用Python分析了5万条相亲网站数据,看相亲男女画像
共 6108字,需浏览 13分钟
·
2021-02-02 09:26
这短短的一生,我们最终都会失去。你不妨大胆一些,爱一个人,攀一座山,追一个梦。
一、前言
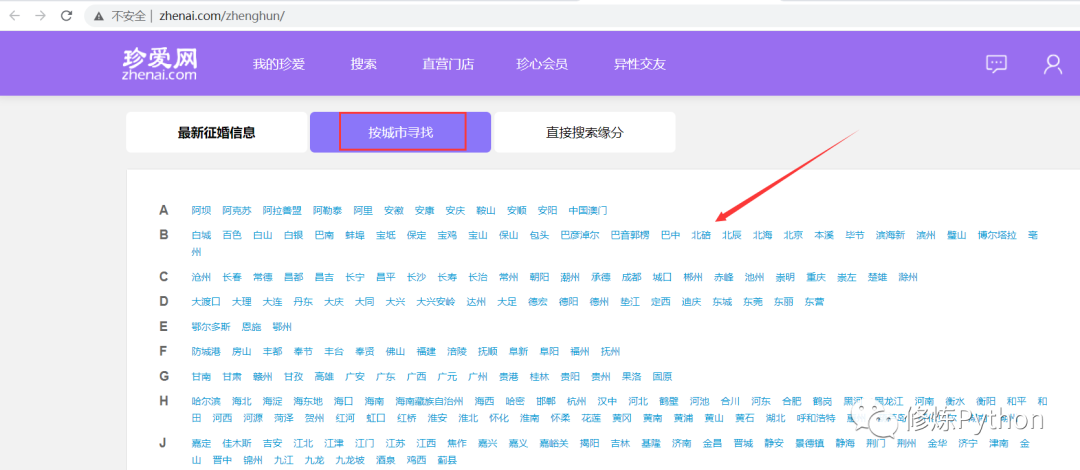
二、数据查看和预处理
import pandas as pd
import re
df = pd.read_excel('marriage.xlsx')
df.head()
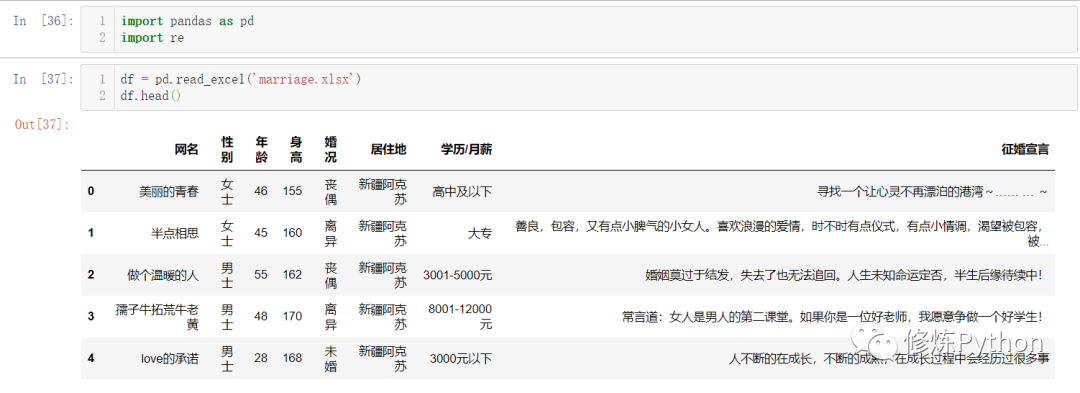
df.info()
可以看到数据都没有缺失值。
获取到的数据里,居住地是各地区的,为了便于分析,需要处理成省级行政区,学历/月薪那一列数据,有些是月薪,有些是学历,可以分别处理成两列数据,是学历的,提取出学历层次,月薪标记为 "未知";是月薪的,提取出月薪并计算,学历标记为 "未知"。
# 获取34个省级行政区域,包括23个省,5个自治区,4个直辖市,2个特别行政区的名称
with open('地区.txt', 'r', encoding='utf-8') as f:
area = f.read().split('\n')
print(area)
print(len(area))
['北京', '上海', '天津', '重庆', '黑龙江', '吉林', '辽宁', '内蒙古', '河北', '新疆', '甘肃', '青海', '陕西', '宁夏', '河南', '山东', '山西', '安徽', '湖北', '湖南', '江苏', '四川', '贵州', '云南', '广西', '西藏', '浙江', '江西', '广东', '福建', '台湾', '海南', '香港', '澳门']
34
areas_list = []
for i in df['居住地']:
for j in area:
if j in i:
areas_list.append(j)
break
else:
areas_list.append('未知')
df['居住地'] = areas_list
df.head()
结果如下:
with open('学历.txt', 'r', encoding='utf-8') as fp:
edu = fp.read().split('\n')
print(edu)
['博士', '硕士', '本科', '大专', '中专', '高中', '初中', '小学']
salary_list = []
edu_list = []
for item in df['学历/月薪']:
if '元' in item: # 这一列的数据是表达月薪的话 计算
data = re.findall('\d+', item)
data = [int(x) for x in data]
salary = int(sum(data) / len(data)) # 取整
