Yolov5总结文档(理论、代码、实验结果)
一、Yolo-v5结构
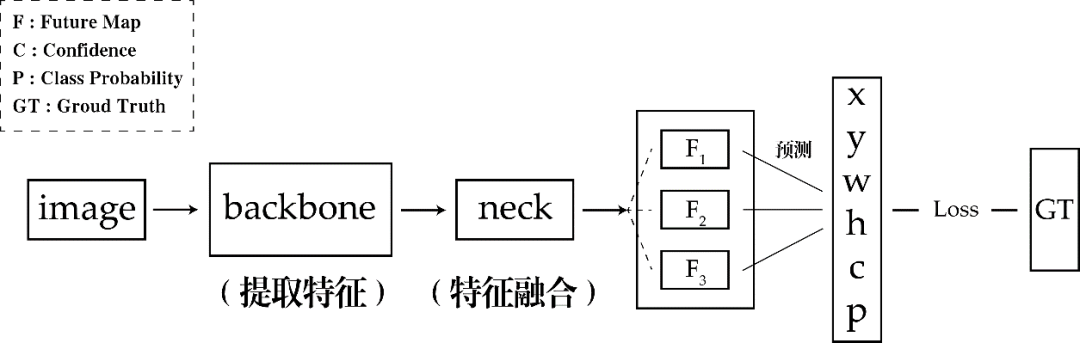
1.输入
2.Backbone(以Yolov5s为例)
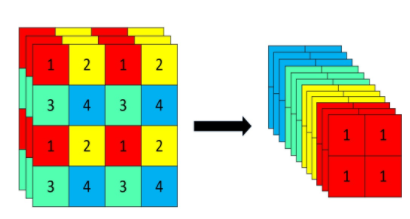
3.Neck
4.Loss
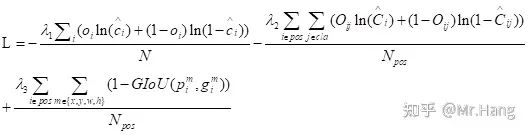
二、修改Backbone
# parameters
nc: 20 # number of classes
depth_multiple: 0.33 # model depth multiple
width_multiple: 0.50 # layer channel multiple
backbone:
# [from, number, module, args]
[[-1, 1, Focus, [64, 3]], # 0-P1/2
[-1, 1, Conv, [128, 3, 2]], # 1-P2/4
[-1, 3, C3, [128]],
[-1, 1, Conv, [256, 3, 2]], # 3-P3/8
[-1, 9, C3, [256]],
[-1, 1, Conv, [512, 3, 2]], # 5-P4/16
[-1, 9, C3, [512]],
[-1, 1, Conv, [1024, 3, 2]], # 7-P5/32
[-1, 1, SPP, [1024, [5, 9, 13]]],
[-1, 3, C3, [1024, False]], # 9
]
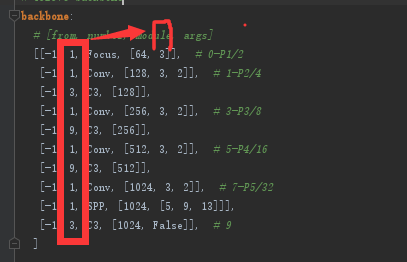
