PyTorch 源码解读之分布式训练了解一下?
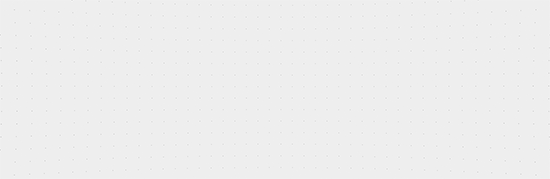
极市导读
本文由浅入深讲解 torch.distributed 这一并行计算包的概念,实现细节和应用方式,并带大家快速入门 PyTorch 分布式训练。 >>加入极市CV技术交流群,走在计算机视觉的最前沿
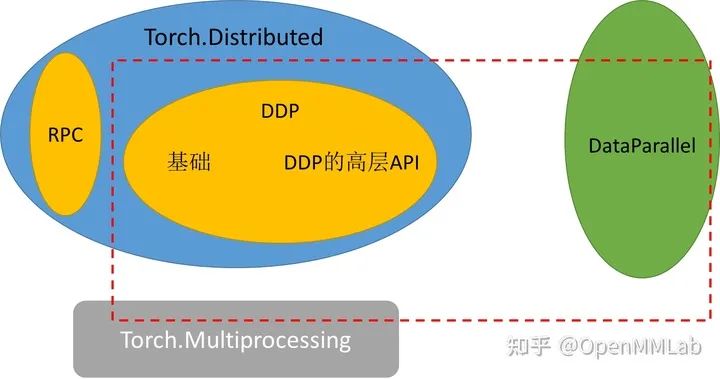
0 前言
由于大规模机器学习的广泛普及,超大型深度学习模型的提出,联邦学习等分布式学习方法的快速发展,分布式机器学习模型训练与部署技术已经日益成为研究者和开发者的必备技术。PyTorch 作为应用最为广泛的深度学习框架,也发展出了一套分布式学习的解决方法。本文由浅入深讲解 torch.distributed 这一并行计算包的概念,实现细节和应用方式,并带大家快速入门 PyTorch 分布式训练。
1 Torch.distributed 概念与定义
定义:首先我们提供 Torch.distributed 的官方定义
torch.distributed 包为运行在一台或多台机器上的多个计算节点之间的 PyTorch 提供支持多进程并行性通信的原语。他能轻松地并行化在跨进程和机器集群的计算。 torch.nn.parallel.DistributedDataParalle(DDP) 是建立在此功能的基础上,以提供同步的分布式训练作为任何 PyTorch 模型的包装器。
可以注意到的是,torch.distributed 的核心功能是进行多进程级别的通信(而非多线程),以此达到多卡多机分布式训练的目的。这与基于 DataParrallel 的多线程训练有明显区别。
通信方式:torch.distributed 的底层通信主要使用 Collective Communication (c10d) library 来支持跨组内的进程发送张量,并主要支持两种类型的通信 API:
collective communication APIs: Distributed Data-Parallel Training (DDP) P2P communication APIs: RPC-Based Distributed Training (RPC)
这两种通信 API 在 PyTorch 中分别对应了两种分布式训练方式:Distributed Data-Parallel Training (DDP) 和 RPC-Based Distributed Training (RPC)。本文着重探讨 Distributed Data-Parallel Training (DDP) 的通信方式和 API
基础概念: 下面介绍一些 torch.distributed 中的关键概念以供参考。这些概念在编写程序时至关重要
Group(进程组)是我们所有进程的子集。 Backend(后端)进程通信库。PyTorch 支持 NCCL,GLOO,MPI。本文不展开讲几种通信后端的区别,感兴趣的同学可以参考官方文档 world_size(世界大小)在进程组中的进程数。 Rank(秩)分配给分布式进程组中每个进程的唯一标识符。它们始终是从 0 到 world_size 的连续整数。
2 Torch.distributed 实例
例子 1:初始化
"""run.py:"""
#!/usr/bin/env python
import os
import torch
import torch.distributed as dist
from torch.multiprocessing import Process
def run(rank, size):
""" Distributed function to be implemented later. """
pass
def init_process(rank, size, fn, backend='gloo'):
""" Initialize the distributed environment. """
os.environ['MASTER_ADDR'] = '127.0.0.1'
os.environ['MASTER_PORT'] = '29500'
dist.init_process_group(backend, rank=rank, world_size=size)
fn(rank, size)
if __name__ == "__main__":
size = 2
processes = []
for rank in range(size):
p = Process(target=init_process, args=(rank, size, run))
p.start()
processes.append(p)
for p in processes:
p.join()
本段程序执行了下面三件事
创建了两个进程 分别加入一个进程组 分别运行 run 函数。此时 run 是一个空白函数,之后的例子会扩充这个函数的内容并在函数内完成多进程的通信操作。
