实践教程 | PyTorch分布式测试踩坑小结
共 22486字,需浏览 45分钟
·
2021-09-26 08:28
点击上方“程序员大白”,选择“星标”公众号
重磅干货,第一时间送达
来源 | https://zhuanlan.zhihu.com/p/276154597
编辑 | 极市平台
极市导读
本文主要介绍测试 Torch 相关模型遇到的分布式问题和解决其他框架遇到的奇奇怪怪的问题。 >>加入极市CV技术交流群,走在计算机视觉的最前沿
现有的训练框架一般都会牵涉到分布式、多线程和多进程等概念,所以较难 debug,而大家作为一些开源框架的使用者,有时未必会那么深入了解一些通信库的内部实现,无法第一时间找到问题,此事不宜求全责备。
因此,本文目的很简单,内容也很浅显,就是让大家可以根据报错信息和现象,得到最有可能的直接原因,从而修正之后能够快速将程序运行起来。
本文档是基于 DLPerf (https://github.com/Oneflow-Inc/DLPerf)测试框架总体测试过程的踩坑总结。测试范围主要涉及 ResNet50-v1.5 和 BERT 模型的 DeepLearningExamples (https://github.com/NVIDIA/DeepLearningExamples/tree/5cc03caa153faab7a2c3b1b5b5d63663f06ce1b4)仓库及各框架官方仓库的单机/多机实现,精度涵盖 FP32 及 AMP(Automatic Mixed Precision )。
What is DLPerf? 我有幸参与了一个 10.24 在南京的 DAI 大会,会上对 DLPerf 进行了英文~~安利~~分享,贴一下相关介绍。
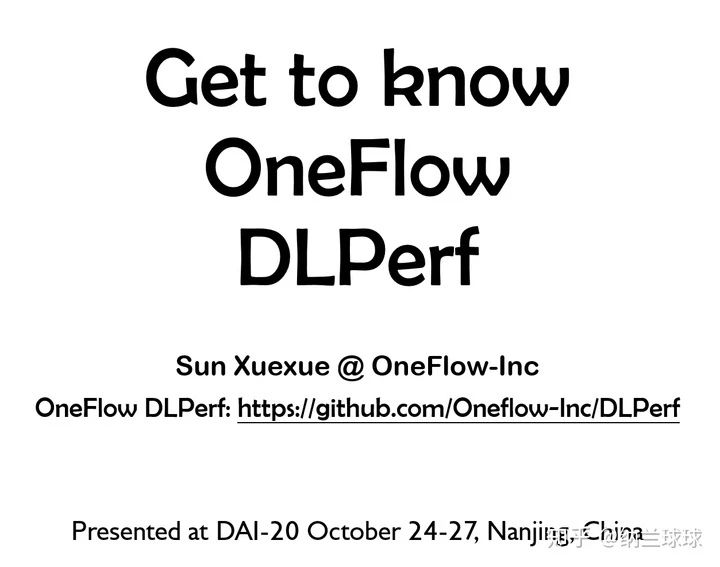
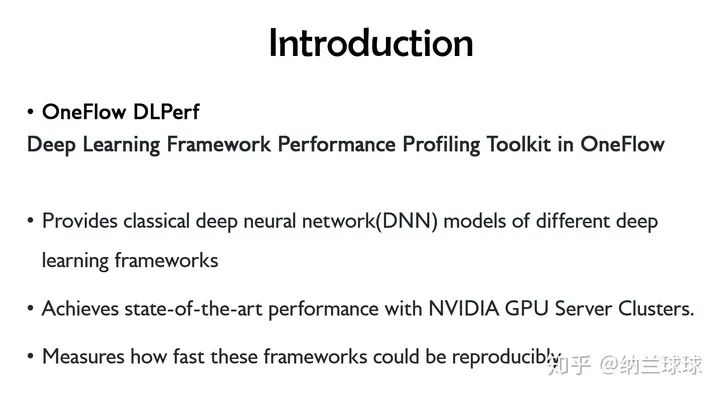
最开始只是为了解决这个小组里遇到的一些调试和流程规范的问题,然后就被拉进来了,这一拉就是两个多月......