salary_list.append(salary)
edu_list.append('未知')
else:
salary_list.append('未知')
for e in edu:
if e in item:
edu_list.append(e)
break
else:
edu_list.append('未知')
print(len(edu_list))
print(len(salary_list))
df['学历'] = edu_list
df['月薪'] = salary_list
df.head()
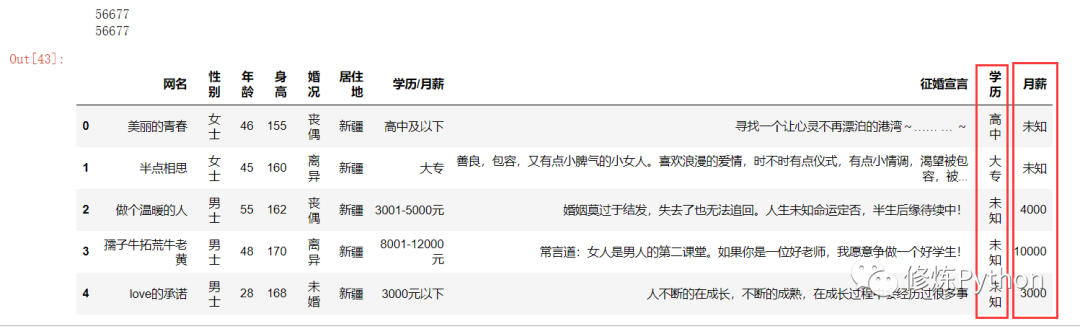
del df['学历/月薪']
df
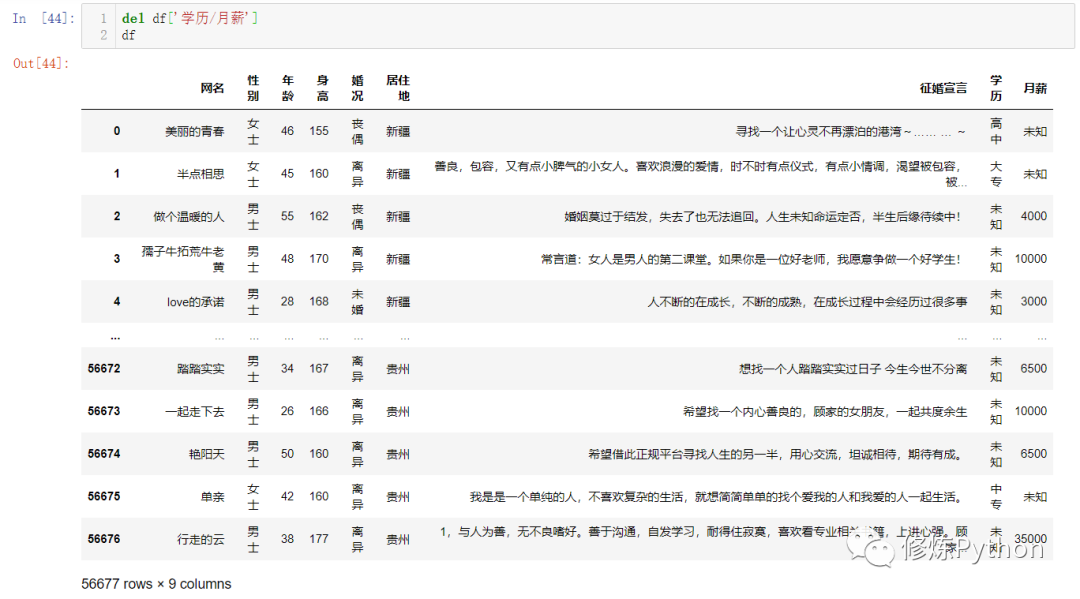
df.to_excel('处理后数据.xlsx', index=False)
三、数据分析
# -*- coding: UTF-8 -*-
"""
@File :男女占比情况.py
@Author :叶庭云
@CSDN :https://yetingyun.blog.csdn.net/
"""
import pandas as pd
import collections
from pyecharts.charts import Pie
from pyecharts import options as opts
from pyecharts.globals import ThemeType, CurrentConfig
# 引用本地js资源渲染
CurrentConfig.ONLINE_HOST = 'D:/python/pyecharts-assets-master/assets/'
# 提取数据
df = pd.read_excel('处理后数据.xlsx')
gender = list(df['性别'])
# 统计男女人数
gender_count = collections.Counter(gender).most_common()
gender_count = [(k, v) for k, v in gender_count]
pie = Pie(init_opts=opts.InitOpts(theme=ThemeType.MACARONS))
# 富文本效果 环图
pie.add('性别', data_pair=gender_count, radius=["40%", "55%"],
label_opts=opts.LabelOpts(
position="outside",
formatter="{a|{a}}{abg|}\n{hr|}\n {b|{b}: }{c} {per|{d}%} ",
background_color="#eee",
border_color="#aaa",
border_width=1,
border_radius=4,
rich={
