一个非常好用的 Python 魔法库【文末查看上周中奖者】
Python 知识大全
共 5172字,需浏览 11分钟
·
2020-11-02 06:21
点击上方“Python 知识大全”,选择“加为星标”
第一时间关注Python技术干货!
【导语】:还在为日常工作中不同的数据集的字段进行匹配烦恼?今天跟大家分享FuzzyWuzzy一个简单易用的模糊字符串匹配工具包。让你多快好省的解决烦恼的匹配问题!
1. 前言
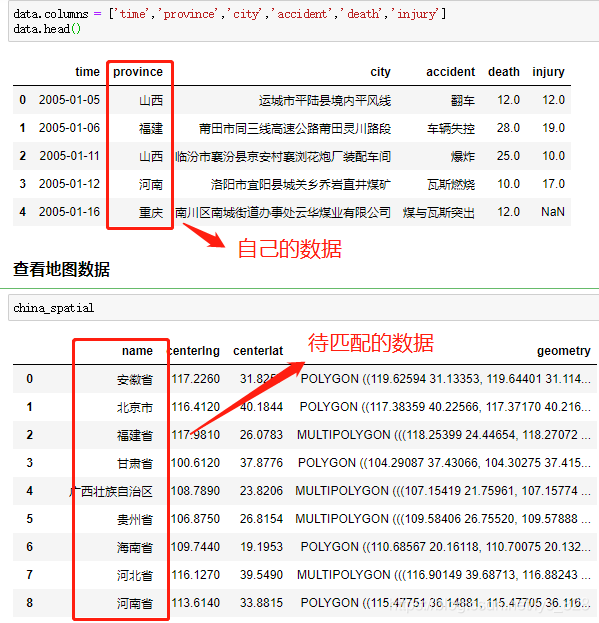
2. FuzzyWuzzy库介绍
pip install -i https://pypi.tuna.tsinghua.edu.cn/simple FuzzyWuzzy
2.1 fuzz模块

2.1.1 简单匹配(Ratio)
fuzz.ratio("河南省", "河南省")
>>> 100
>
fuzz.ratio("河南", "河南省")
>>> 80
2.1.2 非完全匹配(Partial Ratio)
fuzz.partial_ratio("河南省", "河南省")
>>> 100
fuzz.partial_ratio("河南", "河南省")
>>> 100
2.1.3 忽略顺序匹配(Token Sort Ratio)
fuzz.ratio("西藏 自治区", "自治区 西藏")
>>> 50
fuzz.ratio('I love YOU','YOU LOVE I')
>>> 30
fuzz.token_sort_ratio("西藏 自治区", "自治区 西藏")
>>> 100
fuzz.token_sort_ratio('I love YOU','YOU LOVE I')
>>> 100
2.1.4 去重子集匹配(Token Set Ratio)
fuzz.ratio("西藏 西藏 自治区", "自治区 西藏")
40 >
fuzz.token_sort_ratio("西藏 西藏 自治区", "自治区 西藏")
80 >
fuzz.token_set_ratio("西藏 西藏 自治区", "自治区 西藏")
100 >
2.2 process模块
2.2.1 extract提取多条数据
choices = ["河南省", "郑州市", "湖北省", "武汉市"]