例子 2:点对点通信
最简单的多进程通信方式是点对点通信。信息从一个进程被发送到另一个进程。
def run(rank, size):
tensor = torch.zeros(1)
if rank == 0:
tensor += 1
# Send the tensor to process 1
dist.send(tensor=tensor, dst=1)
else:
# Receive tensor from process 0
dist.recv(tensor=tensor, src=0)
print('Rank ', rank, ' has data ', tensor[0])
在上面的示例中,两个进程都从 tensor(0) 开始,然后进程 0 递增张量并将其发送到进程 1,以便它们都以 tensor(1) 结尾。请注意,进程 1 需要分配内存以存储它将接收的数据。
另请注意,send / recv 被阻塞:两个过程都停止,直到通信完成。我们还有另外一种无阻塞的通信方式,请看下例
"""Non-blocking point-to-point communication."""
def run(rank, size):
tensor = torch.zeros(1)
req = None
if rank == 0:
tensor += 1
# Send the tensor to process 1
req = dist.isend(tensor=tensor, dst=1)
print('Rank 0 started sending')
else:
# Receive tensor from process 0
req = dist.irecv(tensor=tensor, src=0)
print('Rank 1 started receiving')
req.wait()
print('Rank ', rank, ' has data ', tensor[0])
我们通过调用 wait 函数以使自己在子进程执行过程中保持休眠状态。由于我们不知道何时将数据传递给其他进程,因此在 req.wait() 完成之前,我们既不应该修改发送的张量也不应该访问接收的张量以防止不确定的写入。
例子 3:进程组间通信
与点对点通信相反,集合允许跨组中所有进程的通信模式。例如,为了获得所有过程中所有张量的总和,我们可以使用 dist.all_reduce(tensor, op, group) 函数进行组间通信
""" All-Reduce example."""
def run(rank, size):
""" Simple point-to-point communication. """
group = dist.new_group([0, 1])
tensor = torch.ones(1)
dist.all_reduce(tensor, op=dist.reduce_op.SUM, group=group)
print('Rank ', rank, ' has data ', tensor[0])
这段代码首先将进程 0 和 1 组成进程组,然后将各自进程中 tensor(1) 相加。由于我们需要组中所有张量的总和,因此我们将 dist.reduce_op.SUM 用作化简运算符。一般来说,任何可交换的数学运算都可以用作运算符。PyTorch 开箱即用,带有 4 个这样的运算符,它们都在元素级运行:
dist.reduce_op.SUM dist.reduce_op.PRODUCT dist.reduce_op.MAX dist.reduce_op.MIN
除了 dist.all_reduce(tensor, op, group) 之外,PyTorch 中目前共有 6 种组间通信方式
distributed.scatter(tensor, scatter_list=None, src=0, group=None, async_op=False):将张量 scatter_list[i] 复制第 i 个进程的过程。例如,在实现分布式训练时,我们将数据分成四份并分别发送到不同的机子上计算梯度。scatter 函数可以用来将信息从 src 进程发送到其他进程上。
tensor | 发送的数据 |
scatter_list | 存储发送数据的列表(只需在 src 进程中指定) |
dst | 发送进程的rank |
group | 指定进程组 |
async_op | 该 op 是否是异步操作 |
distributed.gather(tensor, gather_list=None, dst=0, group=None, async_op=False):从 dst 中的所有进程复制 tensor。例如,在实现分布式训练时,不同进程计算得到的梯度需要汇总到一个进程,并计算平均值以获得统一的梯度。gather 函数可以将信息从别的进程汇总到 dst 进程。
tensor | 接受的数据 |
gather_list | 存储接受数据的列表(只需在dst进程中指定) |