"a": {"color": "#999", "lineHeight": 22, "align": "center"},
"abg": {
"backgroundColor": "#e3e3e3",
"width": "100%",
"align": "right",
"height": 22,
"borderRadius": [4, 4, 0, 0],
},
"hr": {
"borderColor": "#aaa",
"width": "100%",
"borderWidth": 0.5,
"height": 0,
},
"b": {"fontSize": 16, "lineHeight": 33},
"per": {
"color": "#eee",
"backgroundColor": "#334455",
"padding": [2, 4],
"borderRadius": 2,
},
},
),)
pie.set_global_opts(title_opts=opts.TitleOpts(title='相亲男女占比情况'))
pie.set_colors(['red', 'blue']) # 设置颜色
pie.render('男女占比情况.html')
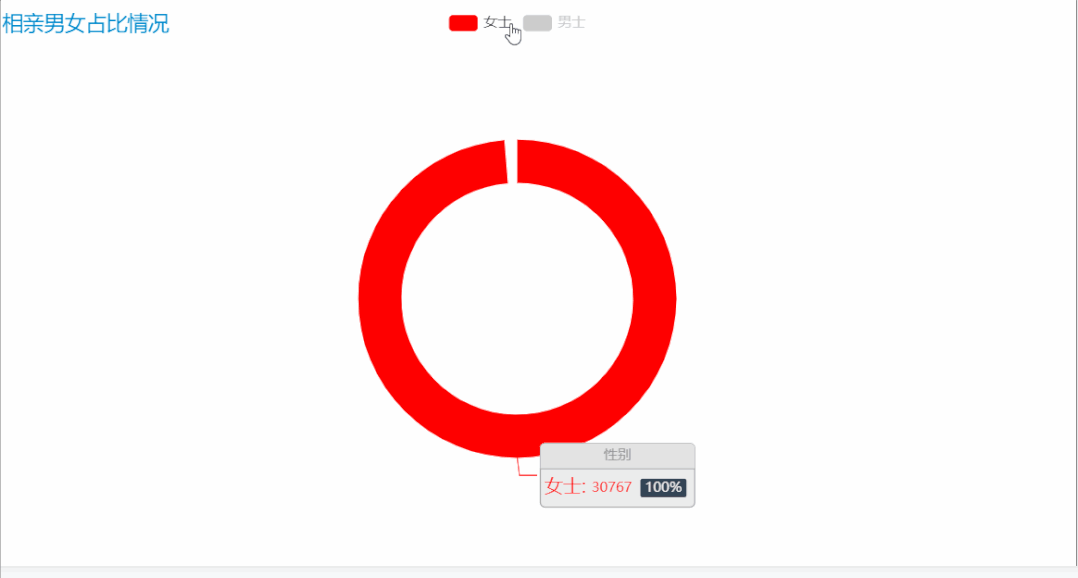
相亲男女中女士多于男士。男士有 25910 人,占比45.72%;女士有 30767 人,占比54.28%。
# -*- coding: UTF-8 -*-
"""
@File :年龄分布.py
@Author :叶庭云
@CSDN :https://yetingyun.blog.csdn.net/
"""
import pandas as pd
import collections
from pyecharts.charts import Bar
from pyecharts.globals import ThemeType, CurrentConfig
from pyecharts import options as opts
CurrentConfig.ONLINE_HOST = 'D:/python/pyecharts-assets-master/assets/'
df = pd.read_excel('处理后数据.xlsx')
age = list(df['年龄'])
age_count = collections.Counter(age).most_common()
# 按年龄排序
age_count.sort(key=lambda x: x[0])
age = [x[0] for x in age_count]
nums = [y[1] for y in age_count]
# print(age_count)
bar = Bar(init_opts=opts.InitOpts(theme=ThemeType.MACARONS))