process.extract("郑州", choices, limit=2)
'郑州市', 90), ('河南省', 0)] > [(
# extract之后的数据类型是列表,即使limit=1,最后还是列表,注意和下面extractOne的区别
2.2.2 extractOne提取一条数据
process.extractOne("郑州", choices)
>>> ('郑州市', 90)
process.extractOne("北京", choices)
>>> ('湖北省', 45)
3. 实战应用
3.1 公司名称字段模糊匹配
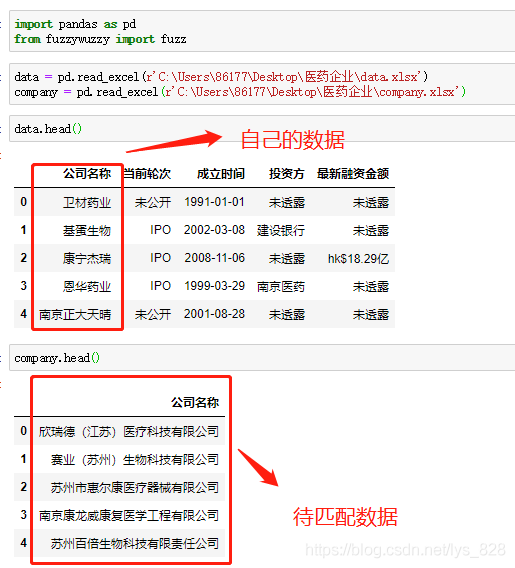
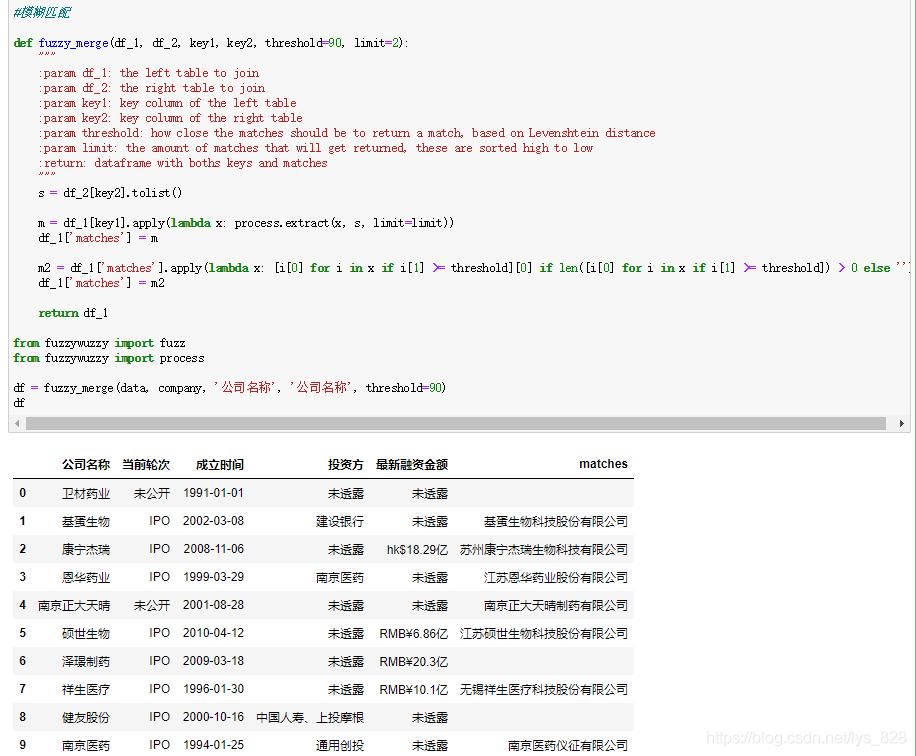
3.1.1 参数讲解:
3.1.2 核心代码讲解
s = df_2[key2].tolist()
m = df_1[key1].apply(lambda x: process.extract(x, s, limit=limit))
df_1['matches'] = m
m2 = df_1['matches'].apply(lambda x: [i[0] for i in x if i[1] >= threshold][0] if len([i[0] for i in x if i[1] >= threshold]) > 0 else '')
#要理解第一个‘matches’字段返回的数据类型是什么样子的,就不难理解这行代码了
#参考一下这个格式:[('郑州市', 90), ('河南省', 0)]
df_1['matches'] = m2
return df_1
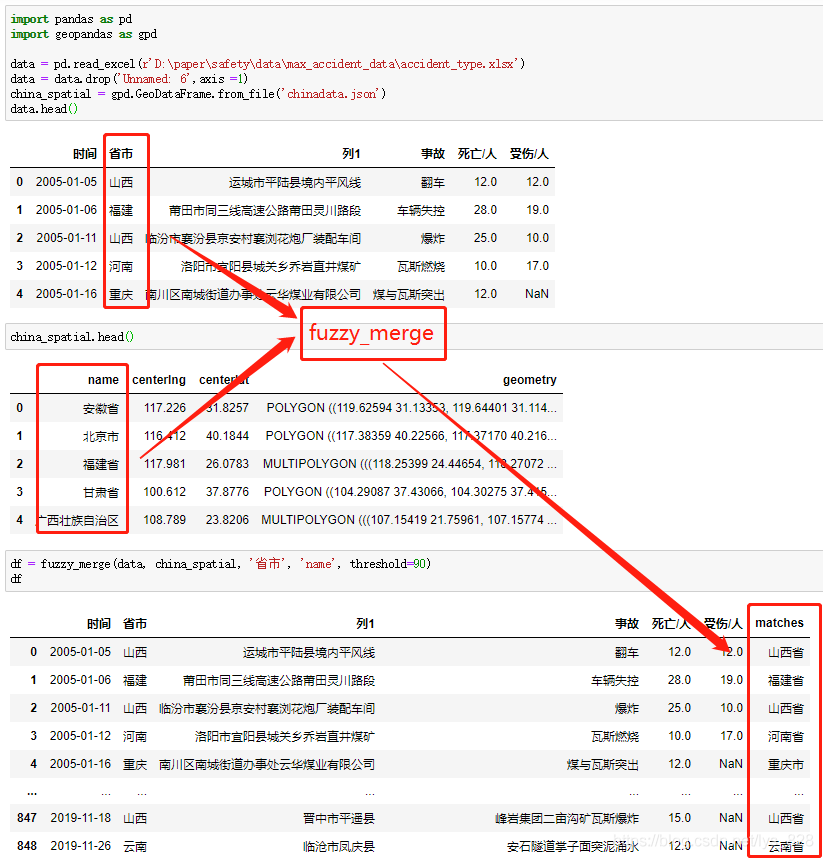
4. 全部函数代码
#模糊匹配
def fuzzy_merge(df_1, df_2, key1, key2, threshold=90, limit=2):
"""
:param df_1: the left table to join
:param df_2: the right table to join
:param key1: key column of the left table
