一文详解ORB-SLAM3中的位姿跟踪Tracking类实现
新机器视觉
共 14323字,需浏览 29分钟
·
2021-04-13 13:23
点击上方“新机器视觉”,选择加"星标"或“置顶”
重磅干货,第一时间送达
1、Tracking构造函数
{
// Load camera parameters from settings file
cv::FileStorage fSettings(strSettingPath, cv::FileStorage::READ);//只读
//读取相机参数
//如果是ROS,DepthMapFactor应该设为1,即深度不进行缩放
bool b_parse_cam = ParseCamParamFile(fSettings);
if(!b_parse_cam)
{
std::cout << "*Error with the camera parameters in the config file*" << std::endl;
}
// Load ORB parameters
//读取ORB特征提取的相关参数,该函数中还会
//根据参数构造ORB提取器mpORBextractorLeft(左目)、mpORBextractorRight(右目)、mpIniORBextractor(初始化用)
bool b_parse_orb = ParseORBParamFile(fSettings);
if(!b_parse_orb)
{
std::cout << "*Error with the ORB parameters in the config file*" << std::endl;
}
initID = 0; lastID = 0;
// Load IMU parameters
bool b_parse_imu = true;
if(sensor==System::IMU_MONOCULAR || sensor==System::IMU_STEREO)
{
//读取imu参数,该函数中还会
//根据参数构建预积分处理器mpImuPreintegratedFromLastKF
b_parse_imu = ParseIMUParamFile(fSettings);
if(!b_parse_imu)
{
std::cout << "*Error with the IMU parameters in the config file*" << std::endl;
}
mnFramesToResetIMU = mMaxFrames;
}
mbInitWith3KFs = false;
mnNumDataset = 0;
if(!b_parse_cam || !b_parse_orb || !b_parse_imu)
{
std::cerr << "**ERROR in the config file, the format is not correct**" << std::endl;
try
{
throw -1;
}
catch(exception &e)
{
}
}
#ifdef SAVE_TIMES
f_track_times.open("tracking_times.txt");
f_track_times << "# ORB_Ext(ms), Stereo matching(ms), Preintegrate_IMU(ms), Pose pred(ms), LocalMap_track(ms), NewKF_dec(ms)" << endl;
f_track_times << fixed ;
#endif
}
2、开始跟踪
cv::Mat Tracking::GrabImageMonocular(const cv::Mat &im, const double ×tamp, string filename)
{
mImGray = im;
//将彩色图像转为灰度图像
if(mImGray.channels()==3)
{
if(mbRGB)
cvtColor(mImGray,mImGray,CV_RGB2GRAY);
else
cvtColor(mImGray,mImGray,CV_BGR2GRAY);
}
else if(mImGray.channels()==4)
{
if(mbRGB)
cvtColor(mImGray,mImGray,CV_RGBA2GRAY);
else
cvtColor(mImGray,mImGray,CV_BGRA2GRAY);
}
//构造Frame,同时完成特征点的提取、计算词袋等操作
if (mSensor == System::MONOCULAR)
{
if(mState==NOT_INITIALIZED || mState==NO_IMAGES_YET ||(lastID - initID) < mMaxFrames)
//还未初始化,用mpIniORBextractor提取器,提取的特征点是正常的5倍
mCurrentFrame = Frame(mImGray,timestamp,mpIniORBextractor,mpORBVocabulary,mpCamera,mDistCoef,mbf,mThDepth);
else
