Neo4j入门(二)批量插入节点、关系
共 9801字,需浏览 20分钟
·
2021-07-16 10:08
本文将介绍如何在Neo4j中批量插入节点、关系,提升图谱构建的效率。
在讲解批量插入节点、关系前,我们需要了解下节点重复创建问题。
节点重复创建
在Neo4j中,我们如果对同一个节点进行重复插入,则图谱中会存在多个重复节点,这是因为Neo4j本身自带一个自增id造成的。
我们来创建name为Google、address为U.S.的节点。使用CQL语句如下:
create (company: Company{name: "Google", address: "U.S."});
create (company: Company{name: "Google", address: "U.S."});
可以看到,图谱中存在两个貌似一模一样的节点,如下图:
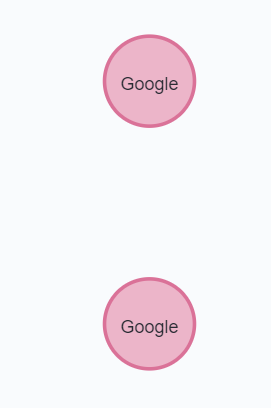
实际上,这两个节点只有id不同,这个id是Neo4j自带的id,由系统生成。
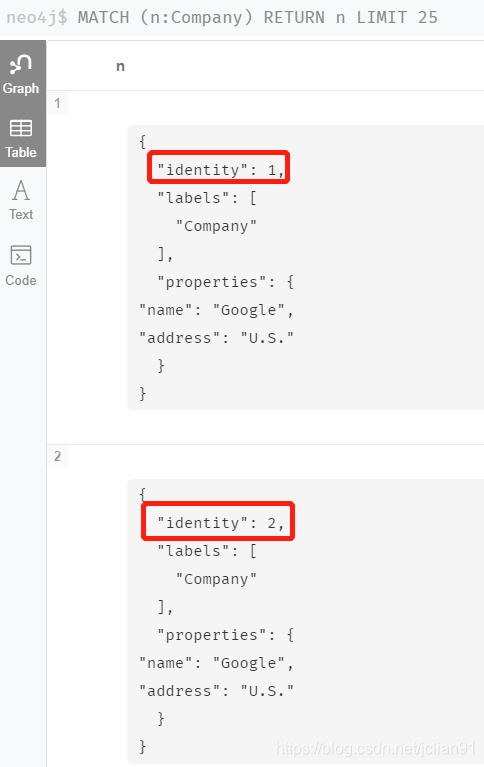
避免重复创建节点的办法如下:
使用MERGE命令代替
在创建节点前,先查询下图谱中该节点是否存在
数据集
我们选用OpenKG中的行政区相邻数据集(数据有点儿小问题,需要自己改动下),访问网址为:http://www.openkg.cn/dataset/xzqh,我们想要构建的示例图谱(局部)如下:
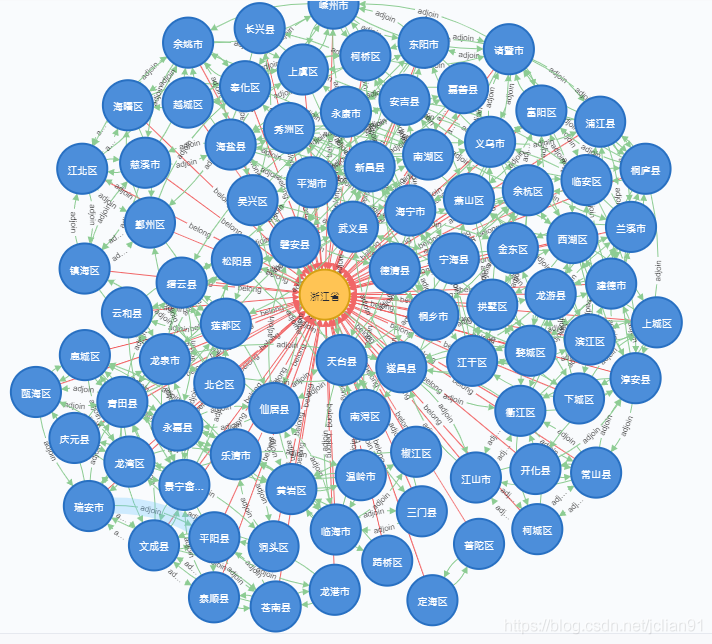
该图谱中一共有个2834个节点(其中城市节点2801个,省份节点33个),18807条关系。
单个节点、关系创建
首先我们采用单个节点、关系依次创建,观察其图谱构建的耗时。示例Python代码如下:
# -*- coding: utf-8 -*-
import json
import time
from py2neo import Graph, Node, Relationship
from py2neo import NodeMatcher, RelationshipMatcher
# 连接Neo4j
url = "http://localhost:7474"
username = "neo4j"
password = "password"
graph = Graph(url, auth=(username, password))
print("neo4j info: {}".format(str(graph)))
# 读取数据
with open("data.json", "r", encoding="utf-8") as f:
data_dict = json.loads(f.read())
nodes = data_dict["nodes"]
relations = data_dict["relations"]
# 创建节点
s_time = time.time()
create_node_cnt = 0
node_matcer = NodeMatcher(graph)
for node in nodes:
label = node["label"]
name = node["name"]
find_node = node_matcer.match(label, name=name).first()
if find_node is None:
attrs = {k: v for k, v in node.items() if k != "label"}
node = Node(label, **attrs)
graph.create(node)
create_node_cnt += 1
print(f"create {create_node_cnt} nodes.")