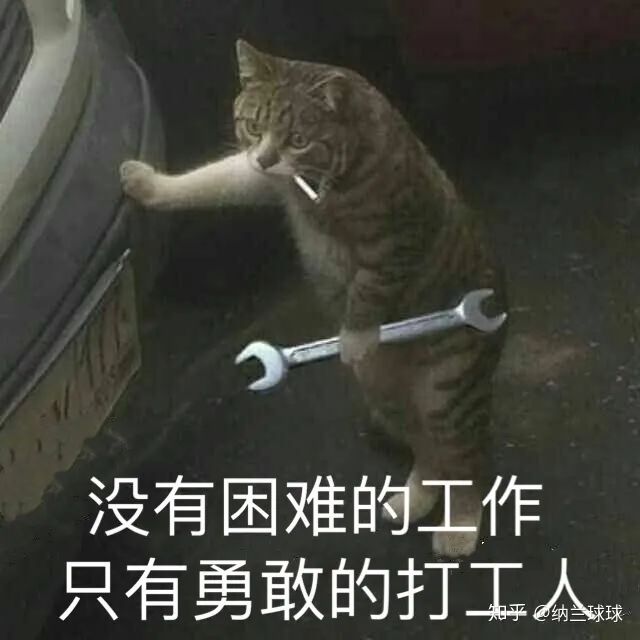
本文主要介绍测试 Torch 相关模型遇到的分布式问题和解决其他框架遇到的奇奇怪怪的问题。
环境 (Environment)
系统
硬件 GPU:Tesla V100-SXM2-16GB x 8 软件 驱动:NVIDIA 440.33.01 系统:Ubuntu 16.04 CUDA:10.2 cuDNN:7.6.5
NGC 容器
系统:Ubuntu 18.04 CUDA 10.2.89 cuDNN 7.6.5 NCCL:2.5.6 PyTorch:1.5.0a0+8f84ded OpenMPI 3.1.4 DALI 0.19.0 Python:3.6.9 更多容器细节请参考 NVIDIA Container Support Matrix。
Feature support matrix
Feature | ResNet50 v1.5 PyTorch |
---|---|
Multi-gpu training | Yes |
Multi-node training | Yes |
NVIDIA DALI | Yes |
Automatic mixed precision (AMP) | Yes |
相同环境结果复现,然后比 NVIDIA 增加了多机运行(Multi-node training)。
NVIDIA/DeepLearningExamples 踩坑
为了解决 torch 分布式遇到的问题,我去扒了 PyTorch 官方分布式相关内容,翻译详见
https://www.yuque.com/go/doc/14358835
实际上,在处理 NVIDIA 脚本的时候,主要难度在使用尽可能少的改动运行多机分布式上,因为大范围逻辑修改会引入潜在的性能变化,并增加用户复现的复杂度,代码版本管理也容易变得混乱。因此,我还是希望只在代码上稍作修改,这样既可以方便操作,同时尽可能地保留其原本的性能表现。当然,测试过程于我而言,是个学习过程,而学如逆水行舟,前期百般不得进,可能是因为我菜(划掉)退了。学习 DISTRIBUTED COMMUNICATION PACKAGE - TORCH.DISTRIBUTED · 语雀实际上,在处理 NVIDIA 脚本的时候,主要难度在使用尽可能少的改动运行多机分布式上,因为大范围逻辑修改会引入潜在的性能变化,并增加用户复现的复杂度,代码版本管理也容易变得混乱。因此,我还是希望只在代码上稍作修改,这样既可以方便操作,同时尽可能地保留其原本的性能表现。当然,测试过程于我而言,是个学习过程,而学如逆水行舟,前期百般不得进,可能是因为我菜【划掉】退了。
大致跑通难易度如下:
ResNet v1.5
ResNet50_v1.5 | 单机 | 多机 |
---|---|---|
容器 | 正常按文档跑就行 | NVIDIA 魔改的 PyTorch 多机跑通之难,难于上青天 |
物理机 | 安装依赖后即可运行 | 较容器里网络问题更易解决 |
BERT
BERT | 单机 | 多机 |
---|---|---|
容器 | 正常按文档跑就行 | 极易修改 |
物理环境运行
物理环境中也可运行该仓库,bert 需要提前准备数据集,而 rn50 原图输入即可。NVIDIA 大佬魔改了容器里的 pytorch,物理机上安装官方 pytorch 使用容器依赖测试,可以对比差异,更好地定位优化。
安装依赖
python3 -m pip install --user -i https://pypi.tuna.tsinghua.edu.cn/simple h5py tqdm boto3
pip3 install --no-cache -r requirement /DeepLearningExamples/PyTorch/LanguageModeling/BERT/requirements.txt
apex 包
此包异常傲娇,必须用单独小标题拉出来特别对待。
假如你用最普通的安装方式,python3 \-m pip install \--user \-i https://pypi.tuna.tsinghua.edu.cn/simple apex
或者 pip3 install apex
,那么在运行 bash scripts/run_pretraining.sh
时会报错:
Traceback (most recent call last):
File "/home/sunxue/DeepLearningExamples/PyTorch/LanguageModeling/BERT/run_pretraining.py", line 37, in <module>
from apex import amp
File "/home/sunxue/.local/lib/python3.6/site-packages/apex/__init__.py", line 18, in <module>
from apex.interfaces import (ApexImplementation,
File "/home/sunxue/.local/lib/python3.6/site-packages/apex/interfaces.py", line 10, in <module>
class ApexImplementation(object):
File "/home/sunxue/.local/lib/python3.6/site-packages/apex/interfaces.py", line 14, in ApexImplementation
implements(IApex)
File "/home/sunxue/.local/lib/python3.6/site-packages/zope/interface/declarations.py", line 706, in implements
raise TypeError(_ADVICE_ERROR % 'implementer')
TypeError: Class advice impossible in Python3. Use the @implementer class decorator instead.
