7个极其重要的Pandas函数!
公众号:尤而小屋
作者:Peter
编辑:Peter
大家好,我是Peter~
本文介绍Pandas中7个极其重要的函数,它们在日常的数据分析会经常使用。
isnull apply groupby unique reset_index sort_values to_csv
同时你还将学会如何造假数据
。
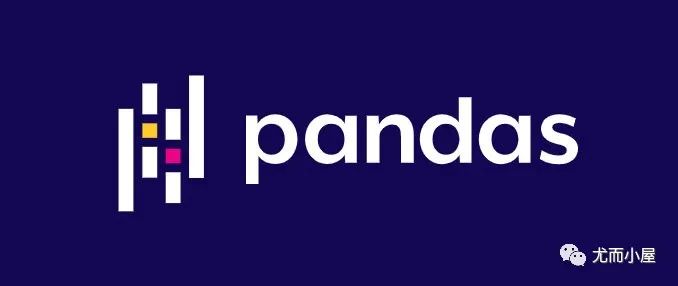
模拟数据
通过numpy和pandas库模拟一份数据,使用了numpy.random.choice
方法
In [1]:
import pandas as pd
import numpy as np
In [2]:
names = ["apple","orange","pear","grape","banana","cantaloupe"]
places = ["陕西","江西","山西","甘肃","新疆","江苏","浙江"]
In [3]:
df = pd.DataFrame({"name": np.random.choice(names, 30), # 名称
"place": np.random.choice(places, 30), # 产地
"price": np.random.randint(1,10,30), # 单价
"weight":np.random.randint(100,1000,30) # 重量
})
df.head(10) # 显示前10条数据
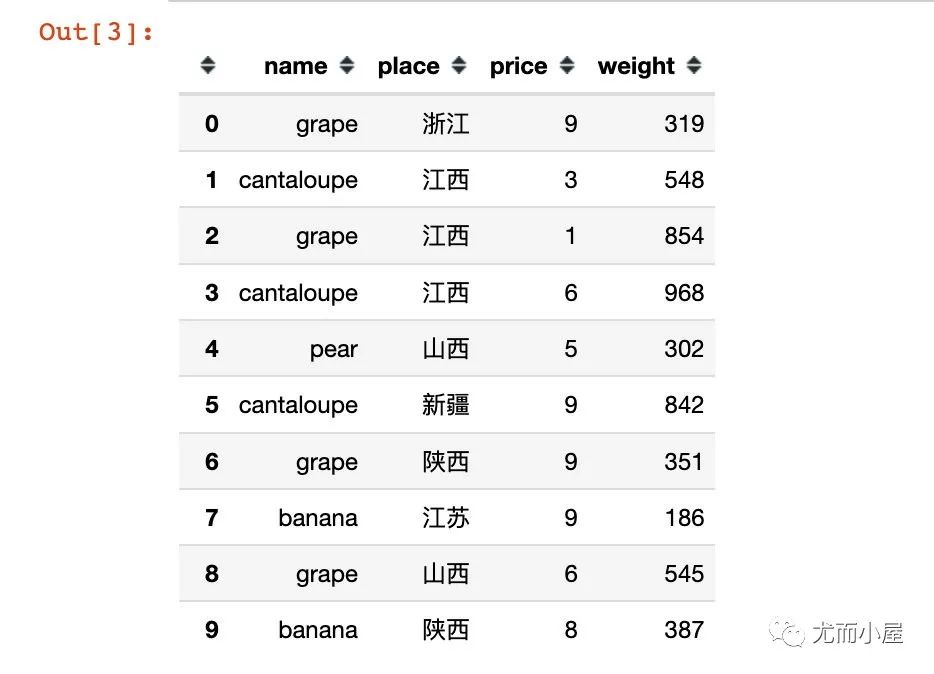
函数1:isnull
查看数据中的缺失值信息。