class h_sigmoid(nn.Module):
def __init__(self, inplace=True):
super(h_sigmoid, self).__init__()
self.relu = nn.ReLU6(inplace=inplace)
def forward(self, x):
return self.relu(x + 3) / 6
class h_swish(nn.Module):
def __init__(self, inplace=True):
super(h_swish, self).__init__()
self.sigmoid = h_sigmoid(inplace=inplace)
def forward(self, x):
y = self.sigmoid(x)
return x * y
class SELayer(nn.Module):
def __init__(self, channel, reduction=4):
super(SELayer, self).__init__()
self.avg_pool = nn.AdaptiveAvgPool2d(1)
self.fc = nn.Sequential(
nn.Linear(channel, channel // reduction),
nn.ReLU(inplace=True),
nn.Linear(channel // reduction, channel),
h_sigmoid()
)
def forward(self, x):
b, c, _, _ = x.size()
y = self.avg_pool(x)
y = y.view(b, c)
y = self.fc(y).view(b, c, 1, 1)
return x * y
class conv_bn_hswish(nn.Module):
def __init__(self, c1, c2, stride):
super(conv_bn_hswish, self).__init__()
self.conv = nn.Conv2d(c1, c2, 3, stride, 1, bias=False)
self.bn = nn.BatchNorm2d(c2)
self.act = h_swish()
def forward(self, x):
return self.act(self.bn(self.conv(x)))
def fuseforward(self, x):
return self.act(self.conv(x))
class MobileNetV3_InvertedResidual(nn.Module):
def __init__(self, inp, oup, hidden_dim, kernel_size, stride, use_se, use_hs):
super(MobileNetV3_InvertedResidual, self).__init__()
assert stride in [1, 2]
self.identity = stride == 1 and inp == oup
if inp == hidden_dim:
self.conv = nn.Sequential(
# dw
nn.Conv2d(hidden_dim, hidden_dim, kernel_size, stride, (kernel_size - 1) // 2, groups=hidden_dim, bias=False),