dst | 汇总进程的rank |
group | 指定进程组 |
async_op | 该op是否是异步操作 |
distributed.reduce(tensor, dst, op, group):将 op 应用于所有 tensor,并将结果存储在 dst 中。
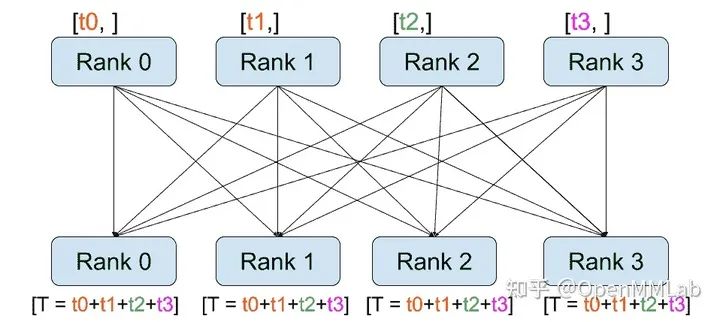
distributed.all_reduce(tensor, op, group):与 reduce 相同,但是结果存储在所有进程中。
distributed.broadcast(tensor, src, group):将tensor从src复制到所有其他进程。
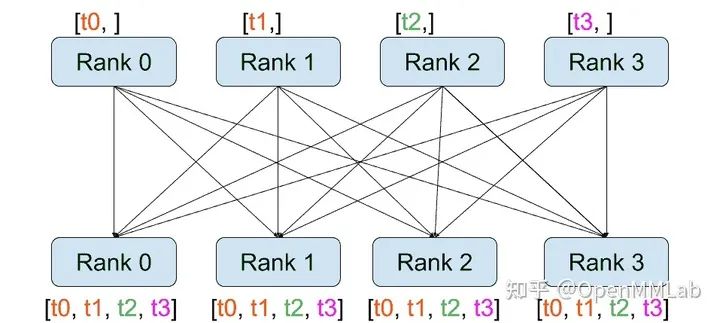
distributed.all_gather(tensor_list, tensor, group):将所有进程中的 tensor 从所有进程复制到 tensor_list
例子 4:分布式梯度下降
分布式梯度下降脚本将允许所有进程在其数据 batch 上计算其模型的梯度,然后平均其梯度。为了在更改进程数时确保相似的收敛结果,我们首先必须对数据集进行分区。
""" Dataset partitioning helper """
class Partition(object):
def __init__(self, data, index):
self.data = data
self.index = index
def __len__(self):
return len(self.index)
def __getitem__(self, index):
data_idx = self.index[index]
return self.data[data_idx]
class DataPartitioner(object):
def __init__(self, data, sizes=[0.7, 0.2, 0.1], seed=1234):
self.data = data
self.partitions = []
rng = Random()
rng.seed(seed)
data_len = len(data)
indexes = [x for x in range(0, data_len)]
rng.shuffle(indexes)
for frac in sizes:
part_len = int(frac * data_len)
self.partitions.append(indexes[0:part_len])
indexes = indexes[part_len:]
def use(self, partition):
return Partition(self.data, self.partitions[partition])
使用上面的代码片段,我们现在可以使用以下几行简单地对任何数据集进行分区
""" Partitioning MNIST """
def partition_dataset():
dataset = datasets.MNIST('./data', train=True, download=True,
transform=transforms.Compose([
transforms.ToTensor(),
transforms.Normalize((0.1307,), (0.3081,))
]))
size = dist.get_world_size()
bsz = 128 / float(size)
partition_sizes = [1.0 / size for _ in range(size)]
partition = DataPartitioner(dataset, partition_sizes)
partition = partition.use(dist.get_rank())
train_set = torch.utils.data.DataLoader(partition,
batch_size=bsz,
shuffle=True)
return train_set, bsz
假设我们有 2 个进程,则每个进程的 train_set 为 60000/2 = 30000 个样本。我们还将 batch 大小除以进程数,以使整体 batch 大小保持为 128。
现在,我们可以编写通常的向前-向后优化训练代码,并添加一个函数调用以平均模型的梯度。
""" Distributed Synchronous SGD Example """
def run(rank, size):
torch.manual_seed(1234)
train_set, bsz = partition_dataset()
model = Net()
optimizer = optim.SGD(model.parameters(),
lr=0.01, momentum=0.5)
num_batches = ceil(len(train_set.dataset) / float(bsz))