下面是直接查看数据中的每个位置是否为缺失值:是为True;否为False
In [4]:
df.isnull()
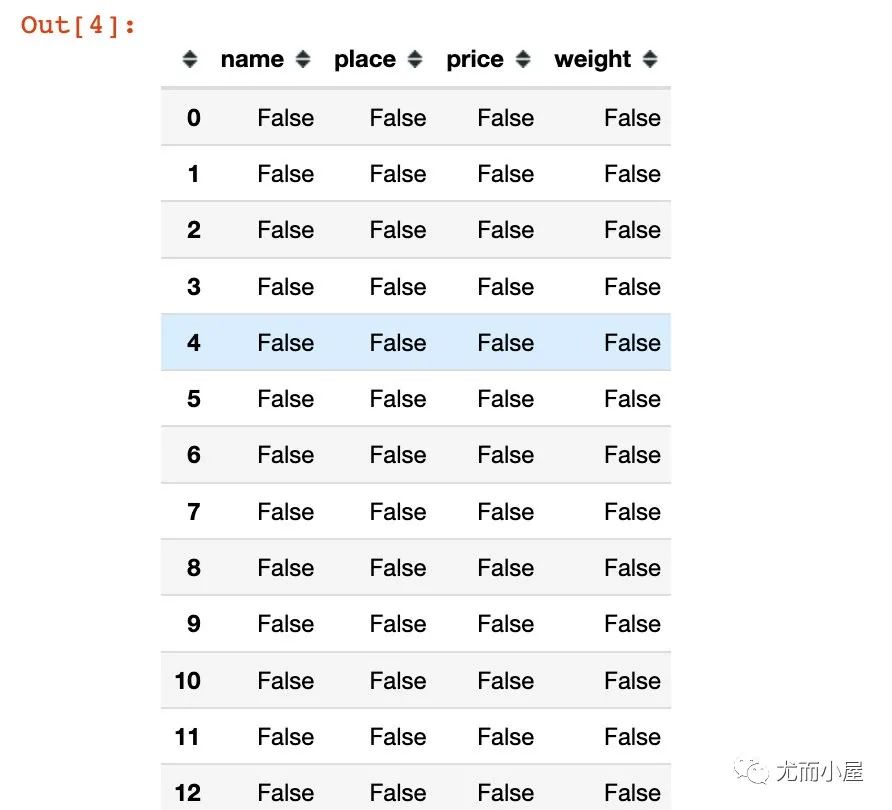
查看每个字段是否存在缺失值;在执行sum的时候,False表示0,True表示1:
In [5]:
df.isnull().sum()
Out[5]:
name 0
place 0
price 0
weight 0
dtype: int64
上面的结果默认是在列方向上求和;可以执行在行axis=1上求和:
In [6]:
df.isnull().sum(axis=1)
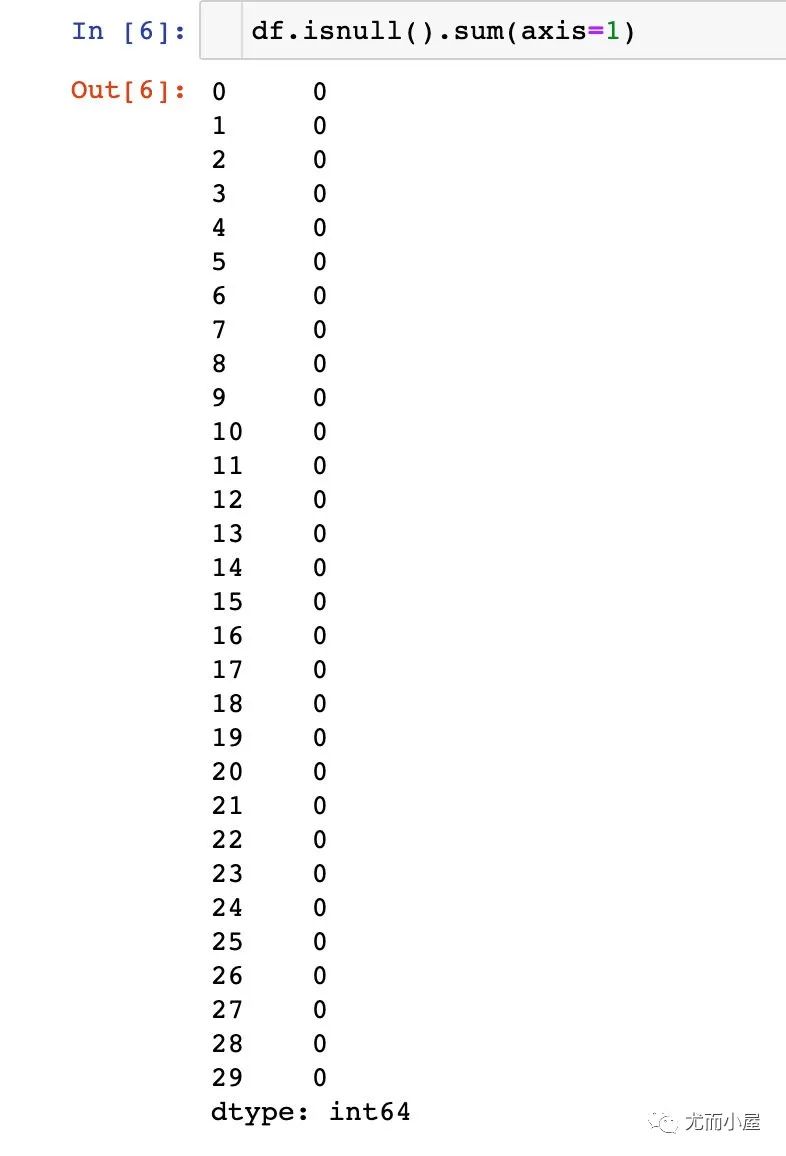
函数2:apply
apply能够对pandas中的数据进行批量操作。
1、单个字段的操作
比如将name字段全部变成首字母大写:
In [7]:
df["new_name"] = df["name"].apply(lambda x: x.title())
df.head()
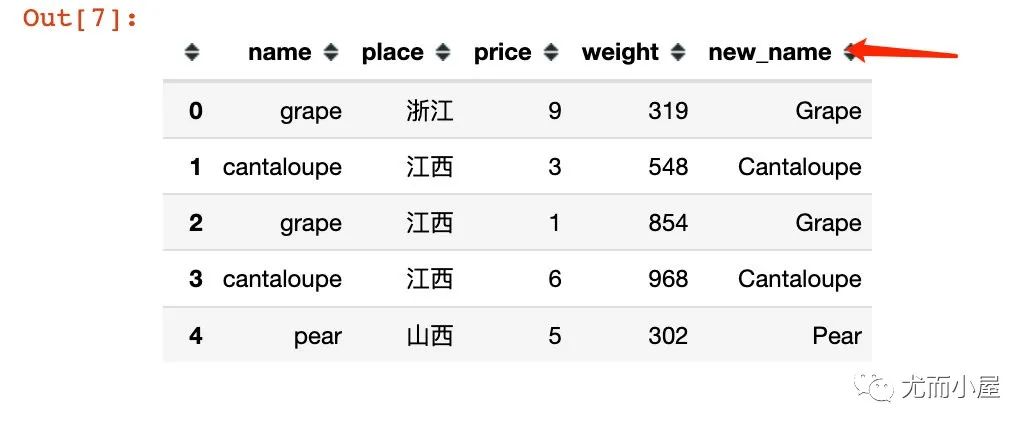
2、多个字段的同时操作:
比如根据price和weight求乘积,结果为amount:
In [8]:
df["amount"] = df[["price","weight"]].apply(lambda x: x["price"] * x["weight"], axis=1)
df.head()
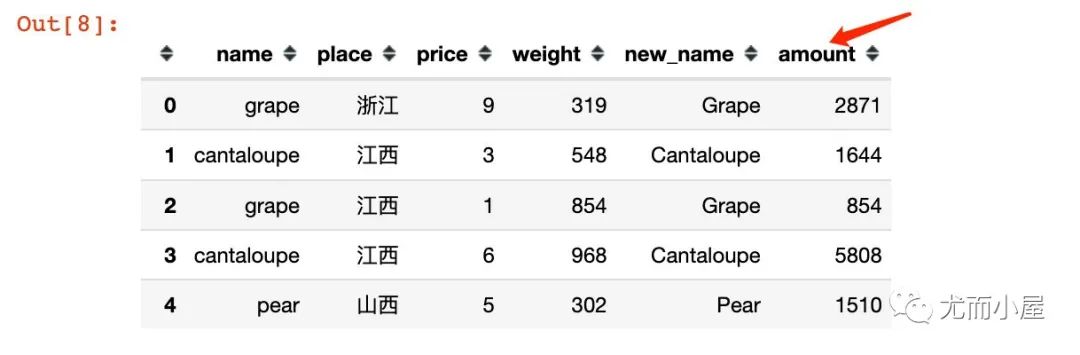
函数3:groupby
