Flink+Iceberg搭建实时数据湖实战

第一部分:Iceberg 核心功能原理剖析 :
Apache Iceberg
摘自官网:
Apache Iceberg is an open table format for huge analytic datasets.
可以看到 Founders 对 Iceberg 的定位是面向海量数据分析场景的高效存储格式。海量数据分析的场景,类比于 Hive 是 Hdfs 的封装一样,本质上解决的还是数仓场景的痛点问题。
Iceberg 在最开始,也确实是在数仓场景朝着更快更好用的 format 目标不断演进,比如支持 schema 变更,文件粒度的 Filter 优化等,但随着和流式计算 Flink 引擎的生态打通,Delete/Update/Merge 语义的出现,场景就会变得多样化起来。
背景
过去业界更多是使用 Hive/Spark on HDFS 作为离线数据仓库的载体,在越来越趋于实时化和快速迭代的场景中,逐渐暴露出以下缺点:
不支持 Row-Level-Update,对于更新的操作需要 overwrite 整张 Hive 表,成本极高 不支持读写分离,用户的读取操作会被另一个用户的写入操作所影响(尤其是流式读取的场景) 不支持版本回滚和快照,需要保存大量历史数据 不支持增量读取,每次扫描全表或分区所有数据 性能低,只能裁剪到 Hive Partition 粒度 不支持 Schema 变更 .....
基本概念
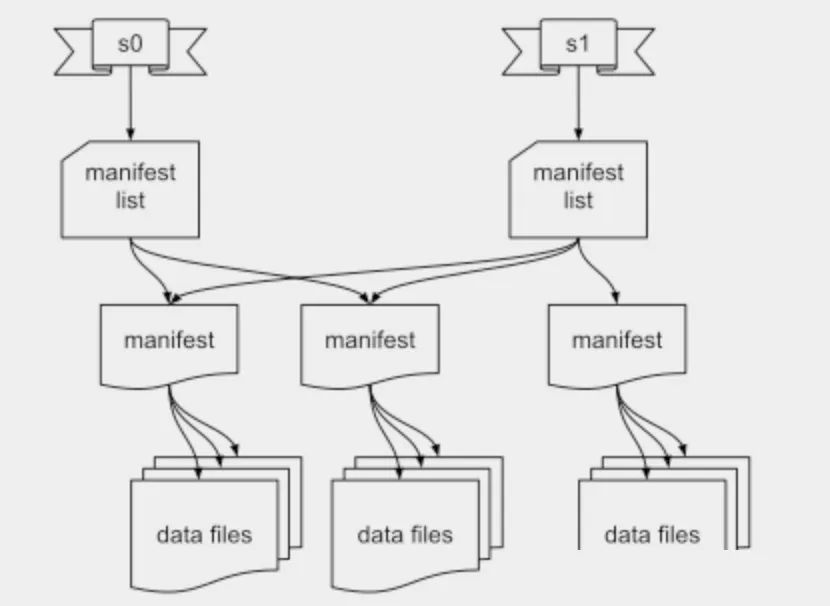
如上图所示,iceberg 将 hdfs 上的文件进行了 snapshot、manifest list、manifest、data files 的分层。
Snapshot:用户的每次 commit(每次写入的 spark job) 会产生一个新的 snapshot Manifest List:维护当前 snapshot 中所有的 manifest Manifest:维护当前 Manifest 下所有的 data files Data File:存储数据的文件,后续 Iceberg 引入了 Delete File,用于存储要删除的数据,文件结构上也是与 Data File 处在同一层
核心功能剖析
Time Travel 和增量读取
Time Travel 指的是用户可以任意读取历史时刻的相关数据,以 Spark 的代码为例:
// time travel to October 26, 1986 at 01:21:00
spark.read
.option("as-of-timestamp", "499162860000")
.format("iceberg")
.load("path/to/table")
上述代码即是在读取 timestamp=499162860000 时,该 Iceberg 表的数据,那么底层原理是什么样子的呢?