for epoch in range(10):
epoch_loss = 0.0
for data, target in train_set:
optimizer.zero_grad()
output = model(data)
loss = F.nll_loss(output, target)
epoch_loss += loss.item()
loss.backward()
average_gradients(model)
optimizer.step()
print('Rank ', dist.get_rank(), ', epoch ',
epoch, ': ', epoch_loss / num_batches)
仍然需要执行 average_gradients(model) 函数,该函数只需要一个模型并计算在所有 rank 上梯度的平均值。
""" Gradient averaging. """
def average_gradients(model):
size = float(dist.get_world_size())
for param in model.parameters():
dist.all_reduce(param.grad.data, op=dist.reduce_op.SUM)
param.grad.data /= size
3 PyTorch 并行/分布式训练
在掌握 torch.distributed 的基础的前提下,我们可以根据自身机器和任务的具体情况使用不同的分布式或并行训练方式:
如果数据和模型可以放在一个 GPU 中,并且不关心训练速度,请使用单设备训练。 如果单个服务器上有多个 GPU,并且您希望更改较少的代码来加快训练速度,请使用单机多 GPU DataParallel。 如果单个服务器上有多个 GPU,且您希望进一步添加代码并加快训练速度,请使用单机多 GPU DistributedDataParallel。 如果应用程序需要跨多个服务器,请使用多机 DistributedDataParallel 和启动脚本。 如果预计会出现错误(例如,OOM),或者在训练期间资源可以动态加入和离开,请使用 torch.elastic 进行分布式训练。
3.1 DataParallel
class torch.nn.DataParallel(module, device_ids=None, output_device=None, dim=0)
DataParallel 自动分割您的数据,并将作业订单发送到多个 GPU 上的多个模型。每个模型完成工作后,DataParallel 会收集并合并结果,然后再将结果返回给您。DataParallel 将相同的模型复制到所有 GPU,其中每个 GPU 消耗输入数据的不同分区。在使用此方法时,batch 理大小应大于使用的 GPU 数量。我们需要注意的是,DataParallel 是通过多线程的方式进行的并行训练,所以并没有使用 torch.distributed 里的线程通信 API。的其运行过程如下图所示
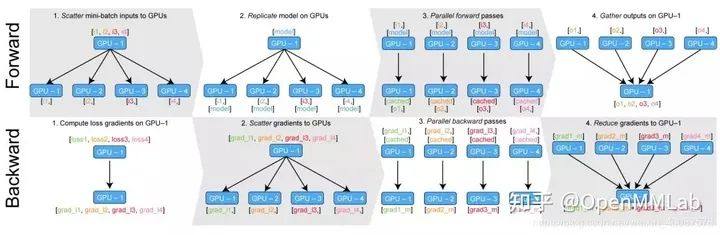
例子 5 DataParallel
创建 dump 数据集和定义模型
class RandomDataset(Dataset):
def __init__(self, size, length):
self.len = length
self.data = torch.randn(length, size)
def __getitem__(self, index):
return self.data[index]
def __len__(self):
return self.len
rand_loader = DataLoader(dataset=RandomDataset(input_size, data_size),
batch_size=batch_size, shuffle=True)
class Model(nn.Module):
# Our model
def __init__(self, input_size, output_size):
super(Model, self).__init__()
self.fc = nn.Linear(input_size, output_size)
def forward(self, input):
output = self.fc(input)
print("\tIn Model: input size", input.size(),
"output size", output.size())
return output
定义模型,放入设备并用 DataParallel 对象进行包装
model = Model(input_size, output_size)
if torch.cuda.device_count() > 1:
print("Let's use", torch.cuda.device_count(), "GPUs!")