这是 apex 使用这种方式安装版本不对,应该使用 https://www.github.com/nvidia/apex 仓库的安装,因此
pip uninstall apex
git clone https://www.github.com/nvidia/apex
cd apex-master
python3 setup.py install
重新运行 bash scripts/run_pretraining.sh
,报错
import amp_C
ModuleNotFoundError: No module named 'amp_C'
根据 https://github.com/NVIDIA/apex/issues/573 中介绍,issue 573 已更新,但需要使用如下命令安装
apex $ git pull
apex $ pip uninstall apex # repeat until you're sure it's gone
apex $ pip install -v --no-cache-dir --global-option="--cpp_ext" --global-option="--cuda_ext" ./
执行后,报错
Traceback (most recent call last):
File "<string>", line 1, in <module>
File "/tmp/pip-req-build-m4qm554e/setup.py", line 152, in <module>
check_cuda_torch_binary_vs_bare_metal(torch.utils.cpp_extension.CUDA_HOME)
File "/tmp/pip-req-build-m4qm554e/setup.py", line 106, in check_cuda_torch_binary_vs_bare_metal
"https://github.com/NVIDIA/apex/pull/323#discussion_r287021798. "
RuntimeError: Cuda extensions are being compiled with a version of Cuda that does not match the version used to compile Pytorch binaries. Pytorch binaries were compiled with Cuda 10.2.
调试放在了金山云上。这是由于金山云 2 号机上的 cuda-10.2 是由 rpm 安装的,并没有在/ usr/local/ 路径下留有 /cuda-10.2 等头文件或源文件,可安装 cuda-10.2 到 /home/user/ 路径。
按照 https://www.cnblogs.com/li-minghao/p/13089405.html 安装cuda10.2 到/home/user/
重新安装 apex,出现 warnning
/home/sunxue/.local/lib/python3.6/site-packages/torch/utils/cpp_extension.py:335: UserWarning: Attempted to use ninja as the BuildExtension backend but we could not find ninja.. Falling back to using the slow distutils backend.
warnings.warn(msg.format('we could not find ninja.'))
/home/sunxue/.local/lib/python3.6/site-packages/torch/utils/cpp_extension.py:277: UserWarning:
!! WARNING !!
!!!!!!!!!!!!!!!!!!!!!!!!!!!!!!!!!!!!!!!!!!!!!!!!!!!!!!!!!!!!!!!!!!!!!!!!!!!!!!!
Your compiler (g++ 4.8.5) may be ABI-incompatible with PyTorch!
Please use a compiler that is ABI-compatible with GCC 5.0 and above.
See https://gcc.gnu.org/onlinedocs/libstdc++/manual/abi.html.
See https://gist.github.com/goldsborough/d466f43e8ffc948ff92de7486c5216d6
for instructions on how to install GCC 5 or higher.
!!!!!!!!!!!!!!!!!!!!!!!!!!!!!!!!!!!!!!!!!!!!!!!!!!!!!!!!!!!!!!!!!!!!!!!!!!!!!!!
!! WARNING !!
warnings.warn(ABI_INCOMPATIBILITY_WARNING.format(compiler))
此时后续会出现错误
building 'apex_C' extension
creating build/temp.linux-x86_64-3.6
creating build/temp.linux-x86_64-3.6/csrc
