ResNet及其变体结构梳理与总结
共 19359字,需浏览 39分钟
·
2021-12-14 21:00
点击上方“小白学视觉”,选择加"星标"或“置顶”
重磅干货,第一时间送达
【导读】2020年,在各大CV顶会上又出现了许多基于ResNet改进的工作,比如:Res2Net,ResNeSt,IResNet,SCNet等等。为了更好的了解ResNet整个体系脉络的发展,我们特此对ResNet系列重新梳理,并制作了一个ResNet专题,希望能帮助大家对ResNet体系有一个更深的理解。本篇文章我们将主要讲解ResNet、preResNet、ResNext以及它们的代码实现。
论文链接:https://arxiv.org/abs/1512.03385
代码地址:https://github.com/KaimingHe/deep-residual-networks
pytorch版:https://github.com/Cadene/pretrained-models.pytorch
ResNet的关键点是:
利用残差结构让网络能够更深、收敛速度更快、优化更容易,同时参数相对之前的模型更少、复杂度更低
ResNet大量使用了批量归一层,而不是Dropout。
对于很深的网络(超过50层),ResNet使用了更高效的瓶颈(bottleneck)结构极大程度上降低了参数计算量。
ResNet的残差结构
为了解决退化问题,我们引入了一个新的深度残差学习block,在这里,对于一个堆积层结构(几层堆积而成)当输入为时,其学习到的特征记为,现在我们希望其可以学习到残差 ,这样其实原始的学习特征是 。之所以这样是因为残差学习相比原始特征直接学习更容易。当残差为0时,此时堆积层仅仅做了恒等映射,至少网络性能不会下降,实际上残差不会为0,这也会使得堆积层在输入特征基础上学习到新的特征,从而拥有更好的性能。
本质也就是不改变目标函数 ,将网络结构拆成两个分支,一个分支是残差映射,一个分支是恒等映射,于是网络仅需学习残差映射即可。对于上述残差单元,我们可以从数学的角度来分析一下,首先上述结构可表示为:
其中和分别表示的是第个残差单元的输入和输出,注意每个残差单元一般包含多层结构。是残差函数,表示学习到的残差,而表示恒等映射,是ReLU激活函数。基于上式,我们求得从浅层到深层的学习特征为:
利用链式规则,可以求得反向过程的梯度:
式子的第一个因子 表示的损失函数到达的梯度,小括号中的1表明短路机制可以无损地传播梯度,而另外一项残差梯度则需要经过带有weights的层,梯度不是直接传递过来的。残差梯度不会那么巧全为-1,而且就算其比较小,有1的存在也不会导致梯度消失。所以残差学习会更容易。要注意上面的推导并不是严格的证明。
残差结构为什么有效?
自适应深度:网络退化问题就体现了多层网络难以拟合恒等映射这种情况,也就是说难以拟合,但使用了残差结构之后,拟合恒等映射变得很容易,直接把网络参数全学习到为0,只留下那个恒等映射的跨层连接即可。于是当网络不需要这么深时,中间的恒等映射就可以多一点,反之就可以少一点。(当然网络中出现某些层仅仅拟合恒等映射的可能性很小,但根据下面的第二点也有其用武之地;另外关于为什么多层网络难以拟合恒等映射,这涉及到信号与系统的知识见:https://www.zhihu.com/question/293243905/answer/484708047)
差分放大器:假设最优更接近恒等映射,那么网络更容易发现除恒等映射之外微小的波动
模型集成:整个ResNet类似于多个网络的集成,原因是删除ResNet的部分网络结点不影响整个网络的性能,但VGGNet会崩溃,具体可以看这篇NIPS论文:Residual Networks Behave Like Ensembles of Relatively Shallow Networks
缓解梯度消失:针对一个残差结构对输入求导就可以知道,由于跨层连接的存在,总梯度在对的导数基础上还会加1
下面给出一个直观理解图:
如上图所示,左边来了一辆装满了“梯度”商品的货车,来领商品的客人一般都要排队一个个拿才可以,如果排队的人太多,后面的人就没有了。于是这时候派了一个人走了“快捷通道”,到货车上领了一部分“梯度”,直接送给后面的人,这样后面排队的客人就能拿到更多的“梯度”。
我们来计算一下1*1卷积的计算量优势:首先看上图右边的bottleneck结构,对于256维的输入特征,参数数目:1x1x256x64+3x3x64x64+1x1x64x256=69632,如果同样的输入输出维度但不使用1x1卷积,而使用两个3x3卷积的话,参数数目为(3x3x256x256)x2=1179648。简单计算下就知道了,使用了1x1卷积的bottleneck将计算量简化为原有的5.9%,收益超高。
ResNet网络设计结构:
def conv3x3(in_planes, out_planes, stride=1):
"""3x3 convolution with padding"""
return nn.Conv2d(in_planes, out_planes, kernel_size=3, stride=stride,
padding=1, bias=False)
class Bottleneck(nn.Module):
expansion = 4
def __init__(self, inplanes, planes, stride=1, downsample=None):
super(Bottleneck, self).__init__()
self.conv1 = nn.Conv2d(inplanes, planes, kernel_size=1, bias=False)
self.bn1 = nn.BatchNorm2d(planes)
self.conv2 = nn.Conv2d(planes, planes, kernel_size=3, stride=stride,
padding=1, bias=False)
self.bn2 = nn.BatchNorm2d(planes)