mCurrentFrame = Frame(mImGray,timestamp,mpORBextractorLeft,mpORBVocabulary,mpCamera,mDistCoef,mbf,mThDepth);
}
//imu模式的Frame构造函数
else if(mSensor == System::IMU_MONOCULAR)
{
if(mState==NOT_INITIALIZED || mState==NO_IMAGES_YET)
{
mCurrentFrame = Frame(mImGray,timestamp,mpIniORBextractor,mpORBVocabulary,mpCamera,mDistCoef,mbf,mThDepth,&mLastFrame,*mpImuCalib);
}
else
mCurrentFrame = Frame(mImGray,timestamp,mpORBextractorLeft,mpORBVocabulary,mpCamera,mDistCoef,mbf,mThDepth,&mLastFrame,*mpImuCalib);
}
// t0存储未初始化时的第1帧时间戳
if (mState==NO_IMAGES_YET)
t0=timestamp;
std::chrono::steady_clock::time_point t0 = std::chrono::steady_clock::now();
mCurrentFrame.mNameFile = filename;
mCurrentFrame.mnDataset = mnNumDataset;
lastID = mCurrentFrame.mnId;
// 跟踪
Track();//最主要的函数
std::chrono::steady_clock::time_point t1 = std::chrono::steady_clock::now();
double t_track = std::chrono::duration_cast<std::chrono::duration<double,std::milli> >(t1 - t0).count();
//保存耗时
#ifdef SAVE_TIMES
f_track_times << mCurrentFrame.mTimeORB_Ext << ",";
f_track_times << mCurrentFrame.mTimeStereoMatch << ",";
f_track_times << mTime_PreIntIMU << ",";
f_track_times << mTime_PosePred << ",";
f_track_times << mTime_LocalMapTrack << ",";
f_track_times << mTime_NewKF_Dec << ",";
f_track_times << t_track << endl;
#endif
//返回当前帧的位姿
return mCurrentFrame.mTcw.clone();
}
3、Track( )函数
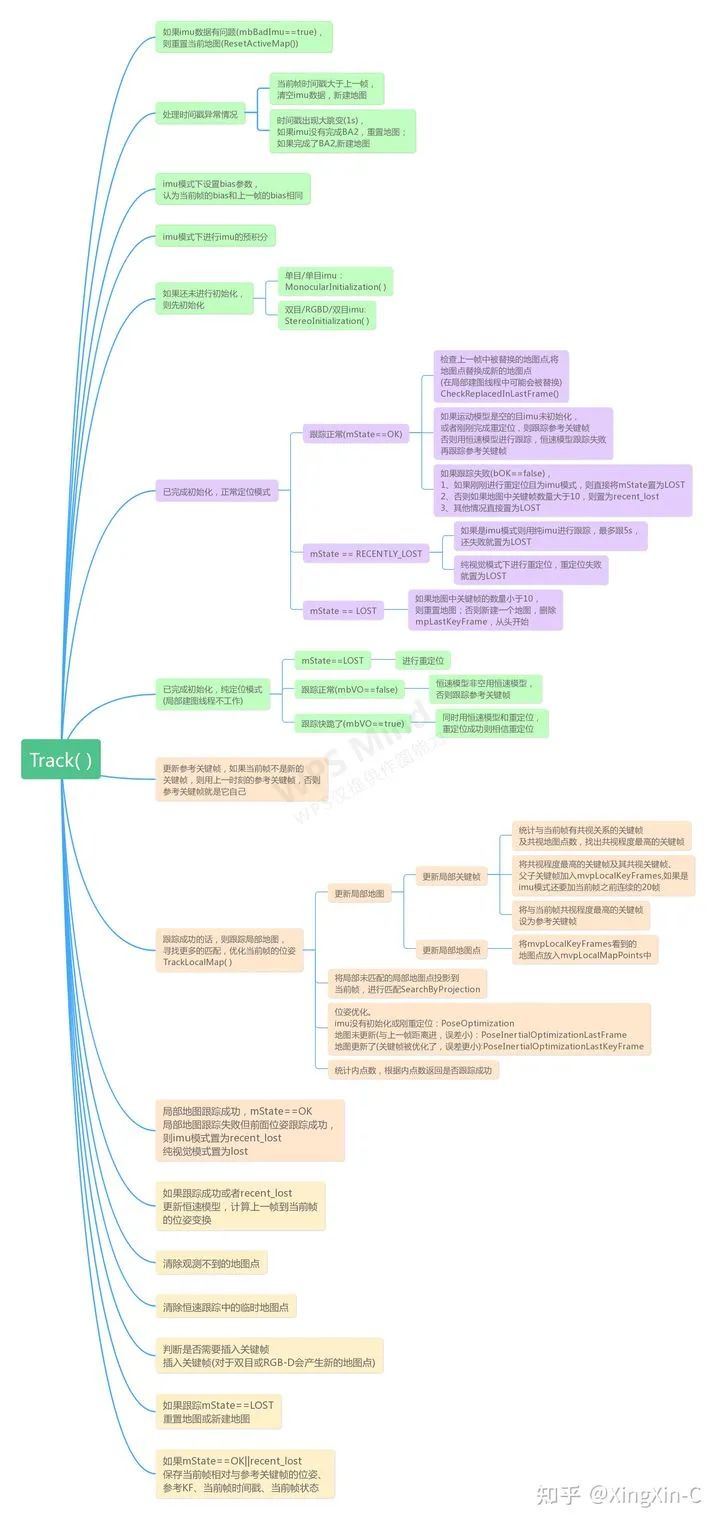
4、利用IMU计算位姿 PredictStateIMU()
5、预积分 PreintegrateIMU()
6、恒速模型跟踪 TrackWithMotionModel()
7、跟踪参考关键帧 TrackReferenceKeyFrame( )
bool Tracking::TrackReferenceKeyFrame()
{
// Compute Bag of Words vector
mCurrentFrame.ComputeBoW();//计算词袋向量mBowVec和特征向量mFeatVec
// We perform first an ORB matching with the reference keyframe
ORBmatcher matcher(0.7,true);
vector<MapPoint*> vpMapPointMatches;
//通过BoW加速匹配。用到了mFeatVec,两个关键帧中 只有节点相同的特征点才会被比较,相同节点中的特征点采用暴力搜索,并且需要检查方向性,
//并且最优的要明显好于次优的。
int nmatches = matcher.SearchByBoW(mpReferenceKF,mCurrentFrame,vpMapPointMatches);
if(nmatches<15)
{
cout << "TRACK_REF_KF: Less than 15 matches!!\n";
return false;
}
mCurrentFrame.mvpMapPoints = vpMapPointMatches;
mCurrentFrame.SetPose(mLastFrame.mTcw);//用上一帧的位姿作为初始值
//mCurrentFrame.PrintPointDistribution();
// cout << " TrackReferenceKeyFrame mLastFrame.mTcw: " << mLastFrame.mTcw << endl;