从「基本概念」中的文件结构可以看到,用户每次新的写入都会产生一个 snapshot,那么 Iceberg 只需要存储用户每次 commit 产生的 metadata,比如时间戳等信息,就能找到对应时刻的 snapshot,并且解析出 Data Files。
增量读取也同理,通过 start 和 end 的时间戳取到时间范围内的 snapshot,并读取所有的 Data Files 作为原始数据。
Fast Scan & Data Filtering
上面提到 Hive 的查询性能低下,其中一个原因是数据计算时,只能下推到 Partition 层面,粒度太粗。而 Iceberg 在细粒度的 Plan 上做了一系列的优化,当一个 Query 进入 Iceberg 后:
根据 timestamp 找到对应的 snapshot(默认最新) 根据 Query 的 Partition 信息从指定 snapshot 中过滤出符合条件的 manifest 文件集合 从 manifest 文件集合中取出所有的 Data Files 对象(只包含元信息) 根据 Data File 的若干个属性,进行更细粒度的数据过滤,包括 column-level value counts, null counts, lower bounds, and upper bounds 等
Delete 实现
为了上线 Row-Level Update 的功能,Iceberg 提供了 Delete 的实现,通过 Delete + Insert 我们可以达到 Update 的目的。在引入 Delete 实现时,引入了两个概念:
Delete File:用于存储删除的数据(分为 position delete 和 equality delete) Sequence Number:是 Data File 和 Delete File 的共有属性之一,主要用于区分 Insert 和 Delete 的先后顺序,否则会出现数据一致性的问题
position & equality delete
Iceberg 引入了 equality_ids 概念,用户建表时可以指定 Table 的 equality_ids 来标识未来 Delete 操作对应的 Key,比如 GDPR 场景,我们需要根据 user_id 来随机删除用户的相关数据,就可以把 equality_ids 设置为 user_id。
两种 Delete 操作对应不同的 Delete File,其存储字段也不同:
position delete:包括三列,file_path(要删除的数据所在的 Data File)、pos(行数)、row(数据) equality delete:包括 equality_ids 中的字段
显而易见,存储 Delete File 的目的是将来读取数据时,进行实时的 Join,而 position delete 在 Join 时能精准定位到文件,并且只需要行号的比较,肯定是更加高效的。所以在 Delete 操作写入时,Iceberg 会将正在写入的数据文件信息存储到内存中,来保证将 DELETE 操作尽量走 position delete 的链路。示意图如下所示:
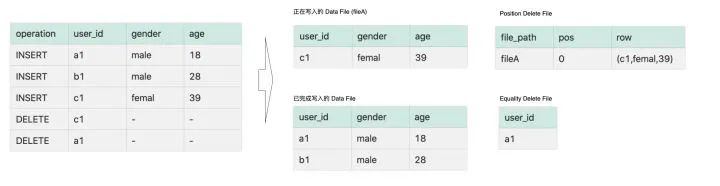
按照时间顺序,依次写入三条 INSERT 和 DELETE 数据,假设 Iceberg Writer 在写入 a1 和 b1 的 INSERT 数据后,就关闭并新开启了一个文件,那么此时写入的记录 c1 和对应的行号会被记录在内存中。此时 Writer 接收到 user_id=c1 的数据后,便能直接从内存中找到 user_id=c1 的数据是在 fileA 中的第一行,此时写下一个 Position Delete File;而 user_id=a1 的 DELETE 数据,由于文件已经关闭,内存中没有记录其信息,所以写下一个 Equality Delete File。
Sequence Number
引入 DELETE 操作后,如果在读取时进行合并,则涉及到一个问题,如果用户对同一个 equality_id 的数据进行插入、删除、再插入,那么读取时该如何保证把第一次插入的数据给删掉,读取第二次插入的数据?