gcc -pthread -Wno-unused-result -Wsign-compare -DNDEBUG -O2 -g -pipe -Wall -Wp,-D_FORTIFY_SOURCE=2 -fexceptions -fstack-protector-strong --param=ssp-buffer-size=4 -grecord-gcc-switches -m64 -mtune=generic -D_GNU_SOURCE -fPIC -fwrapv -fPIC -I/home/sunxue/.local/lib/python3.6/site-packages/torch/include -I/home/sunxue/.local/lib/python3.6/site-packages/torch/include/torch/csrc/api/include -I/home/sunxue/.local/lib/python3.6/site-packages/torch/include/TH -I/home/sunxue/.local/lib/python3.6/site-packages/torch/include/THC -I/usr/include/python3.6m -c csrc/flatten_unflatten.cpp -o build/temp.linux-x86_64-3.6/csrc/flatten_unflatten.o -DTORCH_API_INCLUDE_EXTENSION_H -DTORCH_EXTENSION_NAME=apex_C -D_GLIBCXX_USE_CXX11_ABI=0 -std=c++14
gcc: error: unrecognized command line option ‘-std=c++14’
error: command 'gcc' failed with exit status 1
这是因为它要求使用 gcc 5.x,但是机器上是 gcc 4.8.5。
原本希望在不升级的情况下凑合用用,但是将-std=c++14
替换为 -std=c++1y
后,仍会编译失败,应该是还有其他问题。
所以,不要心存侥幸心理了,还是需要换 gcc 5.x,此处选用 gcc 5.5(实测 5.4 亦可),本地安装 gcc 5.5 后,重新安装 apex,
apex $ git pull
apex $ pip uninstall apex # repeat until you're sure it's gone
apex $ pip install -v --no-cache-dir --global-option="--cpp_ext" --global-option="--cuda_ext" ./
即可安装成功。
修改 run_pretraining.sh 脚本的 --json-summary
路径,./result/dllogger.json
---> ./dllogger.json
,即可运行成功,并成功打印 log:
Iteration: 3%|▎ | 127/4420 [00:16<08:53, 8.05it/s]DLL 2020-08-10 21:03:36.178776 - PARAMETER loss_scale : 524288.0
DLL 2020-08-10 21:03:36.182556 - Training Epoch: 0 Training Iteration: 0 average_loss : 11.1544189453125 step_loss : 10.8203125 learning_rate : 2.9986455118223097e-06
Iteration: 6%|▌ | 255/4420 [00:35<09:11, 7.55it/s]DLL 2020-08-10 21:03:55.047705 - PARAMETER loss_scale : 262144.0
DLL 2020-08-10 21:03:55.051043 - Training Epoch: 0 Training Iteration: 0 average_loss : 11.14263916015625 step_loss : 10.75 learning_rate : 2.9986455118223097e-06
Iteration: 9%|▊ | 383/4420 [00:54<09:43, 6.92it/s]DLL 2020-08-10 21:04:13.038226 - PARAMETER loss_scale : 131072.0
DLL 2020-08-10 21:04:13.041031 - Training Epoch: 0 Training Iteration: 0 average_loss : 11.17144775390625 step_loss : 10.7421875 learning_rate : 2.9986455118223097e-06
Iteration: 12%|█▏ | 511/4420 [01:13<09:24, 6.93it/s]DLL 2020-08-10 21:04:32.085152 - PARAMETER loss_scale : 65536.0
DLL 2020-08-10 21:04:32.087935 - Training Epoch: 0 Training Iteration: 0 average_loss : 11.1295166015625 step_loss : 11.4375 learning_rate : 2.9986455118223097e-06
物理机单机 bert rn50 done。rn50 在物理机上先安装依赖
python3 -m pip install --no-cache -r requirement
apex 安装解决后其他问题不大,直接按脚本跑就可以,多机运行是在容器踩坑之后,该填的都填上了,所以也较为顺利。
容器环境运行
调试的时候用的是 20.06 容器,参考 BERT For PyTorch 的 Quick Start Guide 操作,容器大小其实还可以,就是国网内一旦要下外面的东西就很 emmm,因此下载构建就需要很长时间,这是正常的,最好能有梯子翻墙。
bash scripts/docker/build.sh