# dim = 0 [30, xxx] -> [10, ...], [10, ...], [10, ...] on 3 GPUs
model = nn.DataParallel(model)
model.to(device)
运行模型并输出
for data in rand_loader:
input = data.to(device)
output = model(input)
input size", input.size(), :
output.size())
In Model: input size torch.Size([15, 5]) output size torch.Size([15, 2])
In Model: input size torch.Size([15, 5]) output size torch.Size([15, 2])
Outside: input size torch.Size([30, 5]) output_size torch.Size([30, 2])
In Model: input size torch.Size([15, 5]) output size torch.Size([15, 2])
In Model: input size torch.Size([15, 5]) output size torch.Size([15, 2])
Outside: input size torch.Size([30, 5]) output_size torch.Size([30, 2])
In Model: input size torch.Size([15, 5]) output size torch.Size([15, 2])
In Model: input size torch.Size([15, 5]) output size torch.Size([15, 2])
Outside: input size torch.Size([30, 5]) output_size torch.Size([30, 2])
In Model: input size torch.Size([5, 5]) output size torch.Size([5, 2])
In Model: input size torch.Size([5, 5]) output size torch.Size([5, 2])
Outside: input size torch.Size([10, 5]) output_size torch.Size([10, 2])
我们可以看到,在模型中,数据是按照batch大小的维度被均匀分成多份。在输出后,多块 GPU 上的数据进行合并。
3.2 DistributedDataParallel
当我们了解了 DataParallel 后,下面开始介绍一种基于 torch.distributed 中进程通信函数包装的高层 API
CLASS torch.nn.parallel.DistributedDataParallel(module, device_ids=None, output_device=None, dim=0, broadcast_buffers=True, process_group=None, bucket_cap_mb=**25**, find_unused_parameters=False, check_reduction=False, gradient_as_bucket_view=False)
既然 DataParallel 可以进行并行的模型训练,那么为什么还需要提出 DistributedDataParallel呢?这里我们就需要知道两种方法的实现原理与区别:
如果模型太大而无法容纳在单个 GPU 上,则必须使用模型并行将其拆分到多个 GPU 中。DistributedDataParallel 可以与模型并行一起使用;但 DataParallel 因为必须将模型放入单块 GPU 中,所以难以完成大型模型的训练。 DataParallel 是单进程,多线程的并行训练方式,并且只能在单台机器上运行,而DistributedDataParallel 是多进程,并且适用于单机和多机训练。DistributedDataParallel 还预先复制模型,而不是在每次迭代时复制模型,并避免了全局解释器锁定。 如果您的两个数据都太大而无法容纳在一台计算机和上,而您的模型又太大了以至于无法安装在单个 GPU 上,则可以将模型并行(跨多个 GPU 拆分单个模型)与 DistributedDataParallel 结合使用。在这种情况下,每个 DistributedDataParallel 进程都可以并行使用模型,而所有进程都将并行使用数据。
例子 6 DistributedDataParallel
首先我们需要创建一系列进程,其中需要用到 torch.multiprocessing 中的函数
torch.multiprocessing.spawn(fn, args=(), nprocs=1, join=True, daemon=False, start_method='spawn')
该函数使用 args 作为参数列表运行函数fn,并创建 nprocs 个进程。
如果其中一个进程以非零退出状态退出,则其余进程将被杀死,并引发异常,以终止原因。如果子进程中捕获到异常,则将其转发并将其回溯包括在父进程中引发的异常中。
该函数会通过 fn(i,args) 的形式被调用,其中i是进程索引,而 args 是传递的参数元组。
基于创建的的进程,我们初始化进程组
import os
import tempfile
import torch
import torch.distributed as dist
import torch.nn as nn
import torch.optim as optim
import torch.multiprocessing as mp
from torch.nn.parallel import DistributedDataParallel as DDP
def setup(rank, world_size):
os.environ['MASTER_ADDR'] = 'localhost'
os.environ['MASTER_PORT'] = '12355'
# initialize the process group
dist.init_process_group("gloo", rank=rank, world_size=world_size)