这里的处理方式是将 Data File 和 Delete File 放在一起按写入顺序编号,在读取时,DELETE 只对小于当前 Sequence Number 的 Data File 生效。如果遇到相同记录的并发写入的时候怎么办?这里就要利用 Iceberg 自身的事务机制了,Iceberg Writer 在写入前会检查相关 meta 以及 Sequence Number,如果写入后不符合预期则会采取乐观锁的形式进行重试。
Schema Evolution
Iceberg 的 schema evolution 是其特色之一,支持以下操作:
增加字段 删除字段 重命名字段 修改字段 改变字段顺序
关于 schema 的变更也依赖上面文件结构,由于每次写入时,都会产生 snapshot -> manifest -> data file 的层级,同样,读取时也会从 snapshot 开始读取并路由到对应的底层 data file。所以 Iceberg 只需要每次写入时在 manifest 中记录下 schema 的情况,并在读取时进行对应的转换即可。
第二部分:Flink+Iceberg环境搭建:
1. Flink SQL Client配置Iceberg
Flink集群需要使用Scala 2.12版本的
将Iceberg的依赖包下载放到Flink集群所有服务器的lib目录下,然后重启Flink
[root@flink1 ~]# wget -P /root/flink-1.14.3/lib https://repo.maven.apache.org/maven2/org/apache/iceberg/iceberg-flink-runtime-1.14/0.13.0/iceberg-flink-runtime-1.14-0.13.0.jar
[root@flink1 ~]#
[root@flink1 ~]# scp /root/flink-1.14.3/lib/iceberg-flink-runtime-1.14-0.13.0.jar root@flink2:/root/flink-1.14.3/lib
iceberg-flink-runtime-1.14-0.13.0.jar 100% 23MB 42.9MB/s 00:00
[root@flink1 ~]# scp /root/flink-1.14.3/lib/iceberg-flink-runtime-1.14-0.13.0.jar root@flink3:/root/flink-1.14.3/lib
iceberg-flink-runtime-1.14-0.13.0.jar 100% 23MB 35.4MB/s 00:00
[root@flink1 ~]#
Iceberg默认支持Hadoop Catalog。如果需要使用Hive Catalog,需要将flink-sql-connector-hive-3.1.2_2.12-1.14.3.jar放到Flink集群所有服务器的lib目录下,然后重启Flink
然后启动SQL Client就可以了
2.Java/Scala pom.xml配置
添加如下依赖
<dependency>
<groupId>org.apache.iceberg</groupId>
<artifactId>iceberg-flink</artifactId>
<version>0.13.0</version>
<scope>provided</scope>
</dependency>
3.Catalog
3.1 Hive Catalog
注意:测试的时候,从Hive中查询表数据,查询不到。但是从Trino查询可以查询到数据
使用Hive的metastore保存元数据,HDFS保存数据库表的数据
Flink SQL> create catalog hive_catalog with(
> 'type'='iceberg',
> 'catalog-type'='hive',
> 'property-version'='1',
> 'cache-enabled'='true',
> 'uri'='thrift://hive1:9083',
> 'client'='5',
> 'warehouse'='hdfs://nnha/user/hive/warehouse',
> 'hive-conf-dir'='/root/flink-1.14.3/hive_conf'
> );
[INFO] Execute statement succeed.
Flink SQL>
property-version: 为了向后兼容,以防property格式改变。当前设置为1即可 cache-enabled: 是否开启catalog缓存,默认开启 clients: 在hive metastore中,hive_catalog供客户端访问的连接池大小,默认是2 warehouse: 是Flink集群所在的HDFS路径, hive_catalog下的数据库表存放数据的位置 hive-conf-dir: hive集群的配置目录。只能是Flink集群的本地路径,从hive-site.xml解析出来的HDFS路径,是Flink集群所在HDFS路径 warehouse的优先级比hive-conf-dir的优先级高 如果Hive中已经存在要创建的数据库,则创建的表path会位于Hive的warehouse下
3.2 HDFS Catalog
用HDFS保存元数据和数据库表的数据。warehouse是Flink集群所在的HDFS路径
Flink SQL> create catalog hadoop_catalog with (
> 'type'='iceberg',
> 'catalog-type'='hadoop',
> 'property-version'='1',
> 'cache-enabled'='true',
> 'warehouse'='hdfs://nnha/user/iceberg/warehouse'
> );
[INFO] Execute statement succeed.