报错:
After this operation, 33.8 kB of additional disk space will be used.
Get:1 http://archive.ubuntu.com/ubuntu bionic/universe amd64 libb64-0d amd64 1.2-4 [9276 B]
debconf: unable to initialize frontend: Dialog
debconf: (TERM is not set, so the dialog frontend is not usable.)
debconf: falling back to frontend: Readline
debconf: unable to initialize frontend: Readline
debconf: (This frontend requires a controlling tty.)
debconf: falling back to frontend: Teletype
dpkg-preconfigure: unable to re-open stdin:
忽略,继续 bash scripts/docker/launch.sh
,报错
ERROR: This container was built for NVIDIA Driver Release 450.36 or later, but
version 440.64 was detected and compatibility mode is UNAVAILABLE.
需要升级驱动至 450.36 及以上。驱动升级后,容器启动正常。NGC 20.03 使用原驱动可正常运行。
多机血泪史
自古以来,分布式就是计算机架构理论与基础不可分割的一部分,同时在使用效果的性价比上也争议颇多。但这不是重点,重点是光是使用起来就很复杂。一般的机器学习框架,pytorch、mxnet、tensoflow 等都是使用第三方工具实现分布式计算,这其实就需要你具有 openmpi、horovod、nccl 的预备知识,当它们和 docker 结合可能就更~~~~
docker 容器连通问题
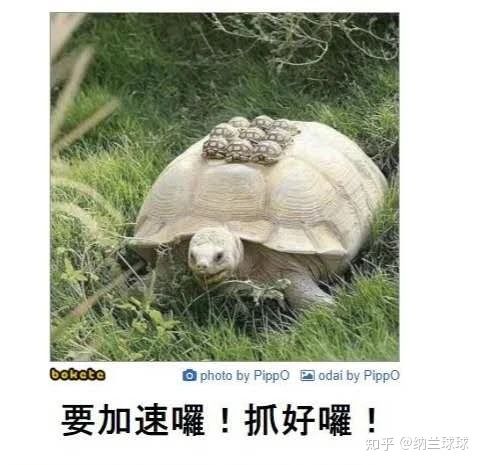
如果是在 docker 容器中进行多机训练,需要保证 docker 容器之间互相可以通过指定端口 ssh 免密登录。即:在10.11.0.2 节点的 docker 容器内通过 ssh root@10.11.0.3 \-p 10001
可以直接登录 10.11.0.3 节点的 docker 容器,无需输入密码。对于 docker 容器,用户可以使用 docker run \--network none
完全禁用网络,禁用所有网络传入和传出, 仅通过文件或 STDIN 和 STDOUT 执行 I/O。而多机分布式运行当然需要相互之间可以通信的容器,官方有 4 种实现方式:
**bridge 模式:**在默认 桥接器上创建一个网络堆栈 **host 模式:**在容器内使用主机的网络堆栈。 **container: <name|id> 模式:**重用另一个容器的网络堆栈,过其名称或 id 指定。 **<network-name>|<network-id> 模式:**连接到用户定义的网络
此处网络堆栈指的是容器用来存储网络相关参数配置的堆栈。可以使用 docke inspect 容器id
来查看具体信息。
1.docker 的 bridge 模式
即 docker 的默认模式。该模式下,容器内部和物理机的端口是隔离的。当 docker 进程启动时,会在主机上创建一个名为 docker0 的虚拟网桥,此主机上启动的 docker 容器会连接到这个虚拟网桥上。虚拟网桥的工作方式和物理交换机类似,这样主机上的所有容器就通过交换机连在了一个二层网络中。
从 docker0 子网中分配一个 IP 给容器使用,并设置 docker0 的 IP 地址为容器的默认网关。在主机上创建一对虚拟网卡 veth pair 设备,docker 将 veth pair 设备的一端放在新创建的容器中,并命名为 eth0(容器的网卡),另一端放在主机中,以 vethxxx 这样类似的名字命名,并将这个网络设备加入到 docker0 网桥中。可以通过 brctl show
命令查看。
不写 --net
(现在新版应该是 --network
)参数,就是 docker 的默认网络模式,bridge 模式。使用 docker run \-p
时,docker 实际是在 iptables 做了 DNAT 规则,实现端口转发功能。可以使用 iptables \-t nat \-vnL
查看。通过 docker run
增加参数如:-p 9000:9000
进行端口映射,表明物理机 9000 端口映射到容器内 9000 端口,docker 容器多机时即可指定 9000 端口进行通信。
2. docker 的 host 模式
如果启动容器的时候使用 host 模式,那么这个容器将不会获得一个独立的 Network Namespace,而是和宿主机共用一个 Network Namespace。容器将不会虚拟出自己的网卡,配置自己的IP等,而是使用宿主机的 IP 和端口。但是,容器的其他方面,如文件系统、进程列表等还是和宿主机隔离的。host 模式,需要通过 docker run
时添加参数 --network=host
指定,该模式下表示容器使用主机的网络堆栈,和物理机共用端口(没有隔离),需要修改容器内 ssh 服务的通信端口号(vim /etc/ssh/sshd_config
,需安装 openssh-server),用于 docker 容器多机通讯。
3. docker 的 container:<name|id> 模式
