OpenCV4.5.1 | 使用一行代码将图像匹配性能提高14%
点击上方“AI算法与图像处理”,选择加"星标"或“置顶”
重磅干货,第一时间送达
https://github.com/iago-suarez/beblid-opencv-demo/blob/main/demo.ipynb
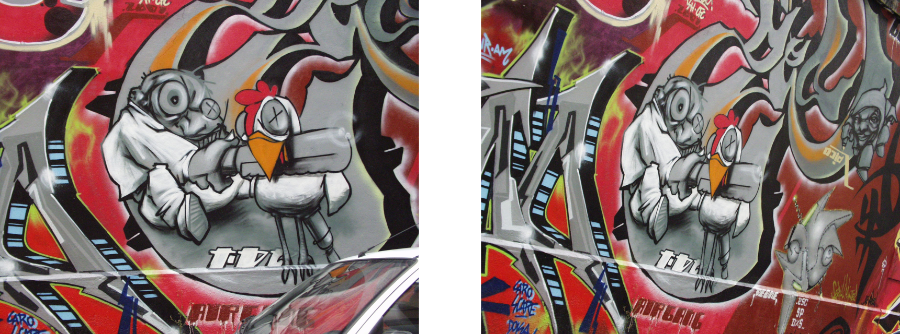
pip install "opencv-contrib-python>=4.5.1"
python
>>> import cv2 as cv
>>> print(f"OpenCV Version: {cv.__version__}")
OpenCV Version: 4.5.1
import cv2 as cv
# Load grayscale images
img1 = cv.imread("graf1.png", cv.IMREAD_GRAYSCALE)
img2 = cv.imread("graf3.png", cv.IMREAD_GRAYSCALE)
if img1 is None or img2 is None:
print('Could not open or find the images!')
exit(0)
# Load homography (geometric transformation between image)
fs = cv.FileStorage("H1to3p.xml", cv.FILE_STORAGE_READ)
homography = fs.getFirstTopLevelNode().mat()
print(f"Homography from img1 to img2:\n{homography}")
detector = cv.ORB_create(10000)
kpts1 = detector.detect(img1, None)
kpts2 = detector.detect(img2, None)
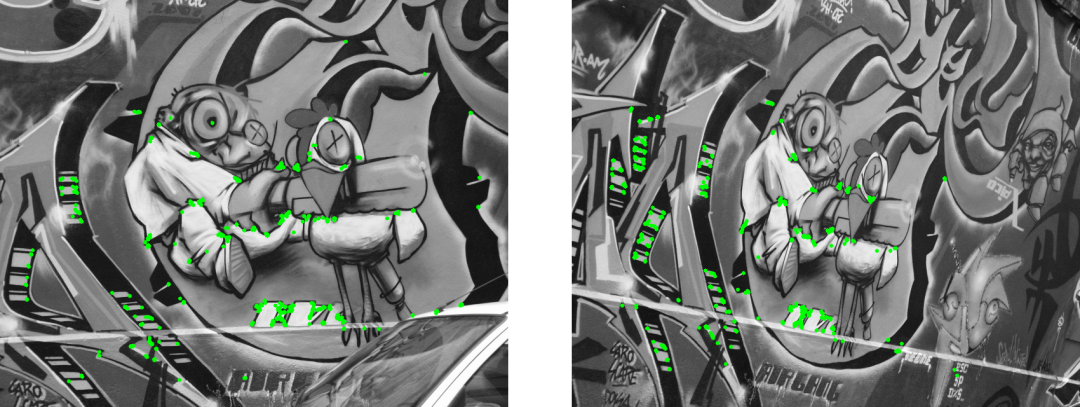
ORB(Oriented FAST and Rotated BRIEF):一个经典的替代品,已经有10年的历史了,效果相当不错。 BEBLID(Boosted effective Binary Local Image Descriptor):2020年推出的一种新的描述符,在多个任务中被证明可以提高ORB。由于BEBLID适用于多种检测方法,因此必须将ORB关键点的比例设置为0.75~1。
# Comment or uncomment to use ORB or BEBLID
descriptor = cv.xfeatures2d.BEBLID_create(0.75)
# descriptor = cv.ORB_create()
kpts1, desc1 = descriptor.compute(img1, kpts1)
kpts2, desc2 = descriptor.compute(img2, kpts2)
matcher = cv.DescriptorMatcher_create(cv.DescriptorMatcher_BRUTEFORCE_HAMMING)
nn_matches = matcher.knnMatch(desc1, desc2, 2)
matched1 = []
matched2 = []
nn_match_ratio = 0.8 # Nearest neighbor matching ratio
for m, n in nn_matches:
if m.distance < nn_match_ratio * n.distance:
matched1.append(kpts1[m.queryIdx])
matched2.append(kpts2[m.trainIdx])
inliers1 = []
inliers2 = []
good_matches = []
inlier_threshold = 2.5 # Distance threshold to identify inliers with homography check
for i, m in enumerate(matched1):
# Create the homogeneous point
col = np.ones((3, 1), dtype=np.float64)