self.conv3 = nn.Conv2d(planes, planes * 4, kernel_size=1, bias=False)
self.bn3 = nn.BatchNorm2d(planes * 4)
self.relu = nn.ReLU(inplace=True)
self.downsample = downsample
self.stride = stride
def forward(self, x):
residual = x
out = self.conv1(x)
out = self.bn1(out)
out = self.relu(out)
out = self.conv2(out)
out = self.bn2(out)
out = self.relu(out)
out = self.conv3(out)
out = self.bn3(out)
if self.downsample is not None:
residual = self.downsample(x)
out += residual
out = self.relu(out)
return out
class ResNet(nn.Module):
def __init__(self, block, layers, num_classes, grayscale):
self.inplanes = 64
if grayscale:
in_dim = 1
else:
in_dim = 3
super(ResNet, self).__init__()
self.conv1 = nn.Conv2d(in_dim, 64, kernel_size=7, stride=2, padding=3,
bias=False)
self.bn1 = nn.BatchNorm2d(64)
self.relu = nn.ReLU(inplace=True)
self.maxpool = nn.MaxPool2d(kernel_size=3, stride=2, padding=1)
self.layer1 = self._make_layer(block, 64, layers[0])
self.layer2 = self._make_layer(block, 128, layers[1], stride=2)
self.layer3 = self._make_layer(block, 256, layers[2], stride=2)
self.layer4 = self._make_layer(block, 512, layers[3], stride=2)
self.avgpool = nn.AvgPool2d(7, stride=1, padding=2)
#self.fc = nn.Linear(2048 * block.expansion, num_classes)
self.fc = nn.Linear(2048, num_classes)
for m in self.modules():
if isinstance(m, nn.Conv2d):
n = m.kernel_size[0] * m.kernel_size[1] * m.out_channels
m.weight.data.normal_(0, (2. / n)**.5)
elif isinstance(m, nn.BatchNorm2d):
m.weight.data.fill_(1)
m.bias.data.zero_()
def _make_layer(self, block, planes, blocks, stride=1):
downsample = None
if stride != 1 or self.inplanes != planes * block.expansion:
downsample = nn.Sequential(
nn.Conv2d(self.inplanes, planes * block.expansion,
kernel_size=1, stride=stride, bias=False),
nn.BatchNorm2d(planes * block.expansion),
)
layers = []
layers.append(block(self.inplanes, planes, stride, downsample))
self.inplanes = planes * block.expansion
for i in range(1, blocks):
layers.append(block(self.inplanes, planes))
return nn.Sequential(*layers)
def forward(self, x):
x = self.conv1(x)
x = self.bn1(x)
x = self.relu(x)
x = self.maxpool(x)
x = self.layer1(x)
x = self.layer2(x)
x = self.layer3(x)
x = self.layer4(x)
#x = self.avgpool(x)
x = x.view(x.size(0), -1)
logits = self.fc(x)
probas = F.softmax(logits, dim=1)
return logits, probas
def resnet101(num_classes, grayscale):
"""Constructs a ResNet-101 model."""
model = ResNet(block=Bottleneck,
layers=[3, 4, 23, 3],
num_classes=NUM_CLASSES,
grayscale=grayscale)
return model
论文链接:https://arxiv.org/abs/1603.05027
代码地址:https://github.com/KaimingHe/resnet-1k-layers.