这个模式指定新创建的容器和已经存在的一个容器共享一个 Network Namespace,而不是和宿主机共享。新创建的容器不会创建自己的网卡,配置自己的 IP,而是和一个指定的容器共享 IP、端口范围等。同样,两个容器除了网络方面,其他的如文件系统、进程列表等还是隔离的。两个容器的进程可以通过 lo 网卡设备通信。Docker 默认的网络环境下,单台主机上的 Docker 容器可以通过 docker0 网桥直接通信,而不同主机上的 Docker 容器之间只能通过在主机上做端口映射进行通信。再加上考虑到各种测试环境的网络复杂性,本次并未采用该方式进行容器互联。
4. docker的 <network-name>|<network-id> 模式
用户可以使用 docker 网络驱动程序或外部网络驱动程序插件创建网络, 建立并使用自己的 bridge 网络或 overlay 网络。但考虑到各种测试环境的网络复杂性,本次并未采用该方式进行容器互联。
最后选用的是暴露集群上容器之间的端口进行 ssh 通信,要使本机开放 ssh 服务,host/bridge 两种方式都可以,只需要安装 openssh-server,设置完相应参数后重启 ssh 服务即可,详情见 NVIDIA/DeepLearningExamples PyTorch BERT 测评。
IB 驱动
参考 How-to: Deploy RDMA accelerated Docker container over InfiniBand fabric 一文,即使物理机安装了 IB 驱动,仍然需要在容器内安装 Mellanox 软硬件组件,因此容器内调用 nccl 走 IB 需要重新安装 IB 驱动,否则只会使用 socket 通信。对于 torch,tf 等主力使用 nccl 或 horovod(也是优先使用 nccl)的框架速度影响比较大,对于使用 openmpi 的mxnet,百兆 socket 和 IB 之间区别没那么显著。对于模型,bert 的增益远比 rn50 明显。
rn50
NVIDIA 大佬们并没有直接使用 torch.multiprocessing ,而是自己写了 multiproc.py 去根据配置的 nnode, node_rank, nproc_per_node, master_addr, master_port
起线程,以实现单机多卡运行。在此基础上修改为多机运行,根据
一文中 torch 的官方叙述,在使用了 GPU 的架构场景下,测试性价比最低的是使用 openmpi(因为需要源码编译 torch 和 openmpi,这当中显而易见地会遇到很多依赖问题),gloo 也并不鼓励,最为推荐的是 nccl,速度快,同时对源码修改最少,是性能测试首当其冲的选择。问题来了,直接传参给 multiproc.py,以 2 机 16 卡为例, 执行
python ./multiproc.py --nnodes 2 --nproc_per_node 8 --master ./main.py --arch resnet50 -c fanin --label-smoothing 0.1 <path to imagenet>
报错
RuntimeError: CUDA error: out of memory
显然这个代码就是只为单机训练定制的,我设想的单机 8 卡运行无法通过修改这个参数直接实现,8 张卡的 gpu 内存会集中分配到 0 号卡上,顿时 oom。由于项目初期的规划不清晰,当初在这里还遭遇了是否要统一使用 mpi 实现多机运行或者是否直接使用 torch.distributed.launch 而不复用 multiproc.py 等等问题,这样一来,实验成本就大大增加了。因此,悲催地遇到了调试 mpi 时线程不完全结束、多种协议使用或者脚本和环境变量交叉使用带来的端口冲突,或者显存占用没释放等每个小白都会遇到的分布式使用问题。horovod 也是一样,同时会因为引入的新框架造成工作量笛卡尔积式增加。
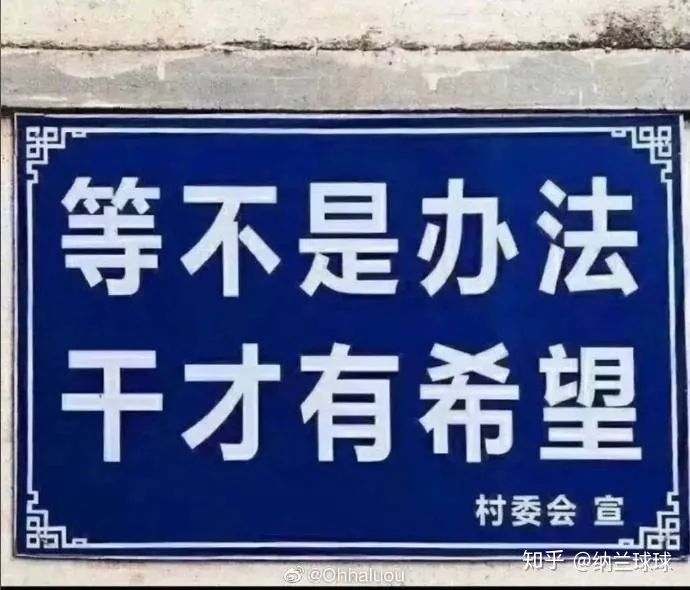
两害相权取其轻,比下来还是用原脚本解决内存问题会比较好。现在看来,解决的思路很清晰,但这个结论来得并不轻松,正所谓山穷水复疑无路,原地返回是正途。
假如改使用 torch.distributed.launch,运行 main.py 又会报错
RuntimeError: Address already in use
......
相反,看下 bert 的 NVIDIA 脚本,因为直接使用了 python \-m
,将库模块作为脚本运行,在不增删 py 文件情况下使用 torch.distributed.launch
,非常方便修改进程分布式参数,只需要注意多机 docker 之间的 ssh 互联即可。
因此对于 torch, 最方便快捷的分布式实现是使用 python3 \-m torch.distributed.launch
,不过这只是解决了多机分布式问题,还要注意计算逻辑,正确使用 allreduce 和 allgather 等集合通信计算~~否则即使是行为艺术,也不推荐跑几个礼拜只为得到我正在训练的幻觉和一个错误结果。
同时因为 torch 调用 nccl 需要手动在每台机器上启动进程,需要自己写 ssh 远程执行脚本来完成自动化。
混合精度
相对与多机运行带来的麻烦,混合精度只需要注意选项的效果,so sweeeeeeeeet! 当你使用 --fp16
选项运行,非常贴心地提示
Warning: FP16_Optimizer is deprecated and dangerous, and will be deleted soon.
If it still works, you're probably getting lucky.
For mixed precision, use the documented API https://nvidia.github.io/apex/amp.html,
with opt_level=O1.