bar.add_xaxis(age)
bar.add_yaxis('人数', nums) # 数据多的时候设置不显示标签
bar.set_global_opts(title_opts=opts.TitleOpts(title='相亲男女年龄分布'))
# 标记最大值 最小值 平均值 标记平均线
bar.set_series_opts(label_opts=opts.LabelOpts(is_show=False),
markpoint_opts=opts.MarkPointOpts(
data=[
opts.MarkPointItem(type_="max", name="最大值"),
opts.MarkPointItem(type_="min", name="最小值"),
opts.MarkPointItem(type_="average", name="平均值")]),
markline_opts=opts.MarkLineOpts(
data=[
opts.MarkLineItem(type_="average", name="平均值")]))
bar.render('年龄分布.html')
结果如下:31岁的相亲男女人数最多,有 2637 人,各个年龄段都有一定数量的相亲男女,我们可以将年龄小于等于 20 岁,大于等于 70 岁的相亲男女数据单独提取出来看看。
import pandas as pd
df = pd.read_excel('处理后数据.xlsx')
df1 = df[df['年龄'] <= 20]
df2 = df1['婚况'].value_counts() # 统计小于等于20岁的相亲男女的婚况
print(df2)
未婚 153
离异 6
丧偶 2
Name: 婚况, dtype: int64
大部分是未婚,年纪轻轻就那么急着相亲吗,再看看婚况是离异的数据。
import pandas as pd
df = pd.read_excel('处理后数据.xlsx')
df1 = df[df['年龄'] <= 20]
df3 = df1[df1['婚况'] == '离异']
print(df3)
网名 性别 ... 学历 月薪
17425 微风轻起 男士 ... 未知 50000
29645 媳妇 女士 ... 大专 未知
30398 仙妹 女士 ... 高中 未知
30485 会员1415395937 男士 ... 未知 35000
36684 微笑着变老 女士 ... 高中 未知
49864 风吹动了风玲 女士 ... 高中 未知
[6 rows x 9 columns]
月薪写着50000、35000的男士有些显眼啊,在数据集中查看。
月薪 50000 的微风轻起,征婚信息年龄写的19,征婚宣言里又写到 1994 年 26 岁;月薪 35000 的会员某某某,征婚信息年龄写的20,征婚宣言里又写到 81 年的,看来网站里年龄、身高这些信息真实性值得怀疑。
相亲男女婚况?