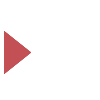
import torch.nn as nn
__all__ = ['preresnet20', 'preresnet32', 'preresnet44',
'preresnet56', 'preresnet110', 'preresnet1202']
def conv3x3(in_planes, out_planes, stride=1):
"3x3 convolution with padding"
return nn.Conv2d(in_planes, out_planes, kernel_size=3, stride=stride,
padding=1, bias=False)
class BasicBlock(nn.Module):
expansion = 1
def __init__(self, inplanes, planes, stride=1, downsample=None):
super(BasicBlock, self).__init__()
self.bn_1 = nn.BatchNorm2d(inplanes)
self.relu = nn.ReLU(inplace=True)
self.conv_1 = conv3x3(inplanes, planes, stride)
self.bn_2 = nn.BatchNorm2d(planes)
self.conv_2 = conv3x3(planes, planes)
self.downsample = downsample
self.stride = stride
def forward(self, x):
residual = x
out = self.bn_1(x)
out = self.relu(out)
out = self.conv_1(out)
out = self.bn_2(out)
out = self.relu(out)
out = self.conv_2(out)
if self.downsample is not None:
residual = self.downsample(x)
out += residual
return out
class Bottleneck(nn.Module):
expansion = 4
def __init__(self, inplanes, planes, stride=1, downsample=None):
super(Bottleneck, self).__init__()
self.bn_1 = nn.BatchNorm2d(inplanes)
self.conv_1 = nn.Conv2d(inplanes, planes, kernel_size=1, bias=False)
self.bn_2 = nn.BatchNorm2d(planes)
self.conv_2 = nn.Conv2d(planes, planes, kernel_size=3, stride=stride,
padding=1, bias=False)
self.bn_3 = nn.BatchNorm2d(planes)
self.conv_3 = nn.Conv2d(planes, planes * 4, kernel_size=1, bias=False)
self.relu = nn.ReLU(inplace=True)
self.downsample = downsample
self.stride = stride
def forward(self, x):
residual = x
out = self.bn_1(x)
out = self.relu(out)
out = self.conv_1(out)
out = self.bn_2(out)
out = self.relu(out)
out = self.conv_2(out)
out = self.bn_3(out)
out = self.relu(out)
out = self.conv_3(out)
if self.downsample is not None:
residual = self.downsample(x)
out += residual
return out
class PreResNet(nn.Module):
def __init__(self, depth, num_classes=1000, block_name='BasicBlock'):
super(PreResNet, self).__init__()
# Model type specifies number of layers for CIFAR-10 model
if block_name.lower() == 'basicblock':
assert (
depth - 2) % 6 == 0, "When use basicblock, depth should be 6n+2, e.g. 20, 32, 44, 56, 110, 1202"
n = (depth - 2) // 6
block = BasicBlock
elif block_name.lower() == 'bottleneck':
assert (
depth - 2) % 9 == 0, "When use bottleneck, depth should be 9n+2 e.g. 20, 29, 47, 56, 110, 1199"
n = (depth - 2) // 9
block = Bottleneck
else:
raise ValueError('block_name shoule be Basicblock or Bottleneck')
self.inplanes = 16
self.conv_1 = nn.Conv2d(3, 16, kernel_size=3, padding=1,
bias=False)
self.layer1 = self._make_layer(block, 16, n)
self.layer2 = self._make_layer(block, 32, n, stride=2)
self.layer3 = self._make_layer(block, 64, n, stride=2)