Optimizer::PoseOptimization(&mCurrentFrame);//位姿优化
// Discard outliers
int nmatchesMap = 0;
//删除外点,统计内点
for(int i =0; i<mCurrentFrame.N; i++)
{
//if(i >= mCurrentFrame.Nleft) break;
if(mCurrentFrame.mvpMapPoints[i])
{
if(mCurrentFrame.mvbOutlier[i])
{
MapPoint* pMP = mCurrentFrame.mvpMapPoints[i];
mCurrentFrame.mvpMapPoints[i]=static_cast<MapPoint*>(NULL);
mCurrentFrame.mvbOutlier[i]=false;
if(i < mCurrentFrame.Nleft){
pMP->mbTrackInView = false;
}
else{
pMP->mbTrackInViewR = false;
}
pMP->mbTrackInView = false;
pMP->mnLastFrameSeen = mCurrentFrame.mnId;
nmatches--;
}
else if(mCurrentFrame.mvpMapPoints[i]->Observations()>0)
nmatchesMap++;
}
}
if (mSensor == System::IMU_MONOCULAR || mSensor == System::IMU_STEREO)
return true;
else
return nmatchesMap>=10;
}
8、重定位 Relocalization( )
9、跟踪局部地图 TrackLocalMap( )
10、关键帧选取策略 NeedNewKeyFrame( )
bool Tracking::NeedNewKeyFrame()
{
//如果是imu模式,没初始化之前每隔0.25s就插入关键帧
if(((mSensor == System::IMU_MONOCULAR) || (mSensor == System::IMU_STEREO)) && !mpAtlas->GetCurrentMap()->isImuInitialized())
{
if (mSensor == System::IMU_MONOCULAR && (mCurrentFrame.mTimeStamp-mpLastKeyFrame->mTimeStamp)>=0.25)
return true;
else if (mSensor == System::IMU_STEREO && (mCurrentFrame.mTimeStamp-mpLastKeyFrame->mTimeStamp)>=0.25)
return true;
else
return false;
}
//纯定位模式不插入关键帧,因为局部建图线程不工作
if(mbOnlyTracking)
return false;
// If Local Mapping is freezed by a Loop Closure do not insert keyframes
//如果Local Mapping被Loop Closure请求停止了,则不插入关键帧
if(mpLocalMapper->isStopped() || mpLocalMapper->stopRequested())
{
return false;
}
// Return false if IMU is initialazing
if (mpLocalMapper->IsInitializing())
return false;
const int nKFs = mpAtlas->KeyFramesInMap();
// Do not insert keyframes if not enough frames have passed from last relocalisation
if(mCurrentFrame.mnId<mnLastRelocFrameId+mMaxFrames && nKFs>mMaxFrames)
{
return false;
}
// Tracked MapPoints in the reference keyframe
int nMinObs = 3;
if(nKFs<=2)
nMinObs=2;
//参考关键帧的地图点中,大于等于最小观测数目的地图点个数,即这些地图点被追踪到了
int nRefMatches = mpReferenceKF->TrackedMapPoints(nMinObs);
// Local Mapping accept keyframes?
bool bLocalMappingIdle = mpLocalMapper->AcceptKeyFrames();
// Check how many "close" points are being tracked and how many could be potentially created.
