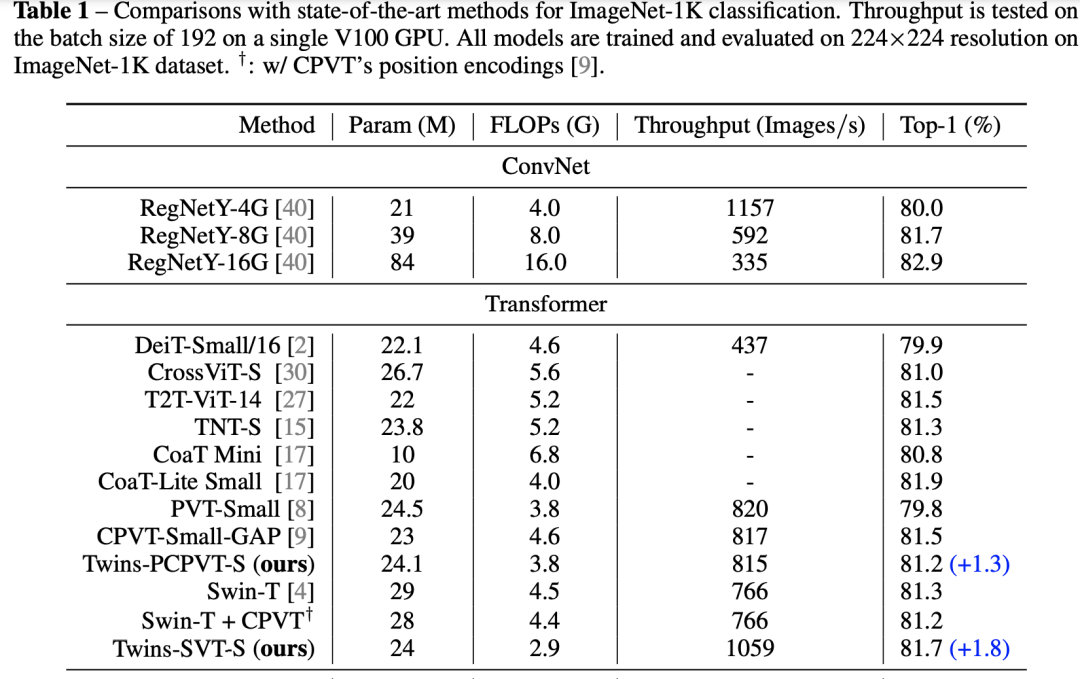
在COCO数据集上,基于Mask R-CNN模型,Twins-SVT也比Swin模型效果要好,而且FLOPs更低,不过这是在800x600图片大小下测试的。毕竟GSA计算复杂度还是和图像大小的平方成正比,当图像输入原来越大时,Twins-SVT也会像PVT那样计算量增加迅速,但是Swin模型是线性增长。
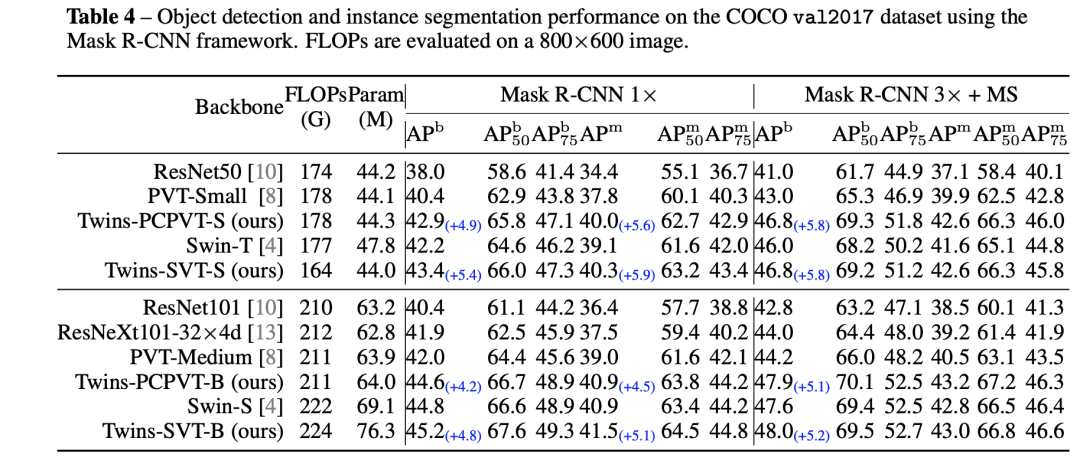
MSG-Transformer
华为提出的MSG-Transformer主要思路是为每个window增加一个信使token(messenger token, MSG),这个不同的windows通过MSG token来建立联系,具体的操作是对MSG token进行shuffle。下图中图像共分为个windows(绿色线条),而每个windows组成一个shuffle region;每个Window都包含一个MSG token,经过window attention之后,同一个shuffle region的MSG token将先进行shuffle,最后才送入MLP中。
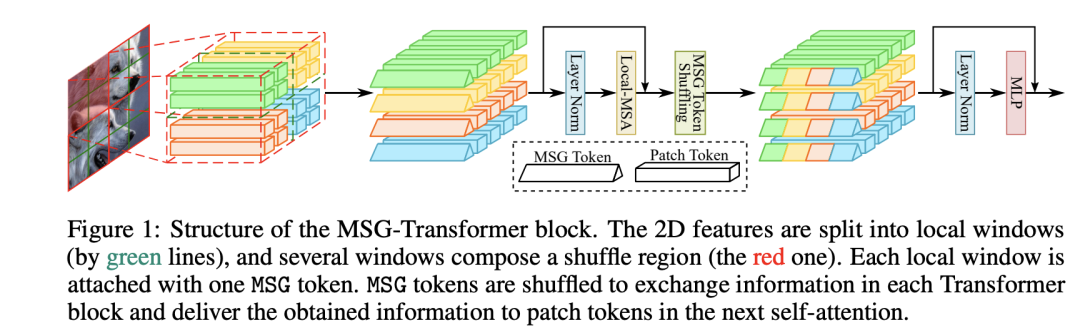
对于一个shuffle region,这里记其大小为,其MSG tokens组合在一起记为,这里是特征维度大小。MSG token的shuffle可以通过reshape->transpose->reshape来实现:
其实就是对MSG tokens的特征进行shuffle,这样shuffle后每个window的MSG token将包含其它windows的部分MSG token特征,从而完成不同windows之间的消息传递:
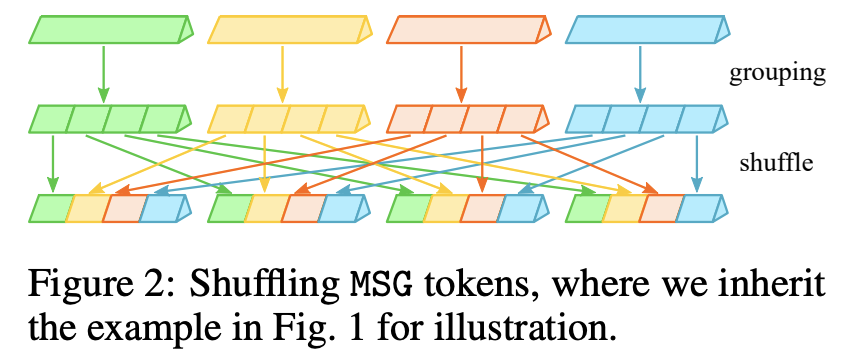
而MSG Transformer主体也采用金字塔结构,不同的stage的取值不同,对于分类任务,各个stage的分别为4,4,2,1。在实现上,我们可以将同一个shuffle region区域放在维度1,而总的shuffle regions和Batch放在第一个维度,这样就非常实现MSG tokens的shuffle:
def window_partition(x, window_size, shuf_size):
"""
Args:
x: (B, H, W, C)
window_size (int): window size
shuf_size (int): shuffle region size
Returns:
windows: (B*num_region, shuf_size**2, window_size**2, C)
"""
B, H, W, C = x.shape
x = x.view(B, H // window_size // shuf_size, shuf_size, window_size,
W // window_size // shuf_size, shuf_size, window_size, C)
windows = x.permute(0, 1, 4, 2, 5, 3, 6, 7).contiguous().view(-1, shuf_size**2, window_size**2, C)
return windows
def shuffel_msg(x):