因此,最好别用这个选项,而是通过修改 apex.amp 的 opt_level
值来进行复合精度的测试。
opt_level | Precision |
---|---|
O0 | FP32 training |
O1 | Mixed Precision (recommended for typical use) |
O2 | “Almost FP16” Mixed Precision |
O3 | FP16 training |
可以根据自己的测试需求修改源码。
PyTorch Official examples/imagenet/ 踩坑
官方仓库只有 resnet50_v1.5,没有 bert 网络,因此物理机上只测试了 resnet50_v1.5 网络的单机多机结果。
resnet50_v1.5 | 单机 | 多机 |
---|---|---|
容器 | 正常按文档跑就行 | 稍作修改 |
物理机 | 正常按文档跑就行 | 稍作修改 |
并添加 DALI 数据处理方式。后续还会增加 AMP 哦~
安装 NVIDIA DALI
使用 NGC 容器中使用的 dali 0.19.0,参考官网文档 。
由于这版本比较旧,所以选择的是 nvidia dali,直接根据 python 版本去镜像下载 whl 包
curl nvidia_dali-0.19.0-1119077-cp37-cp37m-manylinux1_x86_64.whl
python -m pip install nvidia_dali-0.19.0-1119077-cp37-cp37m-manylinux1_x86_64.whl
即可。
对于 dali 包,目前最快速,成功率最高的方式就是确认好需要安装的版本后,直接下载对应版本的 whl 包然后 pip 安装,如果直接根据 daili 官网的文档,使用
pip install --extra-index-url https://developer.download.nvidia.com/compute/redist --upgrade nvidia-dali-cuda110
等命令安装,实际使用时可能会遇到报错
Traceback (most recent call last):
File "/export/nfs/sunxue/DeepLearningExamples/PyTorch/Classification/ConvNets/mnasnet/training/FP32/../../../launch.py", line 7, in <module>
from main import main, add_parser_arguments, available_models
File "/export/nfs/sunxue/DeepLearningExamples/PyTorch/Classification/ConvNets/main.py", line 49, in <module>
from image_classification.dataloaders import *
File "/export/nfs/sunxue/DeepLearningExamples/PyTorch/Classification/ConvNets/image_classification/dataloaders.py", line 79, in <module>
class HybridTrainPipe(Pipeline):
NameError: name 'Pipeline' is not defined
但这并不是 python 包 import 不当造成的问题,设置环境变量并不能解决,还是请安装合适版本。
Q&A
报错
packages/nvidia/dali/pipeline.py", line 410, in share_outputs
return self._pipe.ShareOutputs()
RuntimeError: Critical error in pipeline: CUDA allocation failed
Current pipeline object is no longer valid.
这通常是因为 CUDA 内存不够,可能是 GPU 没全可见,可以试着
export CUDA_VISIBLE_DEVICES=0,1,2,3,4,5,6,7报错
报错
RuntimeError: cuDNN error: CUDNN_STATUS_INTERNAL_ERROR
这通常可能是因为 GPU 显存不够,或者与其他 GPU 正在运行的程序撞车了。
报错
RuntimeError: CUDA tensor detected and the MPI used doesn't have CUDA-aware MPI support
假如你和你最后的倔强坚持想要使用 openmpi,并且遇此报错,请不要抱侥幸心理,源码编译安装支持 GPU 的 openmpi 和 pytorch 吧,详情可参考 Segfault using cuda with openmpi 和 Distributed Communication with openmpi fails。
当初因为时间限制和性能考虑,测试时并没有过多考虑 openMPI 的使用,但是现在了解到 openMPI 的多线程特性,后续使用过也会给出这方面的踩坑小结。
报错
There are not enough slots available in the system to satisfy the 16
slots that were requested by the application:
10.11.0.4:8
Either request fewer slots for your application, or make more slots
available for use.
A "slot" is the Open MPI term for an allocatable unit where we can
launch a process. The number of slots available are defined by the
environment in which Open MPI processes are run:
1. Hostfile, via "slots=N" clauses (N defaults to number of
processor cores if not provided)
2. The --host command line parameter, via a ":N" suffix on the
hostname (N defaults to 1 if not provided)
3. Resource manager (e.g., SLURM, PBS/Torque, LSF, etc.)
4. If none of a hostfile, the --host command line parameter, or an
RM is present, Open MPI defaults to the number of processor cores
In all the above cases, if you want Open MPI to default to the number
of hardware threads instead of the number of processor cores, use the
--use-hwthread-cpus option.
Alternatively, you can use the --oversubscribe option to ignore the
number of available slots when deciding the number of processes to
launch.
这通常是因为两个 host 之间用了空格连接(×),换成用逗号(√),再次运行即可。
报错
RuntimeError: Expected tensor for argument #1 'input' to have the same device as tensor for argument #2 'weight'; but device 0 does not equal 1 (while checking arguments for cudnn_convolution)
这通常是脚本只在一台机器上更改了计算,导致输出数据与其他机器上预留的输出内存大小不符,查找到改动的地方,要么在所有机器上的脚本里修改,要么别改。
使用 nccl 时 GPU 显存有约 220M 左右的分配,但迟迟无动静
ssh 连接有问题,没有通讯成功。
报错
RuntimeError: CUDA out of memory. Tried to allocate 74.00 MiB (GPU 0; 15.78 GiB total capacity; 0 bytes already allocated; 55.44 MiB free; 0 bytes reserved in total by PyTorch)
多机多卡时所有内存都被分配到了第一张卡上。需要梳理逻辑,找到显存分配的地方进行修改。同时,自己写脚本时,应避免使用
运行 NVIDIA BERT 报错
Traceback (most recent call last):
File "/home/leinao/DeepLearningExamples/PyTorch/LanguageModeling/BERT/run_pretraining.py", line 678, in <module>
args, final_loss, train_time_raw, global_step = main()
File "/home/leinao/DeepLearningExamples/PyTorch/LanguageModeling/BERT/run_pretraining.py", line 506, in main