Flink SQL>
通过配置conf/sql-cli-defaults.yaml实现永久catalog。但测试的时候并未生效
[root@flink1 ~]# cat /root/flink-1.14.3/conf/sql-cli-defaults.yaml
catalogs:
- name: hadoop_catalog
type: iceberg
catalog-type: hadoop
property-version: 1
cache-enabled: true
warehouse: hdfs://nnha/user/iceberg/warehouse
[root@flink1 ~]#
[root@flink1 ~]# chown 501:games /root/flink-1.14.3/conf/sql-cli-defaults.yaml
下面我们重点以Hadoop Catalog为例,进行测试讲解
4.数据库和表相关DDL命令
4.1 创建数据库
Catalog下面默认都有一个default数据库
Flink SQL> create database hadoop_catalog.iceberg_db;
[INFO] Execute statement succeed.
Flink SQL> use hadoop_catalog.iceberg_db;
[INFO] Execute statement succeed.
Flink SQL>
会在HDFS目录上创建iceberg_db子目录 如果删除数据库,会删除HDFS上的iceberg_db子目录
4.2 创建表(不支持primary key等)
Flink SQL> create table hadoop_catalog.iceberg_db.my_user (
> user_id bigint comment '用户ID',
> user_name string,
> birthday date,
> country string
> ) comment '用户表'
> partitioned by (birthday, country) with (
> 'write.format.default'='parquet',
> 'write.parquet.compression-codec'='gzip'
> );
[INFO] Execute statement succeed.
Flink SQL>
目前表不支持计算列、primay key, Watermark 不支持计算分区。但是iceberg支持计算分区 因为Iceberg支持primary key。设置属性 'format-version' = '2'和'write.upsert.enabled' = 'true'
,同时表添加primary key,也是可以支持upsert的。可以实现insert、update、delete的功能创建表生成的文件信息如下:
[root@flink1 ~]#
[root@flink1 ~]# hadoop fs -ls hdfs://nnha/user/iceberg/warehouse/iceberg_db/my_user/metadata
Found 2 items
-rw-r--r-- 1 root supergroup 2115 2022-02-13 22:01 hdfs://nnha/user/iceberg/warehouse/iceberg_db/my_user/metadata/v1.metadata.json
-rw-r--r-- 1 root supergroup 1 2022-02-13 22:01 hdfs://nnha/user/iceberg/warehouse/iceberg_db/my_user/metadata/version-hint.text
[root@flink1 ~]#
查看v1.metadata.json,可以看到"current-snapshot-id" : -1
Flink SQL> create table hadoop_catalog.iceberg_db.my_user_copy
> like hadoop_catalog.iceberg_db.my_user;
[INFO] Execute statement succeed.
Flink SQL>
复制的表拥有相同的表结构、分区、表属性
4.3 修改表
修改表属性
Flink SQL> alter table hadoop_catalog.iceberg_db.my_user_copy
> set(
> 'write.format.default'='avro',
> 'write.avro.compression-codec'='gzip'
> );
[INFO] Execute statement succeed.
Flink SQL>
目前Flink只支持修改iceberg的表属性
重命名表
Flink SQL> alter table hadoop_catalog.iceberg_db.my_user_copy
> rename to hadoop_catalog.iceberg_db.my_user_copy_new;
[ERROR] Could not execute SQL statement. Reason:
java.lang.UnsupportedOperationException: Cannot rename Hadoop tables
Flink SQL>
Hadoop Catalog中的表不支持重命名表
4.4 删除表
Flink SQL> drop table hadoop_catalog.iceberg_db.my_user_copy;
[INFO] Execute statement succeed.
Flink SQL>
会删除HDFS上的my_user_copy子目录
5.插入数据到表
5.1 insert into
insert into … values … insert into … select …
Flink SQL> insert into hadoop_catalog.iceberg_db.my_user(
> user_id, user_name, birthday, country
> ) values(1, 'zhang_san', date '2022-02-01', 'china'),
> (2, 'li_si', date '2022-02-02', 'japan');
[INFO] Submitting SQL update statement to the cluster...
