Pytorch nn.Transformer的mask理解
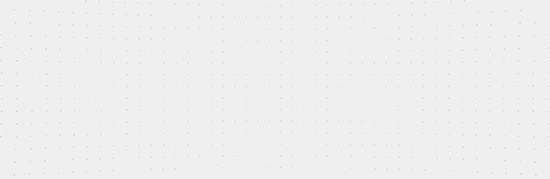
极市导读
本文对pytorch的mas的参数进行了一些补充解释以及说明,主要说明了mask_和key_padding_mask的作用。 >>本周六,极市CVPR2021线下沙龙即将举办,三位CVPR2021论文作者齐聚深圳!【报告三:戴志港-UP-DETR:针对目标检测的无监督预训练transformer】。点击蓝字即可免费报名,名额有限,先到先得!
pytorch也自己实现了transformer的模型,不同于huggingface或者其他地方,pytorch的mask参数要更难理解一些(即便是有文档的情况下),这里做一些补充和说明。(顺带提一句,这里的transformer是需要自己实现position embedding的,别乐呵乐呵的就直接去跑数据了)
16, num_encoder_layers=12) > transformer_model = nn.Transformer(nhead=
10, 32, 512)) > src = torch.rand((
20, 32, 512)) > tgt = torch.rand((
# 没有实现position embedding ,也需要自己实现mask机制。否则不是你想象的transformer > out = transformer_model(src, tgt)
首先看一下官网的参数
src – the sequence to the encoder (required). tgt – the sequence to the decoder (required). src_mask – the additive mask for the src sequence (optional). tgt_mask – the additive mask for the tgt sequence (optional). memory_mask – the additive mask for the encoder output (optional). src_key_padding_mask – the ByteTensor mask for src keys per batch (optional). tgt_key_padding_mask – the ByteTensor mask for tgt keys per batch (optional). memory_key_padding_mask – the ByteTensor mask for memory keys per batch (optional).
这里面最大的区别就是*mask_和*_key_padding_mask,_至于*是src还是tgt,memory,这不重要,模块出现在encoder,就是src,出现在decoder,就是tgt,decoder每个block的第二层和encoder做cross attention的时候,就是memory。
*mask 对应的API是attn_mask,*_key_padding_mask对应的API是key_padding_mask
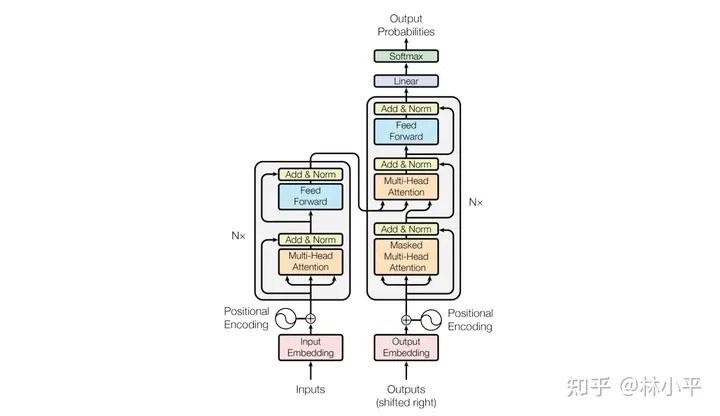
我们看看torch/nn/modules/activation.py当中MultiheadAttention模块对于这2个API的解释:
def forward(self, query, key, value, key_padding_mask=None,
need_weights=True, attn_mask=None):
# type: (Tensor, Tensor, Tensor, Optional[Tensor], bool, Optional[Tensor]) -> Tuple[Tensor, Optional[Tensor]]
r"""
Args:
query, key, value: map a query and a set of key-value pairs to an output.
See "Attention Is All You Need" for more details.
key_padding_mask: if provided, specified padding elements in the key will
be ignored by the attention. When given a binary mask and a value is True,
the corresponding value on the attention layer will be ignored. When given
a byte mask and a value is non-zero, the corresponding value on the attention
layer will be ignored
need_weights: output attn_output_weights.
attn_mask: 2D or 3D mask that prevents attention to certain positions. A 2D mask will be broadcasted for all
the batches while a 3D mask allows to specify a different mask for the entries of each batch.
Shape:
- Inputs:
- query: :math:`(L, N, E)` where L is the target sequence length, N is the batch size, E is
the embedding dimension.
- key: :math:`(S, N, E)`, where S is the source sequence length, N is the batch size, E is
the embedding dimension.
- value: :math:`(S, N, E)` where S is the source sequence length, N is the batch size, E is
the embedding dimension.
- key_padding_mask: :math:`(N, S)` where N is the batch size, S is the source sequence length.
If a ByteTensor is provided, the non-zero positions will be ignored while the position
with the zero positions will be unchanged. If a BoolTensor is provided, the positions with the
value of ``True`` will be ignored while the position with the value of ``False`` will be unchanged.