model, optimizer, lr_scheduler, checkpoint, global_step, criterion = prepare_model_and_optimizer(args, device)
File "/home/leinao/DeepLearningExamples/PyTorch/LanguageModeling/BERT/run_pretraining.py", line 359, in prepare_model_and_optimizer
args.resume_step = max([int(x.split('.pt')[0].split('_')[1].strip()) for x in model_names])
ValueError: max() arg is an empty sequence
这通常是数据集路径错误,导致无数据读入,更换正确的数据集路径。
运行 NVIDIA BERT 报错
Traceback (most recent call last):
File "/home/leinao/DeepLearningExamples/PyTorch/LanguageModeling/BERT/run_pretraining.py", line 678, in <module>
args, final_loss, train_time_raw, global_step = main()
File "/home/leinao/DeepLearningExamples/PyTorch/LanguageModeling/BERT/run_pretraining.py", line 502, in main
device, args = setup_training(args)
File "/home/leinao/DeepLearningExamples/PyTorch/LanguageModeling/BERT/run_pretraining.py", line 303, in setup_training
torch.distributed.init_process_group(backend='nccl', init_method='env://')
File "/home/leinao/anaconda3/lib/python3.7/site-packages/torch/distributed/distributed_c10d.py", line 422, in init_process_group
store, rank, world_size = next(rendezvous_iterator)
File "/home/leinao/anaconda3/lib/python3.7/site-packages/torch/distributed/rendezvous.py", line 172, in _env_rendezvous_handler
store = TCPStore(master_addr, master_port, world_size, start_daemon, timeout)
ValueError: host not found: Name or service not known
端口错误,更换空闲的非特殊端口即可。
使用 multiproc.py 报错
Traceback (most recent call last):
File "main.py", line 540, in <module>
main(args)
File "main.py", line 311, in main
dist.init_process_group(backend="nccl", init_method="env://")
File "/opt/conda/lib/python3.6/site-packages/torch/distributed/distributed_c10d.py", line 393, in init_process_group
store, rank, world_size = next(rendezvous_iterator)
File "/opt/conda/lib/python3.6/site-packages/torch/distributed/rendezvous.py", line 172, in _env_rendezvous_handler
store = TCPStore(master_addr, master_port, world_size, start_daemon, timeout)
RuntimeError: Address already in use
改变端口仍然报错,此时需检查环境变量,一旦设置了 torch 分布式的环境变量,即使都是 nccl 通信,也会地址冲突。
报错
RuntimeError: NCCL error in: ../torch/lib/c10d/ProcessGroupNCCL.cpp:514, unhandled system error, NCCL version 2.6.3
或者死锁,表现为长时间 log 不打印等,通常是集群内个节点上的文件修改不同步,因此脚本不一致导致错误。Modify scripts together or never!
报错
[[INVALID],INVALID] ORTE_ERROR_LOG: Not found in file ess_env_module.c at line 367
[[INVALID],INVALID]-[[59225,0],0] mca_oob_tcp_peer_try_connect: connect to 255.255.255.255:51754 failed: Network is unreachable (101)
--------------------------------------------------------------------------
ORTE was unable to reliably start one or more daemons.
This usually is caused by:
* not finding the required libraries and/or binaries on
one or more nodes. Please check your PATH and LD_LIBRARY_PATH
settings, or configure OMPI with --enable-orterun-prefix-by-default
* lack of authority to execute on one or more specified nodes.
Please verify your allocation and authorities.
* the inability to write startup files into /tmp (--tmpdir/orte_tmpdir_base).
Please check with your sys admin to determine the correct location to use.
* compilation of the orted with dynamic libraries when static are required
(e.g., on Cray). Please check your configure cmd line and consider using
one of the contrib/platform definitions for your system type.
* an inability to create a connection back to mpirun due to a
lack of common network interfaces and/or no route found between
them. Please check network connectivity (including firewalls
and network routing requirements).
在确保了每台机器安装的 openmpi 版本相同时,这多半是 ssh 免密授权没配置好,如 ssh 公钥更新,但 authorized_keys 文件没更新。
By the way
安装 Paddle-DALI-plugin
因为 Paddle 没有对外放出官网所说的 支持了 DALI 优化的容器,所以我就自己装了一下,让它在物理机上跑下试试可以提升多少,然而......
https://www.yuque.com/go/doc/14336512
当然,该仓库将会持续更新,后面很快会有 InsightFace,mask rcnn 等等~
语雀地址:
PyTorch 分布式测试踩坑小结:https://www.yuque.com/docs/share/72990b7e-87ee-4113-8b74-a86a1c881aba%3F%23
更多详情可移步:
NVIDIA/DeepLearningExamples PyTorch ResNet50 v1.5 测评:https://github.com/Oneflow-Inc/DLPerf/tree/master/PyTorch/resnet50v1.5
PyTorch ResNet50 v1.5 测评:https://github.com/Oneflow-Inc/DLPerf/tree/master/PyTorch/resnet50v1.5
参考:
https://github.com/pytorch/pytorch/issues/20200
https://www.cnblogs.com/yy-cxd/p/6553624.html
推荐阅读
关于程序员大白
程序员大白是一群哈工大,东北大学,西湖大学和上海交通大学的硕士博士运营维护的号,大家乐于分享高质量文章,喜欢总结知识,欢迎关注[程序员大白],大家一起学习进步!