# -*- coding: UTF-8 -*-
"""
@File :男女占比情况.py
@Author :叶庭云
@CSDN :https://yetingyun.blog.csdn.net/
"""
import pandas as pd
import collections
from pyecharts.charts import Pie
from pyecharts import options as opts
from pyecharts.globals import ThemeType, CurrentConfig
# 引用本地js资源渲染
CurrentConfig.ONLINE_HOST = 'D:/python/pyecharts-assets-master/assets/'
# 提取数据 婚况不为未填写的
df = pd.read_excel('处理后数据.xlsx')
data = df[df['婚况'] != '未填写']
# 统计各婚况相亲男女人数
data_count = collections.Counter(data['婚况']).most_common()
print(data)
c = (
Pie()
.add(
"婚况",
data_count,
radius=["40%", "55%"],
label_opts=opts.LabelOpts(
position="outside",
formatter="{a|{a}}{abg|}\n{hr|}\n {b|{b}: }{c} {per|{d}%} ",
background_color="#eee",
border_color="#aaa",
border_width=1,
border_radius=4,
rich={
"a": {"color": "#999", "lineHeight": 22, "align": "center"},
"abg": {
"backgroundColor": "#e3e3e3",
"width": "100%",
"align": "right",
"height": 22,
"borderRadius": [4, 4, 0, 0],
},
"hr": {
"borderColor": "#aaa",
"width": "100%",
"borderWidth": 0.5,
"height": 0,
},
"b": {"fontSize": 16, "lineHeight": 33},
"per": {
"color": "#eee",
"backgroundColor": "#334455",
"padding": [2, 4],
"borderRadius": 2,
},
},
),
)
.set_colors(["#8B008B", "#FF1493", "#000000"])
.set_global_opts(title_opts=opts.TitleOpts(title="相亲男女婚况"))
.render("pie_rich_label.html")
)
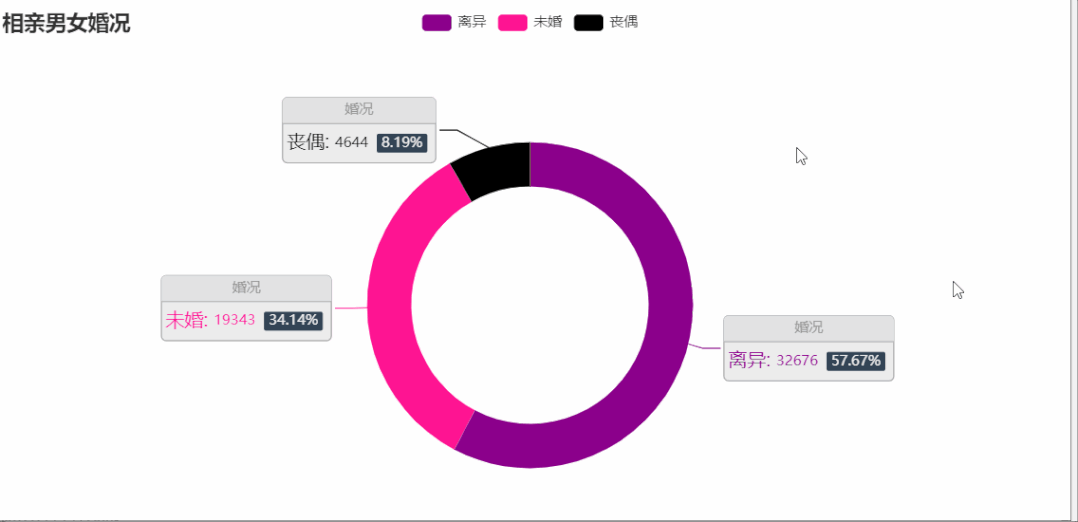
# -*- coding: UTF-8 -*-
"""
@File :学历分布.py
@Author :叶庭云
@CSDN :https://yetingyun.blog.csdn.net/
"""
import pandas as pd
import collections
from pyecharts.charts import Pie
from pyecharts import options as opts
from pyecharts.globals import CurrentConfig
# 引用本地js资源渲染
CurrentConfig.ONLINE_HOST = 'D:/python/pyecharts-assets-master/assets/'
# 提取数据 学历不为未知的
df = pd.read_excel('处理后数据.xlsx')
data = df[df['学历'] != '未知']
# print(data)
# 统计各学历层次相亲男女数量
data_count = collections.Counter(data['学历']).most_common()
c = (
# 宽 高 背景颜色
Pie(init_opts=opts.InitOpts(width="800px", height="500px", bg_color="#2c343c"))
.add(
series_name="相亲男女学历", # 系列名称
data_pair=data_count, # 系列数据项,格式为 [(key1, value1), (key2, value2)...]