nn.BatchNorm2d(hidden_dim),
h_swish() if use_hs else nn.ReLU(inplace=True),
# Squeeze-and-Excite
SELayer(hidden_dim) if use_se else nn.Sequential(),
# pw-linear
nn.Conv2d(hidden_dim, oup, 1, 1, 0, bias=False),
nn.BatchNorm2d(oup),
)
else:
self.conv = nn.Sequential(
# pw
nn.Conv2d(inp, hidden_dim, 1, 1, 0, bias=False),
nn.BatchNorm2d(hidden_dim),
h_swish() if use_hs else nn.ReLU(inplace=True),
# dw
nn.Conv2d(hidden_dim, hidden_dim, kernel_size, stride, (kernel_size - 1) // 2, groups=hidden_dim, bias=False),
nn.BatchNorm2d(hidden_dim),
# Squeeze-and-Excite
SELayer(hidden_dim) if use_se else nn.Sequential(),
h_swish() if use_hs else nn.ReLU(inplace=True),
# pw-linear
nn.Conv2d(hidden_dim, oup, 1, 1, 0, bias=False),
nn.BatchNorm2d(oup),
)
def forward(self, x):
y = self.conv(x)
if self.identity:
return x + y
else:
return y
nc: 20 # number of classes
depth_multiple: 0.33
width_multiple: 0.50
# anchors
anchors:
- [10,13, 16,30, 33,23] # P3/8
- [30,61, 62,45, 59,119] # P4/16
- [116,90, 156,198, 373,326] # P5/32
# custom backbone
backbone:
# MobileNetV3-small
# [from, number, module, args]
[[-1, 1, conv_bn_hswish, [16, 2]], # 0-p1/2
[-1, 1, MobileNetV3_InvertedResidual, [16, 16, 3, 2, 1, 0]], # 1-p2/4
[-1, 1, MobileNetV3_InvertedResidual, [24, 72, 3, 2, 0, 0]], # 2-p3/8
[-1, 1, MobileNetV3_InvertedResidual, [24, 88, 3, 1, 0, 0]], # 3-p3/8
[-1, 1, MobileNetV3_InvertedResidual, [40, 96, 5, 2, 1, 1]], # 4-p4/16
[-1, 1, MobileNetV3_InvertedResidual, [40, 240, 5, 1, 1, 1]], # 5-p4/16