//统计近点中被跟踪到的个数和未跟踪到的个数(非单目和非单目imu)
int nNonTrackedClose = 0;
int nTrackedClose= 0;
if(mSensor!=System::MONOCULAR && mSensor!=System::IMU_MONOCULAR)
{
//特征点的个数N
int N = (mCurrentFrame.Nleft == -1) ? mCurrentFrame.N : mCurrentFrame.Nleft;
for(int i =0; i<N; i++)
{ //特征点的深度大于0小于远近点阈值
if(mCurrentFrame.mvDepth[i]>0 && mCurrentFrame.mvDepth[i]<mThDepth)
{
if(mCurrentFrame.mvpMapPoints[i] && !mCurrentFrame.mvbOutlier[i])
nTrackedClose++;//该点不是外点,则追踪到的近点加一
else
nNonTrackedClose++;//是外点,未追踪到的近点加一
}
}
}
bool bNeedToInsertClose;//跟踪到的近点不多,但未跟踪到的近点很多,说明跟踪得不好
bNeedToInsertClose = (nTrackedClose<100) && (nNonTrackedClose>70);
//当前帧和参考关键帧跟踪到点的比例。阈值越大,越容易达到插入关键帧的条件
float thRefRatio = 0.75f;
if(nKFs<2)
thRefRatio = 0.4f;
//单目插入最频繁
if(mSensor==System::MONOCULAR)
thRefRatio = 0.9f;
if(mpCamera2) thRefRatio = 0.75f;
if(mSensor==System::IMU_MONOCULAR)
{
if(mnMatchesInliers>350) // Points tracked from the local map
thRefRatio = 0.75f;
else
thRefRatio = 0.90f;
}
// Condition 1a: More than "MaxFrames" have passed from last keyframe insertion
const bool c1a = mCurrentFrame.mnId>=mnLastKeyFrameId+mMaxFrames;//太久
// Condition 1b: More than "MinFrames" have passed and Local Mapping is idle
const bool c1b = ((mCurrentFrame.mnId>=mnLastKeyFrameId+mMinFrames) && bLocalMappingIdle);
//Condition 1c: tracking is weak
const bool c1c = mSensor!=System::MONOCULAR && mSensor!=System::IMU_MONOCULAR && mSensor!=System::IMU_STEREO && (mnMatchesInliers<nRefMatches*0.25 || bNeedToInsertClose) ;
// Condition 2: Few tracked points compared to reference keyframe. Lots of visual odometry compared to map matches.
const bool c2 = (((mnMatchesInliers<nRefMatches*thRefRatio || bNeedToInsertClose)) && mnMatchesInliers>15);
// Temporal condition for Inertial cases
bool c3 = false;
if(mpLastKeyFrame)
{ //imu模式下超过0.5s之后则c3为true
if (mSensor==System::IMU_MONOCULAR)
{
if ((mCurrentFrame.mTimeStamp-mpLastKeyFrame->mTimeStamp)>=0.5)
c3 = true;
}
else if (mSensor==System::IMU_STEREO)
{
if ((mCurrentFrame.mTimeStamp-mpLastKeyFrame->mTimeStamp)>=0.5)
c3 = true;
}
}
bool c4 = false;
if ((((mnMatchesInliers<75) && (mnMatchesInliers>15)) || mState==RECENTLY_LOST) && ((mSensor == System::IMU_MONOCULAR))) // MODIFICATION_2, originally ((((mnMatchesInliers<75) && (mnMatchesInliers>15)) || mState==RECENTLY_LOST) && ((mSensor == System::IMU_MONOCULAR)))
c4=true;
else
c4=false;
if(((c1a||c1b||c1c) && c2)||c3 ||c4)
{
// If the mapping accepts keyframes, insert keyframe.
// Otherwise send a signal to interrupt BA
if(bLocalMappingIdle)
{
return true;
}
else
{ //局部建图繁忙的话,请求停止局部BA
mpLocalMapper->InterruptBA();
if(mSensor!=System::MONOCULAR && mSensor!=System::IMU_MONOCULAR)
{ //非单目和非单目imu模式,且关键帧队列中小于3,可以插入
if(mpLocalMapper->KeyframesInQueue()<3)
return true;
else
return false;
}
else //单目和单目imu模式直接不能插入,因为单目本来就插入比较密集,这里就不需要插入了
return false;
}
}
else
return false;
}
End
声明:部分内容来源于网络,仅供读者学术交流之目的。文章版权归原作者所有。如有不妥,请联系删除。
评论