rosetype="radius", # radius:扇区圆心角展现数据的百分比,半径展现数据的大小
radius="55%", # 饼图的半径
center=["50%", "50%"], # 饼图的中心(圆心)坐标,数组的第一项是横坐标,第二项是纵坐标
label_opts=opts.LabelOpts(is_show=False, position="center"), # 标签配置项
)
.set_colors(["#00BFFF", "#00FF7F", "#FF1493", "#8B008B", "#FFFF00", "#556B2F"])
.set_global_opts(
title_opts=opts.TitleOpts(
title="相亲男女学历",
pos_left="center",
pos_top="20",
title_textstyle_opts=opts.TextStyleOpts(color="#fff"),
),
legend_opts=opts.LegendOpts(is_show=False),
)
.set_series_opts(
tooltip_opts=opts.TooltipOpts(
trigger="item", formatter="{a}
{b}: {c} ({d}%)" # 'item': 数据项图形触发,主要在散点图,饼图等无类目轴的图表中使用
),
label_opts=opts.LabelOpts(color="#fff"),
)
.render("相亲男女学历.html")
)
结果如下:相亲男女学历大部分在高中(35.92%)、大专(24.72%),有近六成的相亲男女。本科占比20.7%,中专占比16.35%,硕士、博士高学历的相亲男女人数很少,分别占比2.14%,0.17%。
# -*- coding: UTF-8 -*-
"""
@File :地区分布.py
@Author :叶庭云
@CSDN :https://yetingyun.blog.csdn.net/
"""
import pandas as pd
import collections
from pyecharts import options as opts
from pyecharts.charts import Geo
from pyecharts.globals import ChartType
from pyecharts.globals import ThemeType, CurrentConfig
CurrentConfig.ONLINE_HOST = 'D:/python/pyecharts-assets-master/assets/'
df = pd.read_excel('处理后数据.xlsx')
area = list(df['居住地'])
area_count = collections.Counter(area).most_common(34)
print(area_count)
# 初始化配置项 背景颜色 大小 主题
geo = Geo(init_opts=opts.InitOpts(width='1000px', height='600px', theme=ThemeType.DARK))
# 设置是否显示省份
geo.add_schema(maptype='china', label_opts=opts.LabelOpts(is_show=True))