[-1, 1, MobileNetV3_InvertedResidual, [40, 240, 5, 1, 1, 1]], # 6-p4/16
[-1, 1, MobileNetV3_InvertedResidual, [48, 120, 5, 1, 1, 1]], # 7-p4/16
[-1, 1, MobileNetV3_InvertedResidual, [48, 144, 5, 1, 1, 1]], # 8-p4/16
[-1, 1, MobileNetV3_InvertedResidual, [96, 288, 5, 2, 1, 1]], # 9-p5/32
[-1, 1, MobileNetV3_InvertedResidual, [96, 576, 5, 1, 1, 1]], # 10-p5/32
[-1, 1, MobileNetV3_InvertedResidual, [96, 576, 5, 1, 1, 1]], # 11-p5/32
]
head:
[[-1, 1, Conv, [256, 1, 1]],
[-1, 1, nn.Upsample, [None, 2, 'nearest']],
[[-1, 8], 1, Concat, [1]], # cat backbone P4
[-1, 1, C3, [256, False]], # 15
[-1, 1, Conv, [128, 1, 1]],
[-1, 1, nn.Upsample, [None, 2, 'nearest']],
[[-1, 3], 1, Concat, [1]], # cat backbone P3
[-1, 1, C3, [128, False]], # 19 (P3/8-small)
[-1, 1, Conv, [128, 3, 2]],
[[-1, 16], 1, Concat, [1]], # cat head P4
[-1, 1, C3, [256, False]], # 22 (P4/16-medium)
[-1, 1, Conv, [256, 3, 2]],
[[-1, 12], 1, Concat, [1]], # cat head P5
[-1, 1, C3, [512, False]], # 25 (P5/32-large)
[[19, 22, 25], 1, Detect, [nc, anchors]], # Detect(P3, P4, P5)
]
三、实验结果
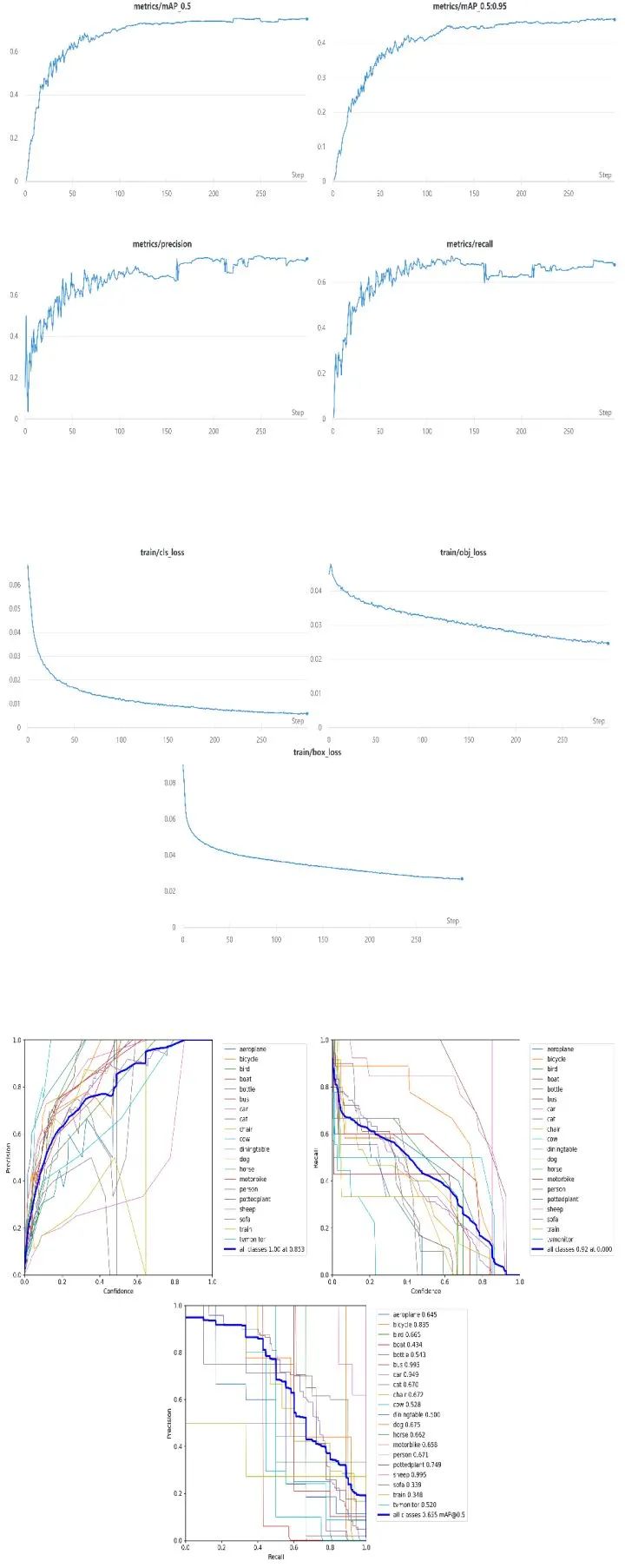
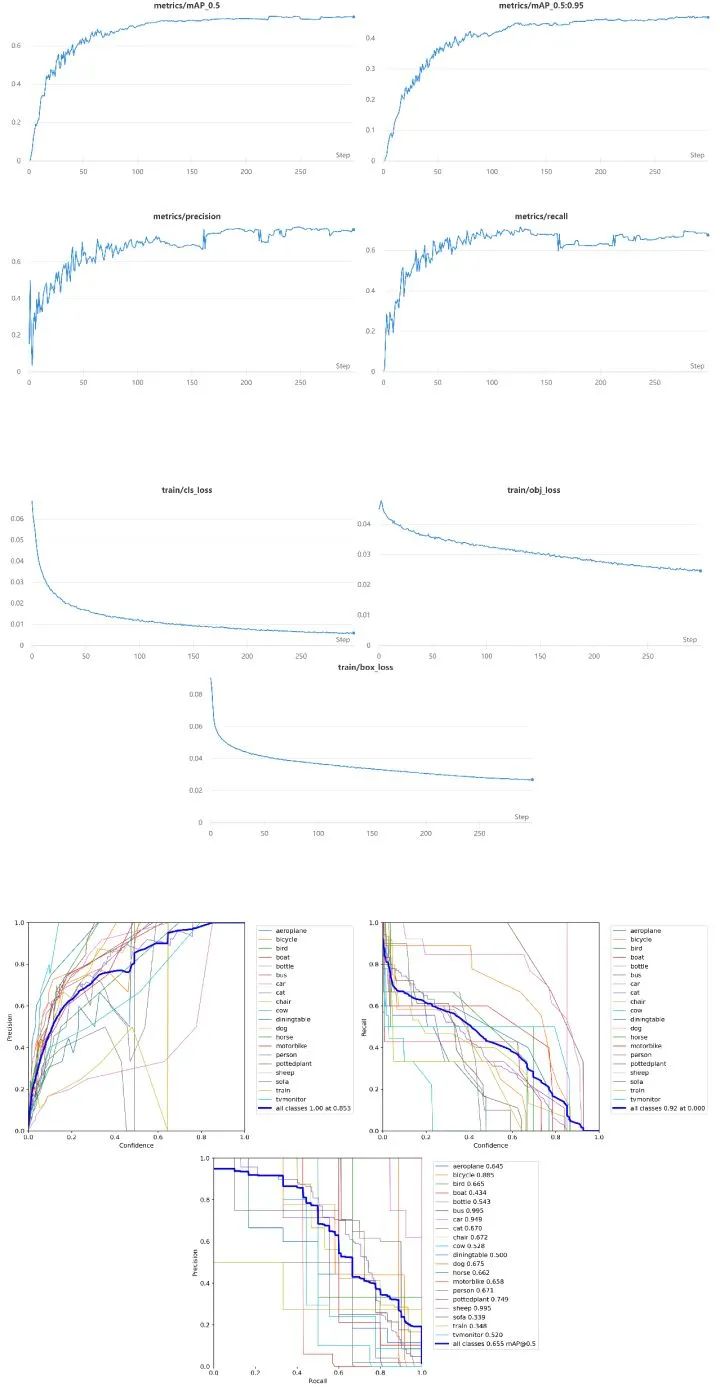
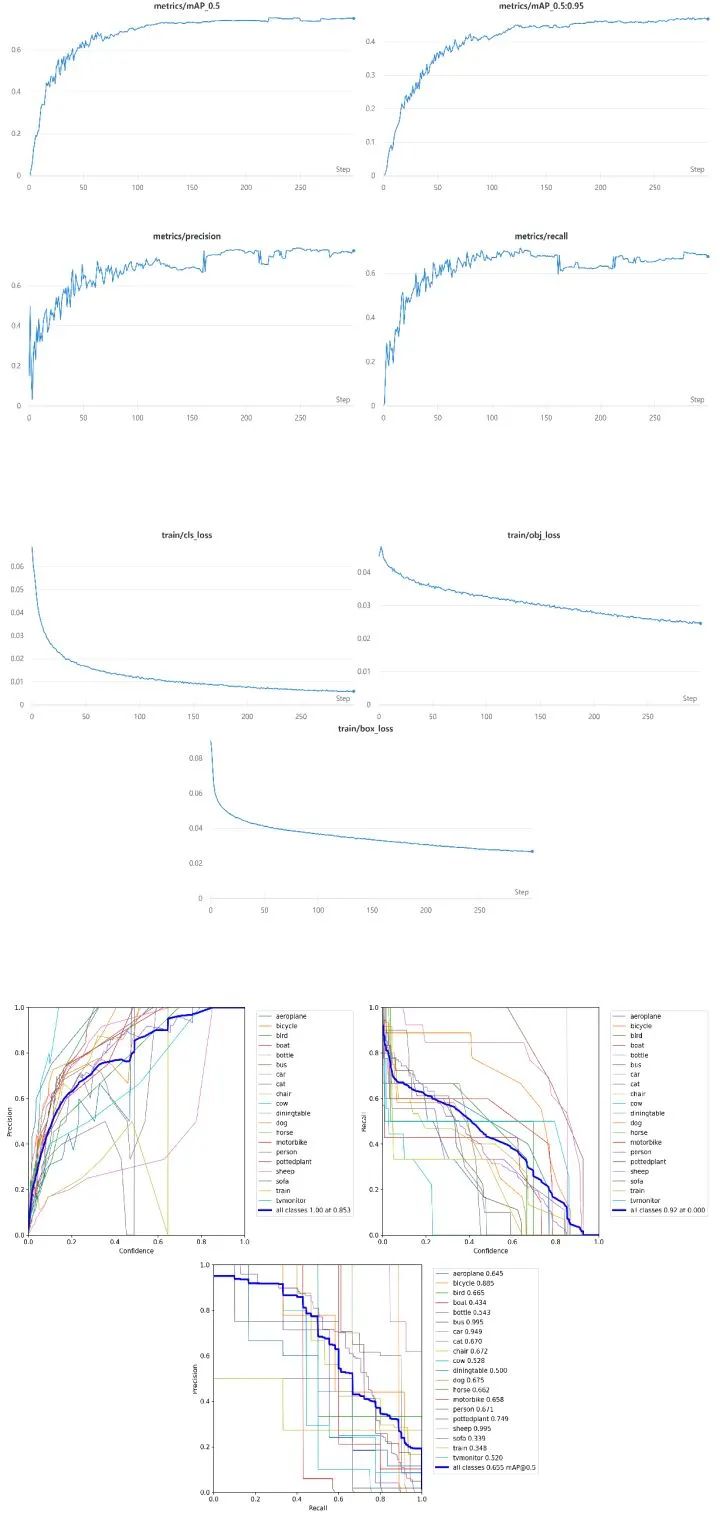
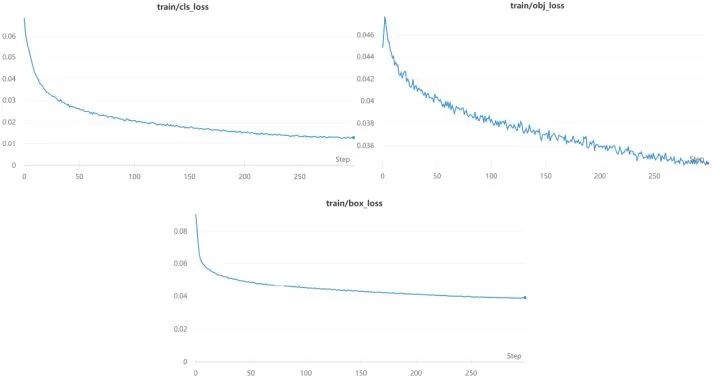
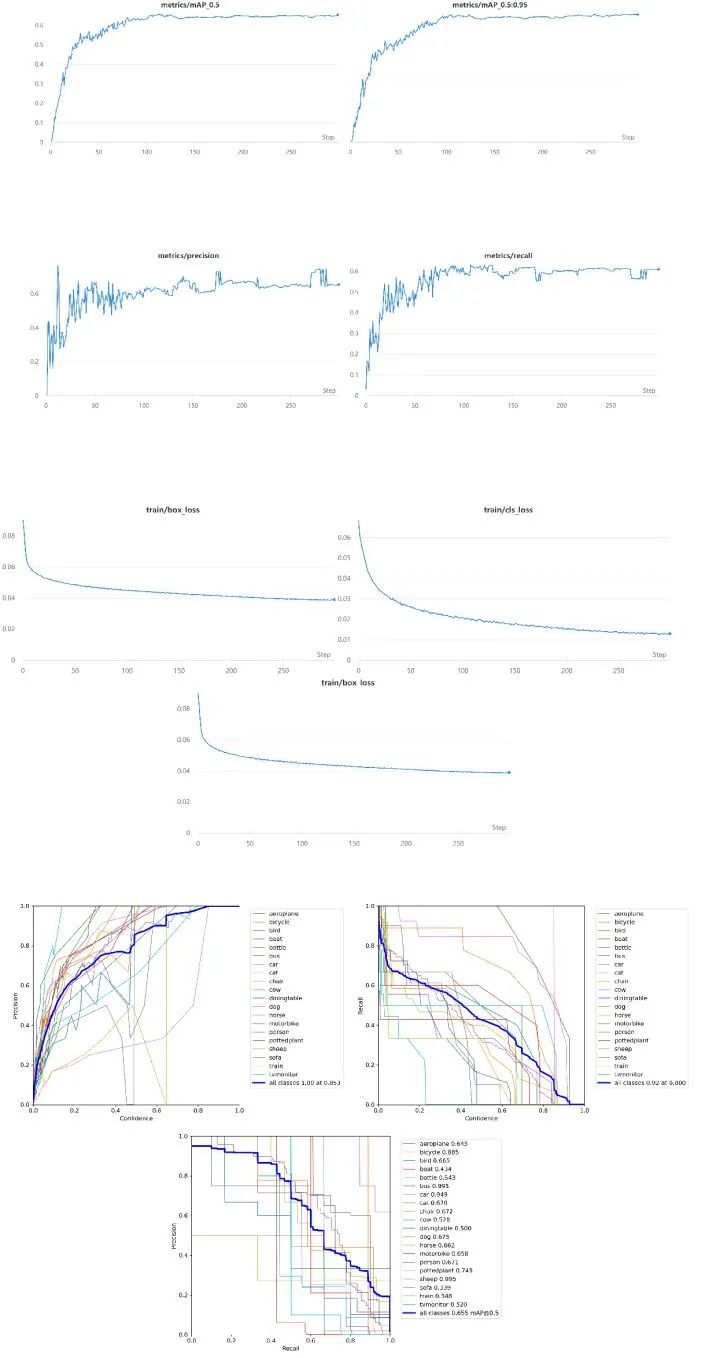
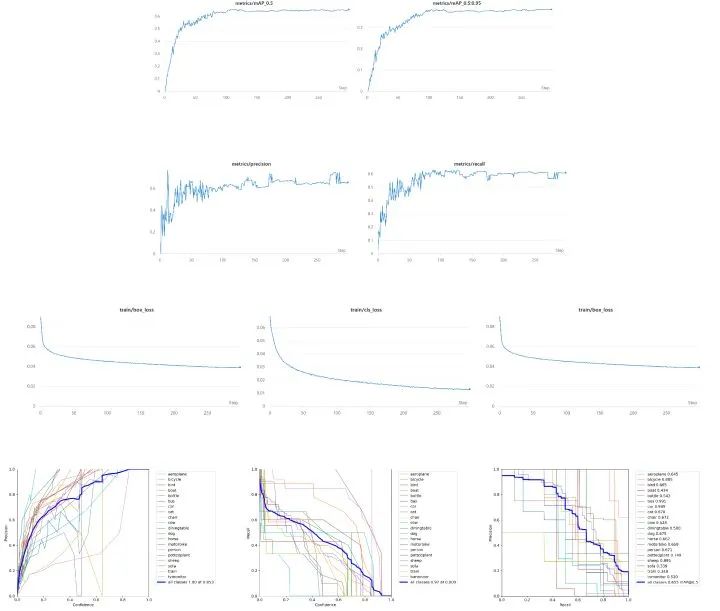
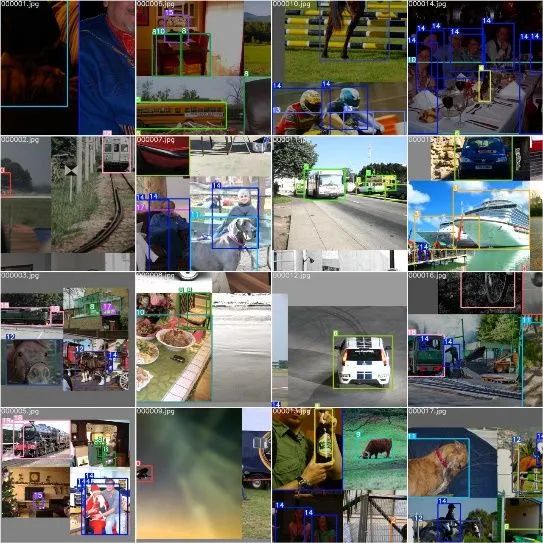
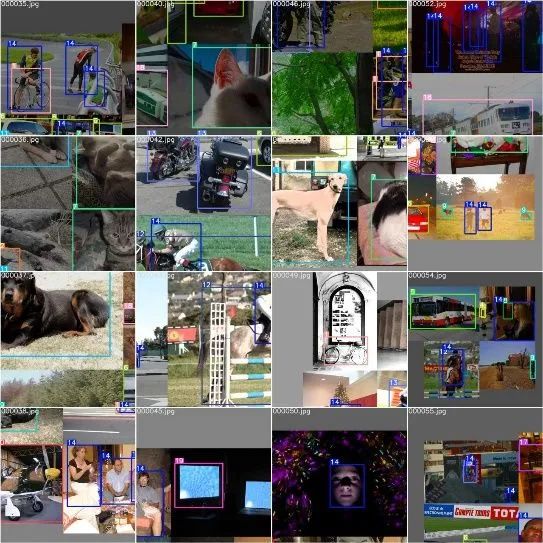
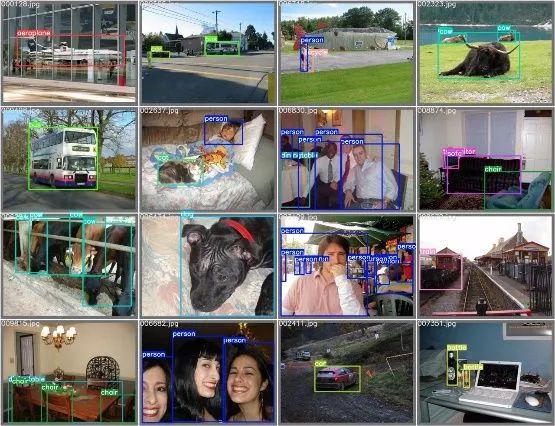
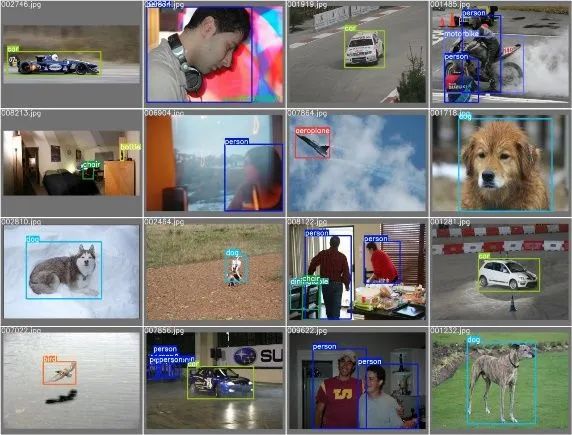
四、总结
评论