col[0:2, 0] = m.pt
# Project from image 1 to image 2
col = np.dot(homography, col)
col /= col[2, 0]
# Calculate euclidean distance
dist = sqrt(pow(col[0, 0] - matched2[i].pt[0], 2) + pow(col[1, 0] - matched2[i].pt[1], 2))
if dist < inlier_threshold:
good_matches.append(cv.DMatch(len(inliers1), len(inliers2), 0))
inliers1.append(matched1[i])
inliers2.append(matched2[i])
单应性估计:https://docs.opencv.org/4.5.1/d9/d0c/group__calib3d.html#ga4abc2ece9fab9398f2e560d53c8c9780 透视n点:https://docs.opencv.org/4.5.1/d9/d0c/group__calib3d.html#ga549c2075fac14829ff4a58bc931c033d 平面跟踪:https://docs.opencv.org/4.5.1/dc/d16/tutorial_akaze_tracking.html 实时姿态估计:https://docs.opencv.org/4.5.1/dc/d2c/tutorial_real_time_pose.html 图像拼接:https://docs.opencv.org/4.5.1/df/d8c/group__stitching__match.html
res = np.empty((max(img1.shape[0], img2.shape[0]), img1.shape[1] + img2.shape[1], 3), dtype=np.uint8)
cv.drawMatches(img1, inliers1, img2, inliers2, good_matches, res)
plt.figure(figsize=(15, 5))
plt.imshow(res)
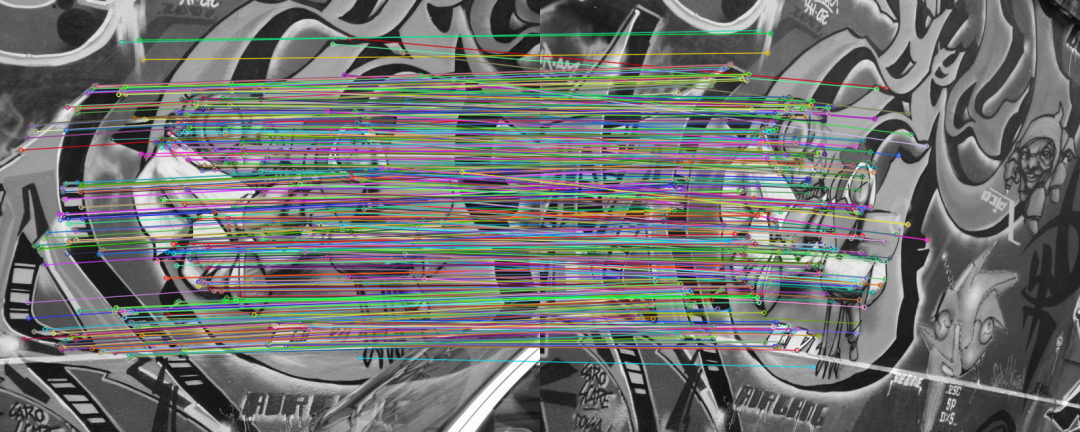

Matching Results (BEBLID)
*******************************
# Keypoints 1: 9105
# Keypoints 2: 9927
# Matches: 660
# Inliers: 512
# Percentage of Inliers: 77.57%
# Comment or uncomment to use ORB or BEBLID
# descriptor = cv.xfeatures2d.BEBLID_create(0.75)
descriptor = cv.ORB_create()
kpts1, desc1 = descriptor.compute(img1, kpts1)
kpts2, desc2 = descriptor.compute(img2, kpts2)
Matching Results (ORB)
*******************************
# Keypoints 1: 9105
# Keypoints 2: 9927
# Matches: 780
# Inliers: 493
# Percentage of Inliers: 63.20%
个人微信(如果没有备注不拉群!) 请注明:地区+学校/企业+研究方向+昵称
下载1:何恺明顶会分享
在「AI算法与图像处理」公众号后台回复:何恺明,即可下载。总共有6份PDF,涉及 ResNet、Mask RCNN等经典工作的总结分析
下载2:终身受益的编程指南:Google编程风格指南
在「AI算法与图像处理」公众号后台回复:c++,即可下载。历经十年考验,最权威的编程规范!
下载3 CVPR2021 在「AI算法与图像处理」公众号后台回复:CVPR,即可下载1467篇CVPR 2020论文 和 CVPR 2021 最新论文
点亮 ,告诉大家你也在看
评论