[INFO] SQL update statement has been successfully submitted to the cluster:
Job ID: f1aa8bee0be5bda8b166cc361e113268
Flink SQL>
Flink SQL> insert into hadoop_catalog.iceberg_db.my_user select (user_id + 1), user_name, birthday, country from hadoop_catalog.iceberg_db.my_user;
[INFO] Submitting SQL update statement to the cluster...
[INFO] SQL update statement has been successfully submitted to the cluster:
Job ID: c408e324ca3861b39176c6bd15770aca
Flink SQL>
HDFS目录结果如下
hdfs://nnha/user/iceberg/warehouse/iceberg_db/my_user/data/birthday=2022-02-01/country=china/00000-0-4ef3835f-b18b-4c48-b47a-85af1771a10a-00001.parquet
hdfs://nnha/user/iceberg/warehouse/iceberg_db/my_user/data/birthday=2022-02-01/country=china/00000-0-6e66c02b-cb09-4fd0-b669-15aa7f5194e4-00001.parquet
hdfs://nnha/user/iceberg/warehouse/iceberg_db/my_user/data/birthday=2022-02-02/country=japan/00000-0-4ef3835f-b18b-4c48-b47a-85af1771a10a-00002.parquet
hdfs://nnha/user/iceberg/warehouse/iceberg_db/my_user/data/birthday=2022-02-02/country=japan/00000-0-6e66c02b-cb09-4fd0-b669-15aa7f5194e4-00002.parquet
5.2 insert overwrite(只有Batch模式支持,且overwrite粒度为partition)
只支持Flink Batch模式,不支持Streaming模式
insert overwrite替换多个整个分区,而不是一行数据。如果不是分区表,则替换的是整个表,如下所示:
Flink SQL> set 'execution.runtime-mode' = 'batch';
[INFO] Session property has been set.
Flink SQL>
Flink SQL> insert overwrite hadoop_catalog.iceberg_db.my_user values (4, 'wang_wu', date '2022-02-02', 'japan');
[INFO] Submitting SQL update statement to the cluster...
[INFO] SQL update statement has been successfully submitted to the cluster:
Job ID: 63cf6c27060ec9ebdce75b785cc3fa3a
Flink SQL> set 'sql-client.execution.result-mode' = 'tableau';
[INFO] Session property has been set.
Flink SQL> select * from hadoop_catalog.iceberg_db.my_user;
+---------+-----------+------------+---------+
| user_id | user_name | birthday | country |
+---------+-----------+------------+---------+
| 1 | zhang_san | 2022-02-01 | china |
| 4 | wang_wu | 2022-02-02 | japan |
| 2 | zhang_san | 2022-02-01 | china |
+---------+-----------+------------+---------+
3 rows in set
birthday=2022-02-02/country=japan分区下的数据如下,insert overwrite也是新增一个文件
birthday=2022-02-02/country=japan/00000-0-1d0ff907-60a7-4062-93a3-9b443626e383-00001.parquet
birthday=2022-02-02/country=japan/00000-0-4ef3835f-b18b-4c48-b47a-85af1771a10a-00002.parquet
birthday=2022-02-02/country=japan/00000-0-6e66c02b-cb09-4fd0-b669-15aa7f5194e
insert ovewrite … partition替换指定分区
Flink SQL> insert overwrite hadoop_catalog.iceberg_db.my_user partition (birthday = '2022-02-02', country = 'japan') select 5, 'zhao_liu';
[INFO] Submitting SQL update statement to the cluster...