# Explicitly setting seed to make sure that models created in two processes
# start from same random weights and biases.
torch.manual_seed(42)
def cleanup():
dist.destroy_process_group()
这里我们使用到了
torch.distributed.init_process_group(backend, init_method=None, timeout=datetime.timedelta(0, 1800), world_size=-1, rank=-1, store=None, group_name='')
这个 API 来初始化默认的分布式进程组,这还将初始化分布式程序包。
该函数有两种主要的调用方式:
明确指定 store,rank 和 world_size。 指定 init_method(URL 字符串),它指示在何处/如何发现对等方。(可选)指定 rank 和 world_size,或在 URL 中编码所有必需的参数并忽略它们。
现在,让我们创建一个 toy model,将其与 DDP 封装在一起,并提供一些虚拟输入数据。请注意,由于 DDP 将 0 级进程中的模型状态广播到 DDP 构造函数中的所有其他进程,因此无需担心不同的 DDP 进程从不同的模型参数初始值开始。
class ToyModel(nn.Module):
def __init__(self):
super(ToyModel, self).__init__()
self.net1 = nn.Linear(10, 10)
self.relu = nn.ReLU()
self.net2 = nn.Linear(10, 5)
def forward(self, x):
return self.net2(self.relu(self.net1(x)))
def demo_basic(rank, world_size):
setup(rank, world_size)
# Assume we have 8 GPU in total
# setup devices for this process, rank 1 uses GPUs [0, 1, 2, 3] and
# rank 2 uses GPUs [4, 5, 6, 7].
n = torch.cuda.device_count() // world_size
device_ids = list(range(rank * n, (rank + 1) * n))
# create model and move it to device_ids[0]
model = ToyModel().to(device_ids[0])
# output_device defaults to device_ids[0]
ddp_model = DDP(model, device_ids=device_ids)
loss_fn = nn.MSELoss()
optimizer = optim.SGD(ddp_model.parameters(), lr=0.001)
optimizer.zero_grad()
outputs = ddp_model(torch.randn(20, 10))
labels = torch.randn(20, 5).to(device_ids[0])
loss_fn(outputs, labels).backward()
optimizer.step()
cleanup()
def run_demo(demo_fn, world_size):
mp.spawn(demo_fn,
args=(world_size,),
nprocs=world_size,
join=True)
if __name__ == "__main__":
run_demo(demo_basic, 2)
例子 7 将 DDP 与模型并行性结合
DDP 还可以与多 GPU 模型一起使用,但是不支持进程内的复制。您需要为每个模型副本创建一个进程,与每个进程的多个模型副本相比,通常可以提高性能。当训练具有大量数据的大型模型时,DDP 包装多 GPU 模型特别有用。使用此功能时,需要小心地实现多 GPU 模型,以避免使用硬编码的设备,因为会将不同的模型副本放置到不同的设备上。
例如,下面这个模型显式的将不同的模块放置在不同的 GPU 上
class ToyMpModel(nn.Module):
def __init__(self, dev0, dev1):
super(ToyMpModel, self).__init__()
self.dev0 = dev0
self.dev1 = dev1
self.net1 = torch.nn.Linear(10, 10).to(dev0)
self.relu = torch.nn.ReLU()
self.net2 = torch.nn.Linear(10, 5).to(dev1)
def forward(self, x):
x = x.to(self.dev0)
x = self.relu(self.net1(x))
x = x.to(self.dev1)
return self.net2(x)
将多 GPU 模型传递给 DDP 时,不得设置 device_ids 和 output_device。输入和输出数据将通过应用程序或模型 forward() 方法放置在适当的设备中。
def demo_model_parallel(rank, world_size):
world_size)
# setup mp_model and devices for this process
dev0 = rank * 2
dev1 = rank * 2 + 1
mp_model = ToyMpModel(dev0, dev1)
ddp_mp_model = DDP(mp_model)
loss_fn = nn.MSELoss()
optimizer = optim.SGD(ddp_mp_model.parameters(), lr=0.001)
optimizer.zero_grad()
# outputs will be on dev1
outputs = ddp_mp_model(torch.randn(20, 10))
labels = torch.randn(20, 5).to(dev1)
labels).backward()
optimizer.step()
cleanup()
if __name__ == "__main__":
4)
例子 8 保存和加载检查点
使用 DDP 时,一种优化方法是仅在一个进程中保存模型,然后将其加载到所有进程中,从而减少写开销。
def demo_checkpoint(rank, world_size):
world_size)