:param key2: key column of the right table
:param threshold: how close the matches should be to return a match, based on Levenshtein distance
:param limit: the amount of matches that will get returned, these are sorted high to low
:return: dataframe with boths keys and matches
"""
s = df_2[key2].tolist()
m = df_1[key1].apply(lambda x: process.extract(x, s, limit=limit))
df_1['matches'] = m
m2 = df_1['matches'].apply(lambda x: [i[0] for i in x if i[1] >= threshold][0] if len([i[0] for i in x if i[1] >= threshold]) > 0 else '')
df_1['matches'] = m2
return df_1
from fuzzywuzzy import fuzz
from fuzzywuzzy import process
df = fuzzy_merge(data, company, '公司名称', '公司名称', threshold=90)
df
文末福利
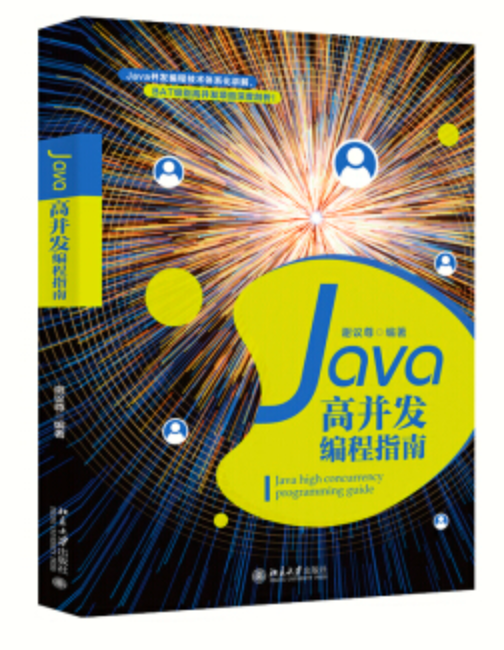
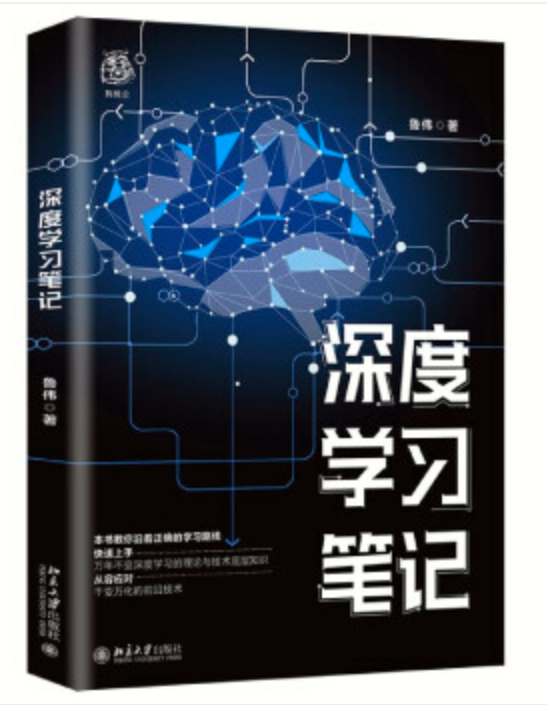
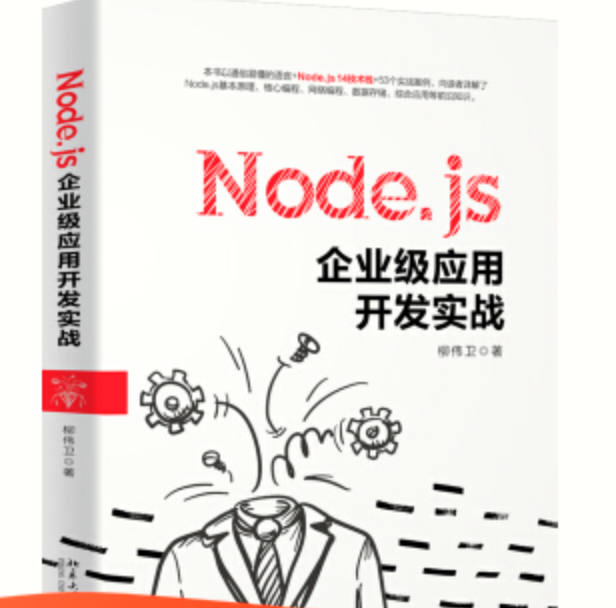
这次送书规则很简单,【除号主之外】规则如下:
最近阅读前十名中的第一名 and 最近分享前十名的第一名截图如下 【数据来自订阅号助手 App】 请 WGL 网网 和 Charline 在公众号回复关键字【微信】联系我。三天后无效!
关注「Python 知识大全」,做全栈开发工程师 岁月有你 惜惜相处 回复 【资料】获取高质量学习资料 好文章,我在看❤️
评论