- attn_mask: 2D mask :math:`(L, S)` where L is the target sequence length, S is the source sequence length.
3D mask :math:`(N*num_heads, L, S)` where N is the batch size, L is the target sequence length,
S is the source sequence length. attn_mask ensure that position i is allowed to attend the unmasked
positions. If a ByteTensor is provided, the non-zero positions are not allowed to attend
while the zero positions will be unchanged. If a BoolTensor is provided, positions with ``True``
is not allowed to attend while ``False`` values will be unchanged. If a FloatTensor
is provided, it will be added to the attention weight.
- Outputs:
- attn_output: :math:`(L, N, E)` where L is the target sequence length, N is the batch size,
E is the embedding dimension.
- attn_output_weights: :math:`(N, L, S)` where N is the batch size,
L is the target sequence length, S is the source sequence length.
"""
key_padding_mask:用来遮蔽<PAD>以避免pad token的embedding输入。形状要求:(N,S) attn_mask:2维或者3维的矩阵。用来避免指定位置的embedding输入。2维矩阵形状要求:(L, S);也支持3维矩阵输入,形状要求:(N*num_heads, L, S)
其中,N是batch size的大小,L是目标序列的长度(the target sequence length),S是源序列的长度(the source sequence length)。这个模块会出现在上图的3个橙色区域,所以the target sequence 并不一定就是指decoder输入的序列,the source sequence 也不一定就是encoder输入的序列。
更准确的理解是,target sequence代表多头attention当中q(查询)的序列,source sequence代表k(键值)和v(值)的序列。例如,当decoder在做self-attention的时候,target sequence和source sequence都是它本身,所以此时L=S,都是decoder编码的序列长度。
key_padding_mask的作用
这里举一个简单的例子:
现在有一个batch,batch_size = 3,长度为4,token表现形式如下:
[
[‘a’,'b','c','<PAD>'],
[‘a’,'b','c','d'],
[‘a’,'b','<PAD>','<PAD>']
]
现在假设你要对其进行self-attention的计算(可以在encoder,也可以在decoder),那么以第三行数据为例,‘a’在做qkv计算的时候,会看到'b','<PAD>','<PAD>',但是我们不希望‘a’看到'<PAD>',因为他们本身毫无意义,所以,需要key_padding_mask遮住他们。
key_padding_mask的形状大小为(N,S),对应这个例子,key_padding_mask为以下形式,key_padding_mask.shape = (3,4):
[
[False, False, False, True],
[False, False, False, False],
[False, False, True, True]
]
值得说明的是,key_padding_mask本质上是遮住key这个位置的值(置0),但是<PAD> token本身,也是会做qkv的计算的,以第三行数据的第三个位置为例,它的q是<PAD>的embedding,k和v分别各是第一个的‘a’和第二个的‘b’,它也会输出一个embedding。
所以你的模型训练在transformer最后的output计算loss的时候,还需要指定ignoreindex=pad_index。以第三行数据为例,它的监督信号是[3205,1890,0,0],pad_index=0 。如此一来,即便位于<PAD>的transformer会疯狂的和有意义的position做qkv,也会输出embedding,但是我们不算它的loss,任凭它各种作妖。
attn_mask的作用
一开始看到有2个mask参数的时候,我也是一脸懵逼的,并且他们的shape居然要求还不一样。attn_mask到底用在什么地方呢?
decoder在做self-attention的时候,每一个位置不同于encoder,他是只能看到上文的信息的。key_padding_mask的shape为(batch_size, source_length),这意味着每个位置的query,他所看到的画面经过key_padding_mask后都是一样的(尽管他能做到batch的每一行数据mask的不一样),这不能满足如下模块的需求:
decoder的mask 多头注意力模块
这里需要的mask如下:
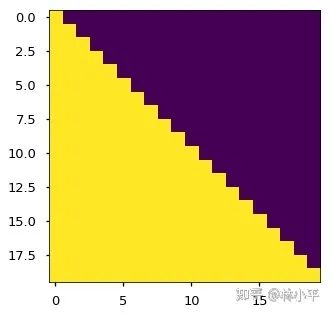
黄色是看得到的部分,紫色是看不到的部分,不同位置需要mask的部分是不一样的
而pytorch的nn.Transformer已经有了帮我们实现的函数:
def generate_square_subsequent_mask(self, sz: int) -> Tensor:
r"""Generate a square mask for the sequence. The masked positions are filled with float('-inf').
Unmasked positions are filled with float(0.0).