# setup devices for this process, rank 1 uses GPUs [0, 1, 2, 3] and
# rank 2 uses GPUs [4, 5, 6, 7].
n = torch.cuda.device_count() // world_size
device_ids = list(range(rank * n, (rank + 1) * n))
model = ToyModel().to(device_ids[0])
# output_device defaults to device_ids[0]
ddp_model = DDP(model, device_ids=device_ids)
loss_fn = nn.MSELoss()
optimizer = optim.SGD(ddp_model.parameters(), lr=0.001)
CHECKPOINT_PATH = tempfile.gettempdir() + "/model.checkpoint"
if rank == 0:
# All processes should see same parameters as they all start from same
# random parameters and gradients are synchronized in backward passes.
# Therefore, saving it in one process is sufficient.
CHECKPOINT_PATH)
# Use a barrier() to make sure that process 1 loads the model after process
# 0 saves it.
dist.barrier()
# configure map_location properly
rank0_devices = [x - rank * len(device_ids) for x in device_ids]
device_pairs = zip(rank0_devices, device_ids)
map_location = {'cuda:%d' % x: 'cuda:%d' % y for x, y in device_pairs}
ddp_model.load_state_dict(
map_location=map_location))
optimizer.zero_grad()
outputs = ddp_model(torch.randn(20, 10))
labels = torch.randn(20, 5).to(device_ids[0])
loss_fn = nn.MSELoss()
labels).backward()
optimizer.step()
# Use a barrier() to make sure that all processes have finished reading the
# checkpoint
dist.barrier()
if rank == 0:
os.remove(CHECKPOINT_PATH)
cleanup()
4 总结
本文讲解了 torch.distributed 这一并行计算包的概念,实现细节和应用方式,并带大家快速入门 PyTorch 分布式训练。我们着重分析了 DataParallel 和 DistributedDataParallel 两种并行训练 API 的使用方法和原理异同
参考资料
https://pytorch.org/docs/stable/distributed.html
https://pytorch.apachecn.org/docs/1.7/59.html
如果觉得有用,就请分享到朋友圈吧!
公众号后台回复“长尾”获取长尾特征学习资源~

# CV技术社群邀请函 #
备注:姓名-学校/公司-研究方向-城市(如:小极-北大-目标检测-深圳)
即可申请加入极市目标检测/图像分割/工业检测/人脸/医学影像/3D/SLAM/自动驾驶/超分辨率/姿态估计/ReID/GAN/图像增强/OCR/视频理解等技术交流群
每月大咖直播分享、真实项目需求对接、求职内推、算法竞赛、干货资讯汇总、与 10000+来自港科大、北大、清华、中科院、CMU、腾讯、百度等名校名企视觉开发者互动交流~
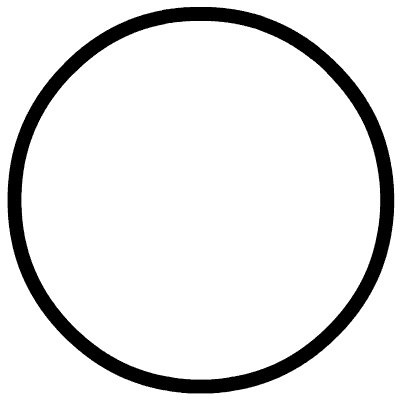