[INFO] SQL update statement has been successfully submitted to the cluster:
Job ID: 97e9ba4131028c53461e739b34108ae0
Flink SQL> select * from hadoop_catalog.iceberg_db.my_user;
+---------+-----------+------------+---------+
| user_id | user_name | birthday | country |
+---------+-----------+------------+---------+
| 1 | zhang_san | 2022-02-01 | china |
| 5 | zhao_liu | 2022-02-02 | japan |
| 2 | zhang_san | 2022-02-01 | china |
+---------+-----------+------------+---------+
3 rows in set
Flink SQL>
6.查询数据
Batch模式
Flink SQL> select * from hadoop_catalog.iceberg_db.my_user;
+---------+-----------+------------+---------+
| user_id | user_name | birthday | country |
+---------+-----------+------------+---------+
| 1 | zhang_san | 2022-02-01 | china |
| 5 | zhao_liu | 2022-02-02 | japan |
| 2 | zhang_san | 2022-02-01 | china |
+---------+-----------+------------+---------+
3 rows in set
Flink SQL>
streaming模式
查看最新的snapshot-id
[root@flink1 conf]# hadoop fs -cat hdfs://nnha/user/iceberg/warehouse/iceberg_db/my_user/metadata/version-hint.text
5
我们前面创建表 + 两次insert + 两次insert overwrite,所以最新的版本号为5。然后我们查看该版本号对于的metadata json文件
[root@flink1 ~]# hadoop fs -cat hdfs://nnha/user/iceberg/warehouse/iceberg_db/my_user/metadata/v5.metadata.json
{
"format-version" : 1,
"table-uuid" : "84a5e90d-7ae9-4dfd-aeab-c74f07447513",
"location" : "hdfs://nnha/user/iceberg/warehouse/iceberg_db/my_user",
"last-updated-ms" : 1644761481488,
"last-column-id" : 4,
"schema" : {
"type" : "struct",
"schema-id" : 0,
"fields" : [ {
"id" : 1,
"name" : "user_id",
"required" : false,
"type" : "long"
}, {
"id" : 2,
"name" : "user_name",
"required" : false,
"type" : "string"
}, {
"id" : 3,
"name" : "birthday",
"required" : false,
"type" : "date"
}, {
"id" : 4,
"name" : "country",
"required" : false,
"type" : "string"
} ]
},
"current-schema-id" : 0,
"schemas" : [ {
"type" : "struct",
"schema-id" : 0,
"fields" : [ {
"id" : 1,
"name" : "user_id",
"required" : false,
"type" : "long"
}, {
"id" : 2,
"name" : "user_name",
"required" : false,
"type" : "string"
}, {
"id" : 3,
"name" : "birthday",
"required" : false,
"type" : "date"
}, {
"id" : 4,
"name" : "country",
"required" : false,
"type" : "string"
} ]
} ],
"partition-spec" : [ {
"name" : "birthday",
"transform" : "identity",
"source-id" : 3,
"field-id" : 1000
}, {
"name" : "country",
"transform" : "identity",
"source-id" : 4,
"field-id" : 1001
} ],
"default-spec-id" : 0,
"partition-specs" : [ {
"spec-id" : 0,
"fields" : [ {
"name" : "birthday",
"transform" : "identity",
"source-id" : 3,
"field-id" : 1000
}, {
"name" : "country",
"transform" : "identity",
"source-id" : 4,
"field-id" : 1001
} ]
} ],
"last-partition-id" : 1001,
"default-sort-order-id" : 0,
"sort-orders" : [ {
"order-id" : 0,
"fields" : [ ]
} ],
"properties" : {
"write.format.default" : "parquet",
"write.parquet.compression-codec" : "gzip"
},
"current-snapshot-id" : 138573494821828246,
"snapshots" : [ {
"snapshot-id" : 8012517928892530314,
"timestamp-ms" : 1644761130111,
"summary" : {
"operation" : "append",
"flink.job-id" : "8f228ae49d34aafb4b2887db3149e3f6",
"flink.max-committed-checkpoint-id" : "9223372036854775807",
"added-data-files" : "2",
"added-records" : "2",
"added-files-size" : "2487",
"changed-partition-count" : "2",
"total-records" : "2",
"total-files-size" : "2487",
"total-data-files" : "2",
"total-delete-files" : "0",
"total-position-deletes" : "0",
"total-equality-deletes" : "0"
},
"manifest-list" : "hdfs://nnha/user/iceberg/warehouse/iceberg_db/my_user/metadata/snap-8012517928892530314-1-5c33451b-48ab-4ce5-be7a-2c2d2dc9e11d.avro",