self.bn = nn.BatchNorm2d(64 * block.expansion)
self.relu = nn.ReLU(inplace=True)
self.avgpool = nn.AvgPool2d(8)
self.fc = nn.Linear(64 * block.expansion, num_classes)
for m in self.modules():
if isinstance(m, nn.Conv2d):
nn.init.kaiming_normal_(m.weight.data)
elif isinstance(m, nn.BatchNorm2d):
m.weight.data.fill_(1)
m.bias.data.zero_()
def _make_layer(self, block, planes, blocks, stride=1):
downsample = None
if stride != 1 or self.inplanes != planes * block.expansion:
downsample = nn.Sequential(
nn.Conv2d(self.inplanes, planes * block.expansion,
kernel_size=1, stride=stride, bias=False))
layers = []
layers.append(block(self.inplanes, planes, stride, downsample))
self.inplanes = planes * block.expansion
for _ in range(1, blocks):
layers.append(block(self.inplanes, planes))
return nn.Sequential(*layers)
def forward(self, x):
x = self.conv_1(x) # 32x32
x = self.layer1(x) # 32x32
x = self.layer2(x) # 16x16
x = self.layer3(x) # 8x8
x = self.bn(x)
x = self.relu(x)
x = self.avgpool(x)
x = x.view(x.size(0), -1)
x = self.fc(x)
return x
def preresnet20(num_classes):
return PreResNet(depth=20, num_classes=num_classes)
def preresnet32(num_classes):
return PreResNet(depth=32, num_classes=num_classes)
def preresnet44(num_classes):
return PreResNet(depth=44, num_classes=num_classes)
def preresnet56(num_classes):
return PreResNet(depth=56, num_classes=num_classes)
def preresnet110(num_classes):
return PreResNet(depth=110, num_classes=num_classes)
def preresnet1202(num_classes):
return PreResNet(depth=1202, num_classes=num_classes)
论文链接:https://arxiv.org/abs/1611.05431
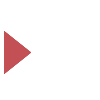
ResNeXt的关键点是:
沿用ResNet的短路连接,并且重复堆叠相同的模块组合。
ResNeXt将ResNet中非跳跃连接的那一分支变为多个分支。
多分支分别处理。
使用1×1卷积降低计算量。其综合了ResNet和Inception的优点。
ResNeXt与Inception最本质的差别,其实是Block内每个分支的拓扑结构,Inception为了提高表达能力/结合不同感受野,每个分支使用了不同的拓扑结构。而ResNeXt则使用了同一拓扑的分支,即ResNeXt的分支是同构的!
因为ResNeXt是同构的,因此继承了VGG/ResNet的精神衣钵:维持网络拓扑结构不变。主要体现在两点:
特征图大小相同,则涉及的结构超参数相同
每当空间分辨率/2(降采样),则卷积核的宽度*2
神经元连接
聚合变换
ResNeXt最终输出模块公式:
此外,ResNeXt巧妙地利用分组卷积进行实现。ResNeXt发现,增加分支数是比加深或加宽更有效地提升网络性能的方式。ResNeXt的命名旨在说明这是下一代(next)的ResNet。
如果一个ResNeXt Block中只有两层conv,前后都可等效成一个大的conv层
上图a的解读:
ResNeXt最核心的地方只存在于被最上最下两层卷积夹着的,中间的部分。
因为第一个分开的conv其实都接受了一样的输入,各分支又有着相同的拓扑结构。类比乘法结合律,这其实就是把一个conv的输出拆开了分掉。(相同输入,不同输出)
而最后一个conv又只对同一个输出负责,因此就可以并起来用一个conv处理。(不同输入,相同输出)
唯一一个输入和输出都不同的,就是中间的3*3conv了。它们的输入,参数,负责的输出都不同,无法合并,因此也相互独立。这才是模型的关键所在。最终模型可以被等效为下图所示的最终形态:
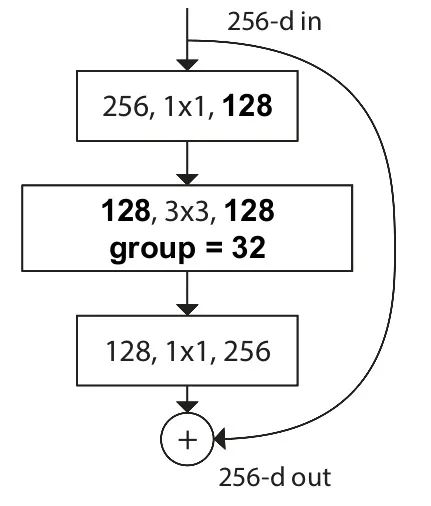
ResNeXt的网络结构设计:
import torch.nn as nn
import torch.nn.functional as F
__all__ = ['resnext29_8x64d', 'resnext29_16x64d']
class Bottleneck(nn.Module):
def __init__(
self,
in_channels,
out_channels,
stride,
cardinality,
base_width,
expansion):
super(Bottleneck, self).__init__()
width_ratio = out_channels / (expansion * 64.)