进行分组聚合的操作:
In [9]:
# 1、统计不同水果的总金额
df.groupby("name")["amount"].sum()
Out[9]:
name
apple 13360
banana 12570
cantaloupe 18777
grape 20960
orange 29995
pear 3694
Name: amount, dtype: int64
上面得到的结果是Series型数据;如果是groupby + agg,得到的则是DataFrame型数据:
In [10]:
# groupby + agg
df.groupby("name").agg({"amount":sum})
Out[10]:
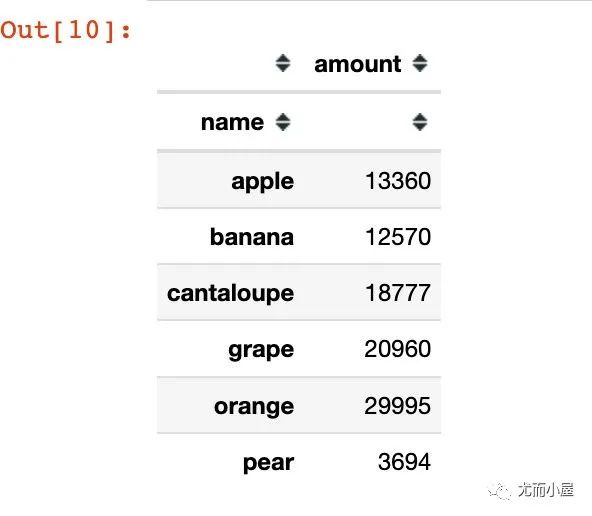
In [11]:
# groupby + to_frame 结果也变成了DataFrame型数据
df.groupby("name")["amount"].sum().to_frame()
Out[11]:
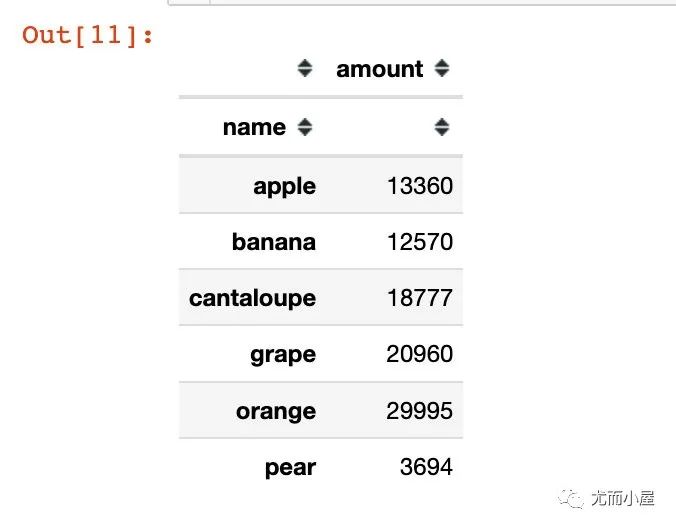
函数4:unique
统计数据的唯一值信息。比如统计每个水果name对应的产地信息place:
In [12]:
df.groupby("name")["place"].unique() # 有去重
Out[12]:
name
apple [浙江, 山西]
banana [江苏, 陕西, 山西, 江西, 甘肃, 浙江]
cantaloupe [江西, 新疆, 山西]
grape [浙江, 江西, 陕西, 山西, 江苏]
