大数据入门:Spark+Kudu的广告业务项目实战笔记(四)
程序源代码
共 4766字,需浏览 10分钟
·
2020-08-22 13:01
点击上方蓝色字体,选择“设为星标”
1.统计需求
完成统计地域分布情况,需要原始请求数、有效请求数、广告请求数、参与竞价数、竞价成功数、广告主展示数、广告主点击数、媒介展示数、媒介点击数、DSP广告消费数、DSP广告成本数。具体指标如下所示:
2.代码编写
先做第一步处理,按上述要求将数据提取出来放在Kudu里。
package com.imooc.bigdata.cp08.business
import com.imooc.bigdata.cp08.`trait`.DataProcess
import com.imooc.bigdata.cp08.utils.{KuduUtils, SQLUtils, SchemaUtils}
import org.apache.spark.sql.SparkSession
object AreaStatProcessor extends DataProcess{
override def process(spark: SparkSession): Unit = {
val sourceTableName = "ods"
val masterAddresses = "hadoop000"
val odsDF = spark.read.format("org.apache.kudu.spark.kudu")
.option("kudu.table",sourceTableName)
.option("kudu.master",masterAddresses)
.load()
odsDF.createOrReplaceTempView("ods")
val resultTmp = spark.sql(SQLUtils.AREA_SQL_STEP1)
resultTmp.show()
}
}
SQL语句较长:
lazy val AREA_SQL_STEP1 = "select provincename,cityname, " +
"sum(case when requestmode=1 and processnode >=1 then 1 else 0 end) origin_request," +
"sum(case when requestmode=1 and processnode >=2 then 1 else 0 end) valid_request," +
"sum(case when requestmode=1 and processnode =3 then 1 else 0 end) ad_request," +
"sum(case when adplatformproviderid>=100000 and iseffective=1 and isbilling=1 and isbid=1 and adorderid!=0 then 1 else 0 end) bid_cnt," +
"sum(case when adplatformproviderid>=100000 and iseffective=1 and isbilling=1 and iswin=1 then 1 else 0 end) bid_success_cnt," +
"sum(case when requestmode=2 and iseffective=1 then 1 else 0 end) ad_display_cnt," +
"sum(case when requestmode=3 and processnode=1 then 1 else 0 end) ad_click_cnt," +
"sum(case when requestmode=2 and iseffective=1 and isbilling=1 then 1 else 0 end) medium_display_cnt," +
"sum(case when requestmode=3 and iseffective=1 and isbilling=1 then 1 else 0 end) medium_click_cnt," +
"sum(case when adplatformproviderid>=100000 and iseffective=1 and isbilling=1 and iswin=1 and adorderid>20000 then 1*winprice/1000 else 0 end) ad_consumption," +
"sum(case when adplatformproviderid>=100000 and iseffective=1 and isbilling=1 and iswin=1 and adorderid>20000 then 1*adpayment/1000 else 0 end) ad_cost " +
"from ods group by provincename,cityname"
在入口里调用:
AreaStatProcessor.process(spark)
本地查看输出是否符合预期:
若符合预期,将此表保存为area_temp并进行第二阶段的SQL编写,求出bid_success_rate、ad_click_rate等百分比,这里要注意过滤除数为0的情况:
lazy val AREA_SQL_STEP2 = "select provincename,cityname, " +
"origin_request," +
"valid_request," +
"ad_request," +
"bid_cnt," +
"bid_success_cnt," +
"bid_success_cnt/bid_cnt bid_success_rate," +
"ad_display_cnt," +
"ad_click_cnt," +
"ad_click_cnt/ad_display_cnt ad_click_rate," +
"ad_consumption," +
"ad_cost from area_tmp " +
"where bid_cnt!=0 and ad_display_cnt!=0"
resultTmp.createOrReplaceTempView("area_tmp")
val result = spark.sql(SQLUtils.AREA_SQL_STEP2)
result.show()
结果如下:
3.落地Kudu
如果之前结果OK的话,就可以把它上传到Kudu里,其中schema编写如下,需要一一对应:
lazy val AREASchema: Schema = {
val columns = List(
new ColumnSchemaBuilder("provincename",Type.STRING).nullable(false).key(true).build(),
new ColumnSchemaBuilder("cityname",Type.STRING).nullable(false).key(true).build(),
new ColumnSchemaBuilder("origin_request",Type.INT64).nullable(false).build(),
new ColumnSchemaBuilder("valid_request",Type.INT64).nullable(false).build(),
new ColumnSchemaBuilder("ad_request",Type.INT64).nullable(false).build(),
new ColumnSchemaBuilder("bid_cnt",Type.INT64).nullable(false).build(),
new ColumnSchemaBuilder("bid_success_cnt",Type.INT64).nullable(false).build(),
new ColumnSchemaBuilder("bid_success_rate",Type.DOUBLE).nullable(false).build(),
new ColumnSchemaBuilder("ad_display_cnt",Type.INT64).nullable(false).build(),
new ColumnSchemaBuilder("ad_click_cnt",Type.INT64).nullable(false).build(),
new ColumnSchemaBuilder("ad_click_rate",Type.DOUBLE).nullable(false).build(),
new ColumnSchemaBuilder("ad_consumption",Type.DOUBLE).nullable(false).build(),
new ColumnSchemaBuilder("ad_cost",Type.DOUBLE).nullable(false).build()
).asJava
val sinkTableName = "area_stat"
val partitionId = "provincename"
val schema = SchemaUtils.AREASchema
KuduUtils.sink(result,sinkTableName,masterAddresses,schema,partitionId)
spark.read.format("org.apache.kudu.spark.kudu")
.option("kudu.master",masterAddresses)
.option("kudu.table",sinkTableName)
.load().show()
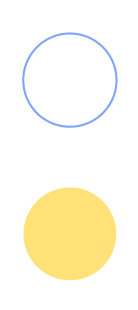
版权声明:
本文为大数据技术与架构整理,原作者独家授权。未经原作者允许转载追究侵权责任。
本文编辑:冷眼丶
微信公众号|import_bigdata
文章不错?点个【在看】吧! ?
评论