# 绘制什么类型图 热力图 涟漪图等
geo.add('相亲男女人数', data_pair=area_count, type_=ChartType.EFFECT_SCATTER)
geo.set_series_opts(label_opts=opts.LabelOpts(is_show=False)) # 不显示数据标签
geo.set_global_opts(title_opts=opts.TitleOpts(title="相亲男女地区分布"),
visualmap_opts=opts.VisualMapOpts(max_=5000, is_piecewise=True, # 划分区间是否精确
pieces=[{"max": 1000, "min": 100, "label": "100-1000", "color": "#708090"}, # 分段 添加图例注释 和颜色
{"max": 1500, "min": 1001, "label": "1001-1500", "color": "#00008B"},
{"max": 2000, "min": 1501, "label": "1501-2000", "color": "#483D8B"},
{"max": 2500, "min": 2001, "label": "2001-2500", "color": "#1E90FF"},
{"max": 3000, "min": 2501, "label": "2501-3000", "color": "#8B008B"},
{"max": 5000, "min": 3001, "label": ">=3000", "color": "#FF0000"}])
)
geo.render('地区分布.html')
[('重庆', 4436), ('广东', 2637), ('四川', 2519), ('山东', 2398), ('河南', 2160), ('上海', 2156), ('云南', 2039), ('北京', 2037), ('台湾', 1997), ('安徽', 1920), ('江苏', 1919), ('天津', 1918), ('黑龙江', 1918), ('湖南', 1800), ('新疆', 1799), ('辽宁', 1680), ('甘肃', 1680), ('广西', 1679), ('湖北', 1679), ('内蒙古', 1559), ('山西', 1440), ('福建', 1440), ('江西', 1440), ('浙江', 1440), ('陕西', 1439), ('河北', 1439), ('青海', 1339), ('贵州', 1200), ('吉林', 1080), ('西藏', 942), ('宁夏', 702), ('海南', 360), ('香港', 353), ('澳门', 117)]
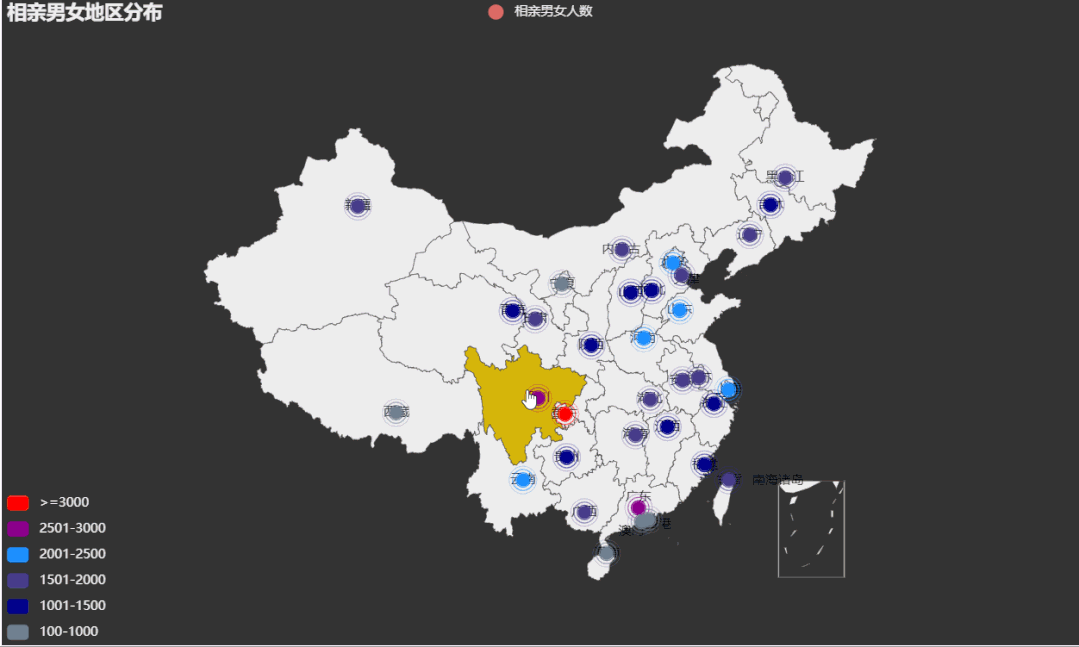
重庆、广东、四川等地区的相亲男女人数最多。
征婚宣言一般是介绍自己情况,表达对另一半的要求和期望。下面我们分别来看看相亲男女征婚宣言里关键词都有些什么。
# -*- coding: UTF-8 -*-
"""
@File :征婚宣言词云.py
@Author :叶庭云
@CSDN :https://yetingyun.blog.csdn.net/
"""