orange [浙江, 山西, 江苏]
pear [山西, 新疆]
Name: place, dtype: object
In [13]:
df.groupby("name")["place"].apply(list) # 不去重
Out[13]:
name
apple [浙江, 山西]
banana [江苏, 陕西, 山西, 江西, 甘肃, 浙江]
cantaloupe [江西, 江西, 新疆, 山西, 新疆]
grape [浙江, 江西, 陕西, 山西, 浙江, 江苏, 浙江, 江苏] # 有重复值
orange [浙江, 山西, 山西, 江苏, 山西, 浙江, 山西]
pear [山西, 新疆]
Name: place, dtype: object
如果是直接使用list函数,则会在对应的列表中展示全部的数据(包含重复值):
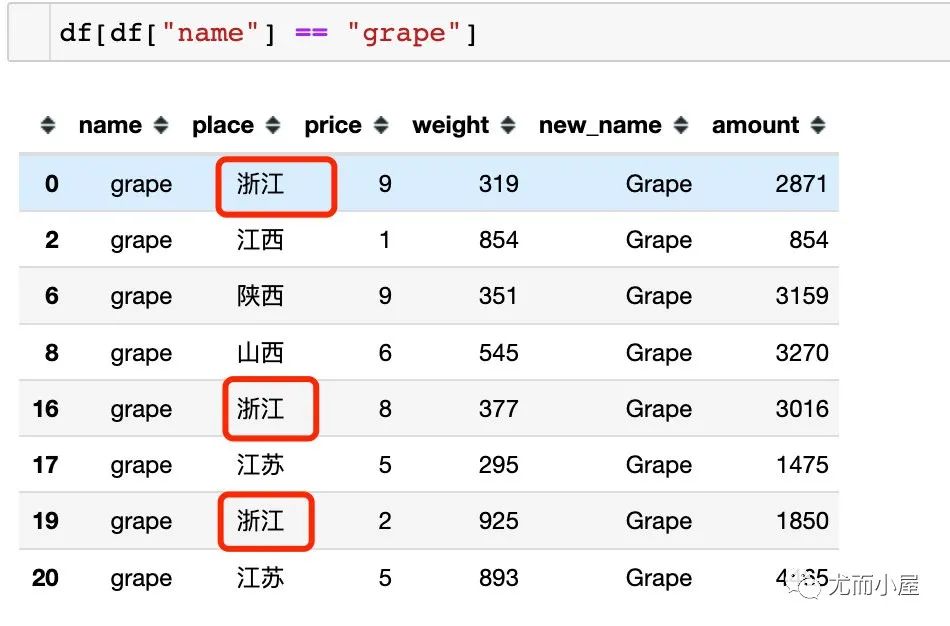
函数5:reset_index
将DataFrame的索引进行重置:
In [15]:
data = df.groupby("name")["amount"].sum() # 不加reset_index()
data
Out[15]:
name
apple 13360
banana 12570
cantaloupe 18777
grape 20960
orange 29995
pear 3694
Name: amount, dtype: int64
In [16]:
type(data) # Series型数据
Out[16]:
pandas.core.series.Series
In [17]:
data = df.groupby("name")["amount"].sum().reset_index() # 不加reset_index()
data
Out[17]:
name | amount | |
---|---|---|
0 | apple | 13360 |
1 | banana | 12570 |
2 | cantaloupe | 18777 |
3 | grape | 20960 |
4 | orange | 29995 |
5 | pear | 3694 |
In [18]:
type(data) # DataFrame数据
Out[18]:
pandas.core.frame.DataFrame
函数6:sort_values
sort_values是对数据进行排序,默认是升序
In [19]:
data # 使用上面的data数据
Out[19]:
name | amount | |
---|---|---|
0 | apple | 13360 |
1 | banana | 12570 |
2 | cantaloupe | 18777 |
3 | grape | 20960 |
4 | orange | 29995 |
5 | pear | 3694 |
In [20]:
data.sort_values(by="amount") # 默认升序
Out[20]:
name | amount | |
---|---|---|
5 | pear | 3694 |
1 | banana | 12570 |
0 | apple | 13360 |
2 | cantaloupe | 18777 |
3 | grape | 20960 |
4 | orange | 29995 |
In [21]:
data.sort_values(by="amount",
ascending=False # 指定降序
)
Out[21]:
name | amount | |
---|---|---|
4 | orange | 29995 |
3 | grape | 20960 |
2 | cantaloupe | 18777 |
0 | apple | 13360 |
1 | banana | 12570 |
5 | pear | 3694 |
In [22]:
data.sort_values(by="amount",
ascending=False, # 指定降序
ignore_index=True # 忽略索引设置
)
Out[22]:
name | amount | |
---|---|---|
0 | orange | 29995 |
1 | grape | 20960 |
2 | cantaloupe | 18777 |
3 | apple | 13360 |
4 | banana | 12570 |
5 | pear | 3694 |
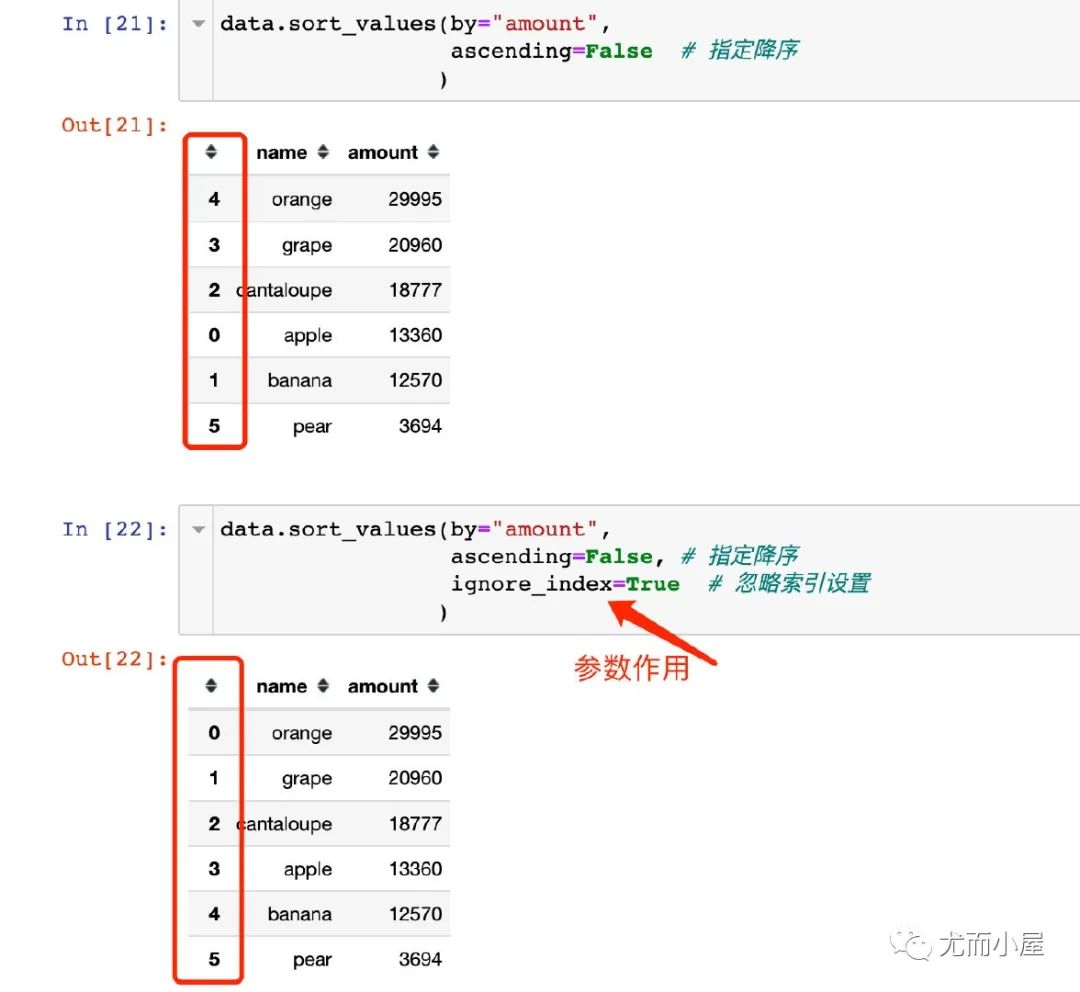
函数7:to_csv
将DataFrame保存成csv格式:
In [23]:
df.to_csv("newdata.csv",index=False) # csv格式
# df.to_csv("newdata.xls",index=False) # Excel格式
点击关注公众号,阅读更多精彩内容