D = cardinality * int(base_width * width_ratio)
self.relu = nn.ReLU(inplace=True)
self.conv_reduce = nn.Conv2d(
in_channels, D, kernel_size=1, stride=1, padding=0, bias=False)
self.bn_reduce = nn.BatchNorm2d(D)
self.conv_conv = nn.Conv2d(
D,
D,
kernel_size=3,
stride=stride,
padding=1,
groups=cardinality,
bias=False)
self.bn = nn.BatchNorm2d(D)
self.conv_expand = nn.Conv2d(
D, out_channels, kernel_size=1, stride=1, padding=0, bias=False)
self.bn_expand = nn.BatchNorm2d(out_channels)
self.shortcut = nn.Sequential()
if in_channels != out_channels:
self.shortcut.add_module(
'shortcut_conv',
nn.Conv2d(
in_channels,
out_channels,
kernel_size=1,
stride=stride,
padding=0,
bias=False))
self.shortcut.add_module(
'shortcut_bn', nn.BatchNorm2d(out_channels))
def forward(self, x):
out = self.conv_reduce.forward(x)
out = self.relu(self.bn_reduce.forward(out))
out = self.conv_conv.forward(out)
out = self.relu(self.bn.forward(out))
out = self.conv_expand.forward(out)
out = self.bn_expand.forward(out)
residual = self.shortcut.forward(x)
return self.relu(residual + out)
class ResNeXt(nn.Module):
"""
ResNext optimized for the Cifar dataset, as specified in
https://arxiv.org/pdf/1611.05431.pdf
"""
def __init__(
self,
cardinality,
depth,
num_classes,
base_width,
expansion=4):
""" Constructor
Args:
cardinality: number of convolution groups.
depth: number of layers.
num_classes: number of classes
base_width: base number of channels in each group.
expansion: factor to adjust the channel dimensionality
"""
super(ResNeXt, self).__init__()
self.cardinality = cardinality
self.depth = depth
self.block_depth = (self.depth - 2) // 9
self.base_width = base_width
self.expansion = expansion
self.num_classes = num_classes
self.output_size = 64
self.stages = [64, 64 * self.expansion, 128 *
self.expansion, 256 * self.expansion]
self.conv_1_3x3 = nn.Conv2d(3, 64, 3, 1, 1, bias=False)
self.bn_1 = nn.BatchNorm2d(64)
self.stage_1 = self.block('stage_1', self.stages[0], self.stages[1], 1)
self.stage_2 = self.block('stage_2', self.stages[1], self.stages[2], 2)
self.stage_3 = self.block('stage_3', self.stages[2], self.stages[3], 2)
self.fc = nn.Linear(self.stages[3], num_classes)
for m in self.modules():
if isinstance(m, nn.Conv2d):
nn.init.kaiming_normal_(m.weight.data)
elif isinstance(m, nn.BatchNorm2d):
m.weight.data.fill_(1)
m.bias.data.zero_()
def block(self, name, in_channels, out_channels, pool_stride=2):
block = nn.Sequential()
for bottleneck in range(self.block_depth):
name_ = '%s_bottleneck_%d' % (name, bottleneck)
if bottleneck == 0:
block.add_module(
name_,
Bottleneck(
in_channels,
out_channels,
pool_stride,
self.cardinality,
self.base_width,
self.expansion))
else:
block.add_module(
name_,
Bottleneck(
out_channels,
out_channels,
1,
self.cardinality,
self.base_width,
self.expansion))
return block
def forward(self, x):
x = self.conv_1_3x3.forward(x)
x = F.relu(self.bn_1.forward(x), inplace=True)
x = self.stage_1.forward(x)
x = self.stage_2.forward(x)
x = self.stage_3.forward(x)
x = F.avg_pool2d(x, 8, 1)
x = x.view(-1, self.stages[3])
return self.fc(x)
def resnext29_8x64d(num_classes):
return ResNeXt(
cardinality=8,
depth=29,
num_classes=num_classes,
base_width=64)
def resnext29_16x64d(num_classes):
return ResNeXt(
cardinality=16,
depth=29,
num_classes=num_classes,
base_width=64)
https://zhuanlan.zhihu.com/p/54289848
https://zhuanlan.zhihu.com/p/28124810
https://zhuanlan.zhihu.com/p/31727402
https://zhuanlan.zhihu.com/p/56961832
https://zhuanlan.zhihu.com/p/54072011
https://github.com/BIGBALLON/CIFAR-ZOO
https://zhuanlan.zhihu.com/p/78019001
交流群
欢迎加入公众号读者群一起和同行交流,目前有SLAM、三维视觉、传感器、自动驾驶、计算摄影、检测、分割、识别、医学影像、GAN、算法竞赛等微信群(以后会逐渐细分),请扫描下面微信号加群,备注:”昵称+学校/公司+研究方向“,例如:”张三 + 上海交大 + 视觉SLAM“。请按照格式备注,否则不予通过。添加成功后会根据研究方向邀请进入相关微信群。请勿在群内发送广告,否则会请出群,谢谢理解~