"""
mask = (torch.triu(torch.ones(sz, sz)) == 1).transpose(0, 1)
mask = mask.float().masked_fill(mask == 0, float('-inf')).masked_fill(mask == 1, float(0.0))
return mask
还是上面那个例子,以第一行数据['a','b','c','<PAD>'],为例(假设我们在用decoder做生成,研究block 的第一层layer 也就是self-attention),此时:
'a'可以看到'a' 'b'可以看到'a','b' 'c'可以看到'a','b','c' '<PAD>'理论上不应该看到什么,但是只要它头顶的监督信号是ignore_index,那就没有关系,所以让他看到'a','b','c','<PAD>'
回想一下attn_mask的形状要求,2维的时候是(L,S),3维的时候是(N*num_heads, L, S)。此时,由于qkv都是同一个序列(decoder底下的序列)所以L=S;又因为对于batch每一行数据来说,他们的mask机制都是一样的,即第i个位置的值,都只能看到上文的信息,所以我们的attn_mask用二维的就行,内部实现的时候会把mask矩阵广播到batch每一行数据中:
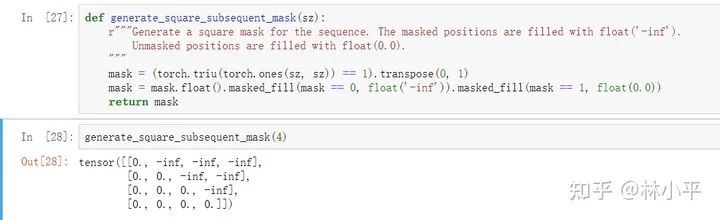
一般而言,除非你需要魔改transformer,例如让不同的头看不同的信息,否则二维的矩阵足够使用了。
什么时候用key_padding_mask,什么时候用attn_mask?
个人感觉最好是按照上面的约定的习惯来用,实际上,2个mask共同作用于同一个模型,非要用attn_mask代替key_padding_mask把<PAD>遮住,行不行?当然可以,只不过这只会增加你的工作量。
推荐阅读
2021-03-21
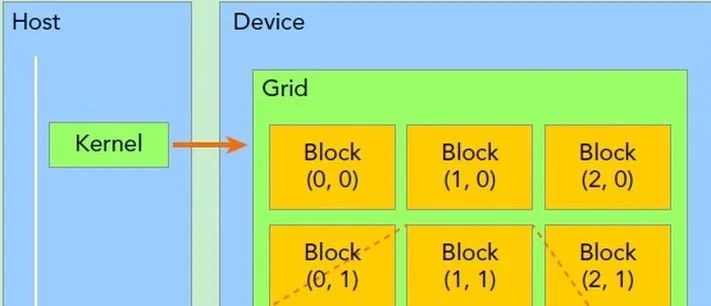
2020-11-06
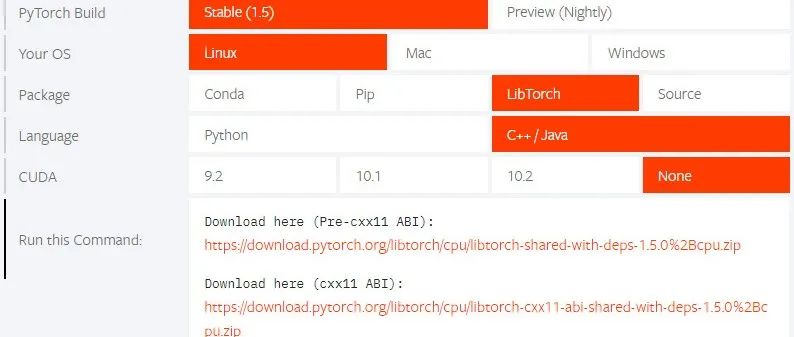
2020-08-24
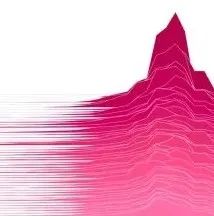

# CV技术社群邀请函 #
备注:姓名-学校/公司-研究方向-城市(如:小极-北大-目标检测-深圳)
即可申请加入极市目标检测/图像分割/工业检测/人脸/医学影像/3D/SLAM/自动驾驶/超分辨率/姿态估计/ReID/GAN/图像增强/OCR/视频理解等技术交流群
每月大咖直播分享、真实项目需求对接、求职内推、算法竞赛、干货资讯汇总、与 10000+来自港科大、北大、清华、中科院、CMU、腾讯、百度等名校名企视觉开发者互动交流~
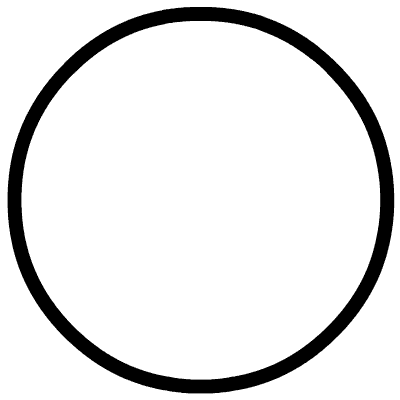