# 创建关系
create_rel_cnt = 0
relation_matcher = RelationshipMatcher(graph)
for relation in relations:
s_node, s_label = relation["subject"], relation["subject_type"]
e_node, e_label = relation["object"], relation["object_type"]
rel = relation["predicate"]
start_node = node_matcer.match(s_label, name=s_node).first()
end_node = node_matcer.match(e_label, name=e_node).first()
if start_node is not None and end_node is not None:
r_type = relation_matcher.match([start_node, end_node], r_type=rel).first()
if r_type is None:
graph.create(Relationship(start_node, rel, end_node))
create_rel_cnt += 1
print(f"create {create_rel_cnt} relations.")
# 输出信息
e_time = time.time()
print(f"create {create_node_cnt} nodes, create {create_rel_cnt} relations.")
print(f"cost time: {round((e_time-s_time)*1000, 4)}ms")
上述创建图谱脚本共耗时802.1秒。
无疑上述操作过程是非常耗时的,在创建节点时,需要先查询每个节点在图谱中是否存在,不存在则创建该节点;在创建关系时,需要先查询两个节点是否存在,如节点存在,而关系不存在,则创建该关系。在整个操作过程中,需要频繁地查询图谱、创建节点、创建关系,这无疑是该脚本耗时的地方所在。
批量节点、关系创建
通过创建子图(Subgraph),我们可以实现批量创建节点、关系,这样可以提升图谱构建的效率。批量节点、关系创建的Python代码如下:
# -*- coding: utf-8 -*-
import json
import time
from py2neo import Graph, Node, Relationship, Subgraph
from py2neo import RelationshipMatcher
# 连接Neo4j
url = "http://localhost:7474"
username = "neo4j"
password = "password"
graph = Graph(url, auth=(username, password))
print("neo4j info: {}".format(str(graph)))
# 读取数据
with open("data.json", "r", encoding="utf-8") as f:
data_dict = json.loads(f.read())
nodes = data_dict["nodes"]
relations = data_dict["relations"]
# 查询city和province节点是否在图谱中
cql = "match (n:province) return (n.name);"
province_names = [_["(n.name)"] for _ in graph.run(cql).data()]
cql = "match (n:city) return (n.name);"
city_names = [_["(n.name)"] for _ in graph.run(cql).data()]
# 创建节点
s_time = time.time()
create_node_cnt = 0
create_nodes = []
for node in nodes:
label = node["label"]
name = node["name"]
if label == "city" and name not in city_names:
attrs = {k: v for k, v in node.items() if k != "label"}
create_nodes.append(Node(label, **attrs))
create_node_cnt += 1
elif label == "province" and name not in province_names:
attrs = {k: v for k, v in node.items() if k != "label"}
create_nodes.append(Node(label, **attrs))
create_node_cnt += 1
# 批量创建节点
batch_size = 50
if create_nodes:
for i in range(len(create_nodes)//50 + 1):
subgraph = Subgraph(create_nodes[i*batch_size: (i+1)*batch_size])
graph.create(subgraph)
print(f"create {(i+1)*batch_size} nodes")
# 创建关系
cql = "match (n:province) return (n);"
province_nodes = [_["n"] for _ in graph.run(cql).data()]
cql = "match (n:city) return (n);"
city_nodes = [_["n"] for _ in graph.run(cql).data()]
city_dict = {_["name"]: _ for _ in city_nodes}
province_dict = {_["name"]: _ for _ in province_nodes}
create_rel_cnt = 0
create_relations = []
rel_matcher = RelationshipMatcher(graph)
for relation in relations:
s_node, s_label = relation["subject"], relation["subject_type"]
e_node, e_label = relation["object"], relation["object_type"]
rel = relation["predicate"]
start_node, end_node = None, None
if s_label == "city":
start_node = city_dict.get(s_node, None)
if e_label == "city":
end_node = city_dict.get(e_node, None)
elif e_label == "province":
end_node = province_dict.get(e_node, None)
if start_node is not None and end_node is not None:
r_type = rel_matcher.match([start_node, end_node], r_type=rel).first()
if r_type is None:
create_relations.append(Relationship(start_node, rel, end_node))
create_rel_cnt += 1
# 批量创建关系
batch_size = 50
if create_relations:
for i in range(len(create_relations)//50 + 1):
subgraph = Subgraph(relationships=create_relations[i*batch_size: (i+1)*batch_size])
graph.create(subgraph)
print(f"create {(i+1)*batch_size} relations")
# 输出信息
e_time = time.time()
print(f"create {create_node_cnt} nodes, create {create_rel_cnt} relations.")
print(f"cost time: {round((e_time-s_time)*1000, 4)}ms")
初次运行该脚本时,创建整个图谱(创建2834个节点,18807条关系)需要95.1秒。
再次运行该脚本时,创建整个图谱(创建0个节点,0条关系)需要522.5秒。
注意,上述脚本的耗时需要体现在查询所有city和province节点,并返回这些节点的查询过程中,构建节点、关系是很快的,但为了避免重复插入节点和关系,这一步查询所有city和province节点是很有必要的。当然,如果节点数量过大时,应考虑其他方案,因为查询所有节点也是很耗时并且消耗内存的。
总结
本文主要介绍了如何在Neo4j中批量创建节点、关系,从而提升图谱构建效率。
2021年7月15日于上海浦东,此日上海酷暑逼人~