"schema-id" : 0
}, {
"snapshot-id" : 453371561664052237,
"parent-snapshot-id" : 8012517928892530314,
"timestamp-ms" : 1644761150082,
"summary" : {
"operation" : "append",
"flink.job-id" : "813b7a17c21ddd003e1a210b1366e0c5",
"flink.max-committed-checkpoint-id" : "9223372036854775807",
"added-data-files" : "2",
"added-records" : "2",
"added-files-size" : "2487",
"changed-partition-count" : "2",
"total-records" : "4",
"total-files-size" : "4974",
"total-data-files" : "4",
"total-delete-files" : "0",
"total-position-deletes" : "0",
"total-equality-deletes" : "0"
},
"manifest-list" : "hdfs://nnha/user/iceberg/warehouse/iceberg_db/my_user/metadata/snap-453371561664052237-1-bc0e56ec-9f78-4956-8412-4d8ca70ccc19.avro",
"schema-id" : 0
}, {
"snapshot-id" : 6410282459040239217,
"parent-snapshot-id" : 453371561664052237,
"timestamp-ms" : 1644761403566,
"summary" : {
"operation" : "overwrite",
"replace-partitions" : "true",
"flink.job-id" : "f7085f68e5ff73c1c8aa1f4f59996068",
"flink.max-committed-checkpoint-id" : "9223372036854775807",
"added-data-files" : "1",
"deleted-data-files" : "2",
"added-records" : "1",
"deleted-records" : "2",
"added-files-size" : "1244",
"removed-files-size" : "2459",
"changed-partition-count" : "1",
"total-records" : "3",
"total-files-size" : "3759",
"total-data-files" : "3",
"total-delete-files" : "0",
"total-position-deletes" : "0",
"total-equality-deletes" : "0"
},
"manifest-list" : "hdfs://nnha/user/iceberg/warehouse/iceberg_db/my_user/metadata/snap-6410282459040239217-1-2b20c57e-5428-4483-9f7b-928b980dd50d.avro",
"schema-id" : 0
}, {
"snapshot-id" : 138573494821828246,
"parent-snapshot-id" : 6410282459040239217,
"timestamp-ms" : 1644761481488,
"summary" : {
"operation" : "overwrite",
"replace-partitions" : "true",
"flink.job-id" : "d434d6d4f658d61732d7e9a0a85279fc",
"flink.max-committed-checkpoint-id" : "9223372036854775807",
"added-data-files" : "1",
"deleted-data-files" : "1",
"added-records" : "1",
"deleted-records" : "1",
"added-files-size" : "1251",
"removed-files-size" : "1244",
"changed-partition-count" : "1",
"total-records" : "3",
"total-files-size" : "3766",
"total-data-files" : "3",
"total-delete-files" : "0",
"total-position-deletes" : "0",
"total-equality-deletes" : "0"
},
"manifest-list" : "hdfs://nnha/user/iceberg/warehouse/iceberg_db/my_user/metadata/snap-138573494821828246-1-b243b39e-7122-4571-b6fa-c902241e36a8.avro",
"schema-id" : 0
} ],
"snapshot-log" : [ {
"timestamp-ms" : 1644761130111,
"snapshot-id" : 8012517928892530314
}, {
"timestamp-ms" : 1644761150082,
"snapshot-id" : 453371561664052237
}, {
"timestamp-ms" : 1644761403566,
"snapshot-id" : 6410282459040239217
}, {
"timestamp-ms" : 1644761481488,
"snapshot-id" : 138573494821828246
} ],
"metadata-log" : [ {
"timestamp-ms" : 1644760911017,
"metadata-file" : "hdfs://nnha/user/iceberg/warehouse/iceberg_db/my_user/metadata/v1.metadata.json"
}, {
"timestamp-ms" : 1644761130111,
"metadata-file" : "hdfs://nnha/user/iceberg/warehouse/iceberg_db/my_user/metadata/v2.metadata.json"
}, {
"timestamp-ms" : 1644761150082,
"metadata-file" : "hdfs://nnha/user/iceberg/warehouse/iceberg_db/my_user/metadata/v3.metadata.json"
}, {
"timestamp-ms" : 1644761403566,
"metadata-file" : "hdfs://nnha/user/iceberg/warehouse/iceberg_db/my_user/metadata/v4.metadata.json"
} ]
}[root@flink1 ~]#
可以看到 "current-snapshot-id" : 138573494821828246,
,表示当前的snapshot-id
Flink SQL> set 'execution.runtime-mode' = 'streaming';
[INFO] Session property has been set.