# (B, G, win**2+1, C)
B, G, N, C = x.shape
if G == 1:
return x
msges = x[:, :, 0] # (B, G, C)
assert C % G == 0
msges = msges.view(-1, G, G, C//G).transpose(1, 2).reshape(B, G, 1, C)
x = torch.cat((msges, x[:, :, 1:]), dim=2)
return x
MSG Transformer的window attention和Swin Transformer一样也采用相对位置编码,但是多了一个MSG token,所以相对位置编码多了两个参数(其它patch tokens相对MSG token,MSG token相对其它patch tokens)。另外在每个stage开始的token merging操作,对MSG token也采取类似的处理:2x2个windows的MSG token进行concat,并进行线性变换。
MSG Transformer引入的MSG token对计算量和模型参数都影响不大,所以其和Swin Transformer一样其计算复杂度线性于图像大小。在ImageNet上,其模型效果和Swin接近,但其在CPU上速度较快:
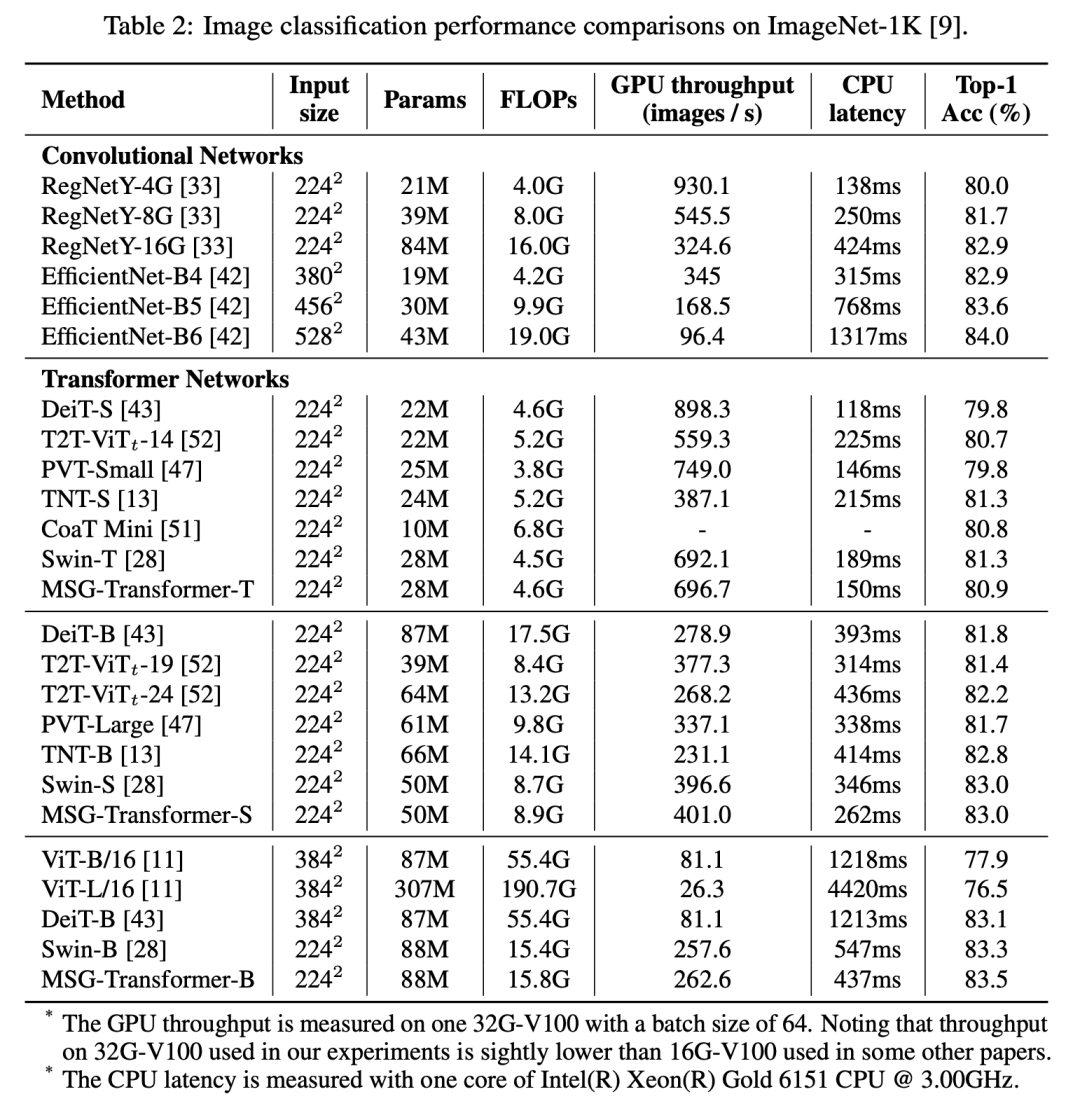
在COCO数据集上,基于Mask R-CNN模型,也可以和Swin模型取得类似的效果:
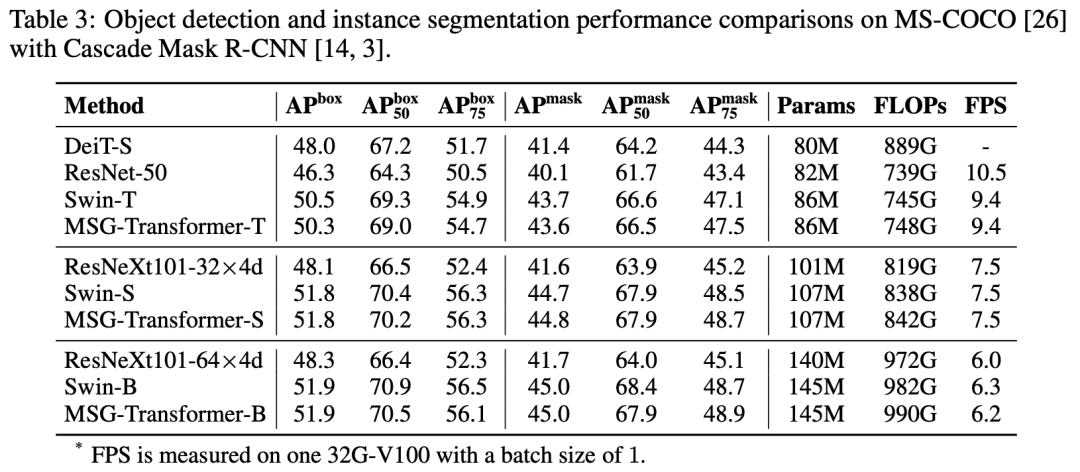
参考
Shuffle Transformer: Rethinking Spatial Shuffle for Vision Transformer Twins: Revisiting the Design of Spatial Attention in Vision Transformers Glance-and-Gaze Vision Transformer MSG-Transformer: Exchanging Local Spatial Information by Manipulating Messenger Tokens Pyramid Vision Transformer: A Versatile Backbone for Dense Prediction without Convolutions Swin Transformer: Hierarchical Vision Transformer using Shifted Windows Demystifying Local Vision Transformer: Sparse Connectivity, Weight Sharing, and Dynamic Weight
推荐阅读
谷歌AI用30亿数据训练了一个20亿参数Vision Transformer模型,在ImageNet上达到新的SOTA!
"未来"的经典之作ViT:transformer is all you need!
PVT:可用于密集任务backbone的金字塔视觉transformer!
涨点神器FixRes:两次超越ImageNet数据集上的SOTA
不妨试试MoCo,来替换ImageNet上pretrain模型!
机器学习算法工程师
一个用心的公众号