import pandas as pd
import jieba
import collections
import re
from wordcloud import WordCloud
import matplotlib.pyplot as plt
import numpy as np
from PIL import Image
# 提取性别 征婚宣言这两列数据就好
df = pd.read_excel('处理后数据.xlsx')[['性别', '征婚宣言']]
# df1 = df[df['性别'] == '女士']['征婚宣言']
df2 = df[df['性别'] == '女士']['征婚宣言']
# 读取停用词数据
with open('stop_words.txt', encoding='utf-8') as f:
con = f.read().split('\n') # 得到每一行的停用词
stop_words = set()
for i in con:
stop_words.add(i)
result_list = []
for data in df2:
# 文本预处理 去除一些无用的字符 只提取出中文出来
new_data = re.findall('[\u4e00-\u9fa5]+', data, re.S)
new_data = "/".join(new_data)
# 文本分词
seg_list_exact = jieba.cut(new_data, cut_all=True)
# 去除停用词和单个词
for word in seg_list_exact:
if word not in stop_words and len(word) > 1:
result_list.append(word)
print(result_list)
# 筛选后统计
word_counts = collections.Counter(result_list)
mask_ = 255 - np.array(Image.open('woman_mask.png'))
# 绘制词云
my_cloud = WordCloud(
background_color='white', # 设置背景颜色 默认是black
mask=mask_,
font_path='simhei.ttf', # 设置字体 显示中文
max_font_size=112, # 设置字体最大值
min_font_size=12, # 设置字体最小值
random_state=88 # 设置随机生成状态,即多少种配色方案
).generate_from_frequencies(word_counts)
# 绘制词云
plt.figure(figsize=(8, 5), dpi=200)
# 显示生成的词云图片
plt.imshow(my_cloud, interpolation='bilinear')
# 显示设置词云图中无坐标轴
plt.axis('off')
plt.savefig('woman_cloud.png', dpi=200)
plt.show()
结果如下:
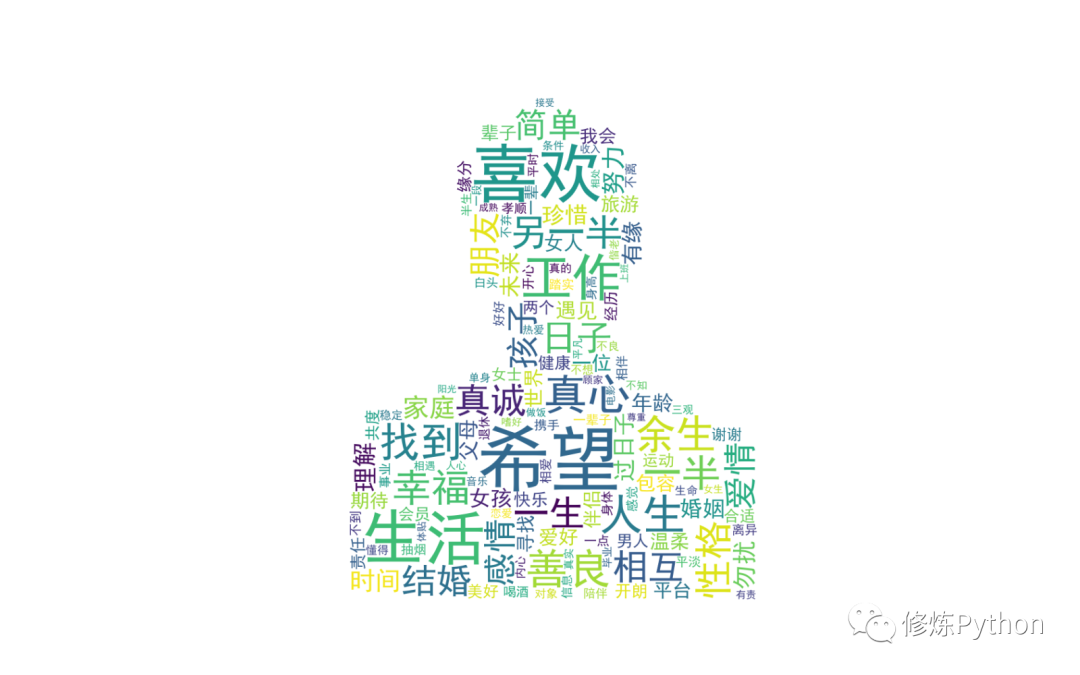
相亲男女征婚宣言里,喜欢、希望、生活、善良、真诚、真心、幸福、性格等都是出现频率高的词语。
见面礼
扫码加我微信备注「三剑客」送你上图三本Python入门电子书
推荐阅读