Flink SQL>
Flink SQL> select * from hadoop_catalog.iceberg_db.my_user
> /*+ options(
> 'streaming'='true',
> 'monitor-interval'='5s'
> )*/ ;
+----+----------------------+--------------------------------+------------+--------------------------------+
| op | user_id | user_name | birthday | country |
+----+----------------------+--------------------------------+------------+--------------------------------+
| +I | 5 | zhao_liu | 2022-02-02 | japan |
| +I | 2 | zhang_san | 2022-02-01 | china |
| +I | 1 | zhang_san | 2022-02-01 | china |
可以看到最新snapshot对应的数据
Flink SQL> select * from hadoop_catalog.iceberg_db.my_user
> /*+ options(
> 'streaming'='true',
> 'monitor-interval'='5s',
> 'start-snapshot-id'='138573494821828246'
> )*/ ;
+----+----------------------+--------------------------------+------------+--------------------------------+
| op | user_id | user_name | birthday | country |
+----+----------------------+--------------------------------+------------+--------------------------------+
这里只能指定最后一个insert overwrite操作的snapshot id,及其后面的snapshot id,否则后台会报异常,且程序一直处于restarting的状态:
java.lang.UnsupportedOperationException: Found overwrite operation, cannot support incremental data in snapshots (8012517928892530314, 138573494821828246]
在本示例中snapshot id: 138573494821828246,是最后一个snapshot id,同时也是最后一个insert overwrite操作的snapshot id。如果再insert两条数据,则只能看到增量的数据
Flink SQL> insert into hadoop_catalog.iceberg_db.my_user(
> user_id, user_name, birthday, country
> ) values(6, 'zhang_san', date '2022-02-01', 'china');
[INFO] Submitting SQL update statement to the cluster...
[INFO] SQL update statement has been successfully submitted to the cluster:
Job ID: 8eb279e61aed66304d78ad027eaf8d30
Flink SQL> insert into hadoop_catalog.iceberg_db.my_user(
> user_id, user_name, birthday, country
> ) values(7, 'zhang_san', date '2022-02-01', 'china');
[INFO] Submitting SQL update statement to the cluster...
[INFO] SQL update statement has been successfully submitted to the cluster:
Job ID: 70a050e455d188d0d3f3adc2ba367fb6
Flink SQL> select * from hadoop_catalog.iceberg_db.my_user
> /*+ options(
> 'streaming'='true',
> 'monitor-interval'='30s',
> 'start-snapshot-id'='138573494821828246'
> )*/ ;
+----+----------------------+--------------------------------+------------+--------------------------------+
| op | user_id | user_name | birthday | country |
+----+----------------------+--------------------------------+------------+--------------------------------+
| +I | 6 | zhang_san | 2022-02-01 | china |
| +I | 7 | zhang_san | 2022-02-01 | china |
streaming模式支持读取增量snapshot数据 如果不指定start-snapshot-id,则先读取当前snapshot全量数据,再读取增量数据。如果指定start-snapshot-id,读取该snapshot-id之后的增量数据,即不读取该snapshot-id的数据 monitor-interval:表示监控新提交的数据文件的时间间隔,默认1s
